Riemannian data preprocessing in machine learning to focus on QCD color structure
arxiv(2023)
摘要
Identifying the quantum chromodynamics (QCD) color structure of processes provides additional information to enhance the reach for new physics searches at the large Hadron collider (LHC). Analyses of QCD color structure in the decay process of a boosted particle have been spotted as information becomes well localized in the limited phase space. While these kinds of a boosted jet analyses provide an efficient way to identify the color structure, the constrained phase space reduces the number of available data, resulting in a low significance. In this letter, we provide a simple but a novel data preprocessing method using a Riemann sphere to utilize a full phase space by decorrelating QCD structure from kinematics. We can achieve statistical stability by enlarging the size of testable data set with focusing on QCD structure effectively. We demonstrate the power of our method with the finite statistics of the LHC Run 2. Our method is complementary to conventional boosted jet analyses in utilizing QCD information over a wide range of a phase space.
更多查看译文
关键词
Collider physics, Higgs particle, Machine learning, The LHC experiment
AI 理解论文
溯源树
样例
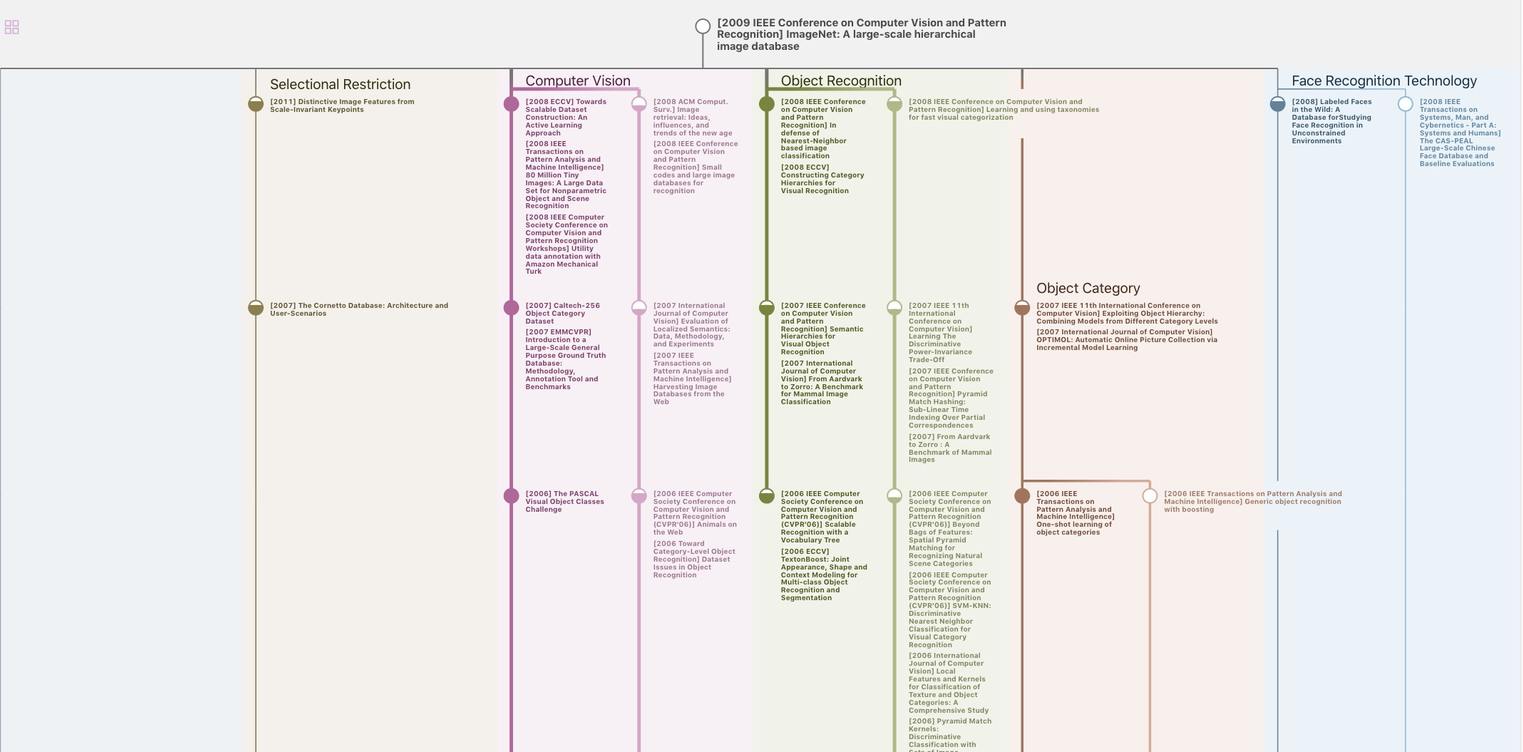
生成溯源树,研究论文发展脉络
Chat Paper
正在生成论文摘要