SVASeg: Sparse Voxel-Based Attention for 3D LiDAR Point Cloud Semantic Segmentation
REMOTE SENSING(2022)
摘要
3D LiDAR has become an indispensable sensor in autonomous driving vehicles. In LiDAR-based 3D point cloud semantic segmentation, most voxel-based 3D segmentors cannot efficiently capture large amounts of context information, resulting in limited receptive fields and limiting their performance. To address this problem, a sparse voxel-based attention network is introduced for 3D LiDAR point cloud semantic segmentation, termed SVASeg, which captures large amounts of context information between voxels through sparse voxel-based multi-head attention (SMHA). The traditional multi-head attention cannot directly be applied to the non-empty sparse voxels. To this end, a hash table is built according to the incrementation of voxel coordinates to lookup the non-empty neighboring voxels of each sparse voxel. Then, the sparse voxels are grouped into different groups, and each group corresponds to a local region. Afterwards, position embedding, multi-head attention and feature fusion are performed for each group to capture and aggregate the context information. Based on the SMHA module, the SVASeg can directly operate on the non-empty voxels, maintaining a comparable computational overhead to the convolutional method. Extensive experimental results on the SemanticKITTI and nuScenes datasets show the superiority of SVASeg.
更多查看译文
关键词
sparse voxel-based attention,LiDAR point cloud,semantic segmentation,SVASeg
AI 理解论文
溯源树
样例
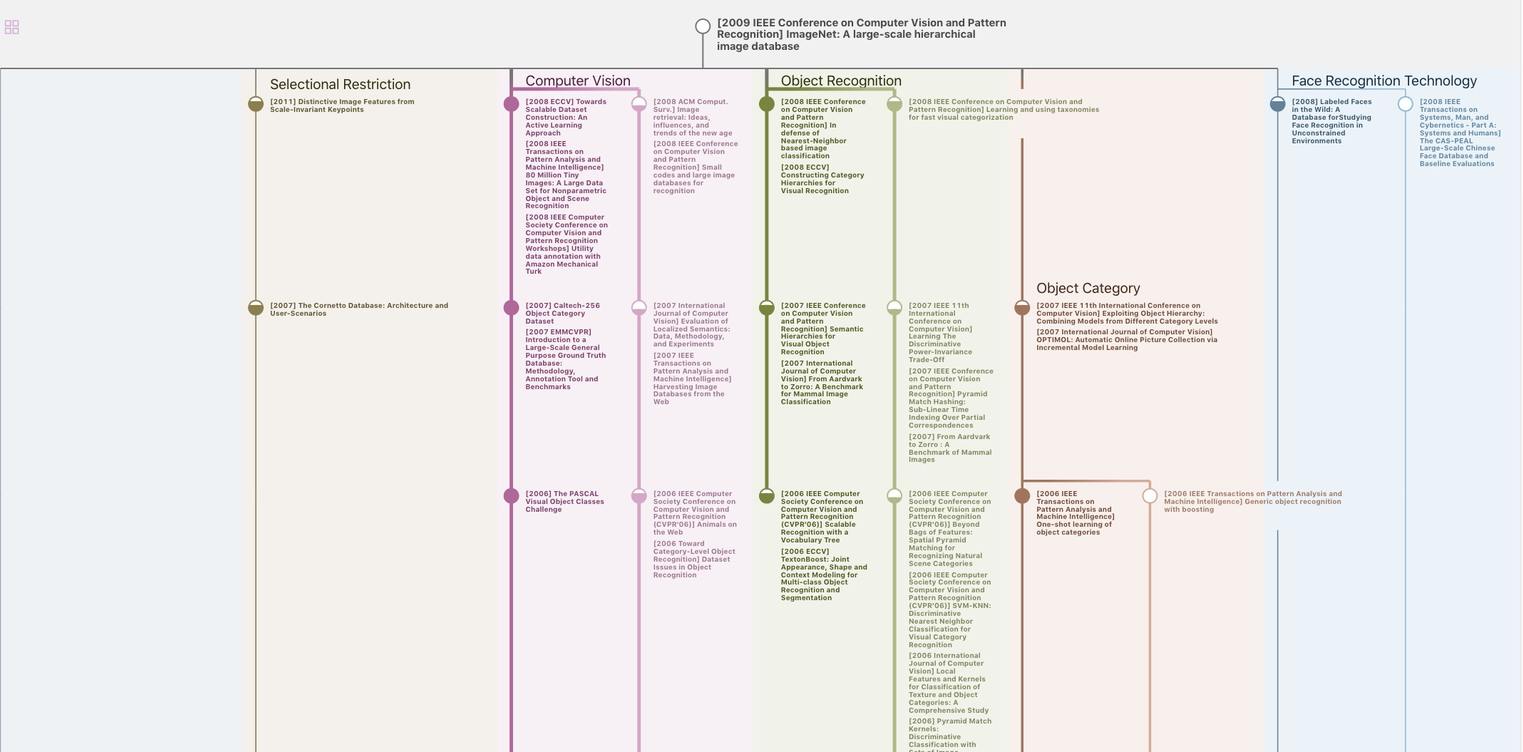
生成溯源树,研究论文发展脉络
Chat Paper
正在生成论文摘要