Deep Learning to Near-Surface Humidity Retrieval from Multi-Sensor Remote Sensing Data over the China Seas
REMOTE SENSING(2022)
摘要
Near-surface humidity (Q(a)) is a key parameter that modulates oceanic evaporation and influences the global water cycle. Remote sensing observations act as feasible sources for long-term and large-scale Q(a) monitoring. However, existing satellite Q(a) retrieval models are subject to apparent uncertainties due to model errors and insufficient training data. Based on in situ observations collected over the China Seas over the last two decades, a deep learning approach named Ensemble Mean of Target deep neural networks (EMTnet) is proposed to improve the satellite Q(a) retrieval over the China Seas for the first time. The EMTnet model outperforms five representative existing models by nearly eliminating the mean bias and significantly reducing the root-mean-square error in satellite Q(a) retrieval. According to its target deep neural network selection process, the EMTnet model can obtain more objective learning results when the observational data are divergent. The EMTnet model was subsequently applied to produce 30-year monthly gridded Q(a) data over the China Seas. It indicates that the climbing rate of Q(a) over the China Seas under the background of global warming is probably underestimated by current products.
更多查看译文
关键词
near-surface humidity,remote sensing,deep learning,China Seas
AI 理解论文
溯源树
样例
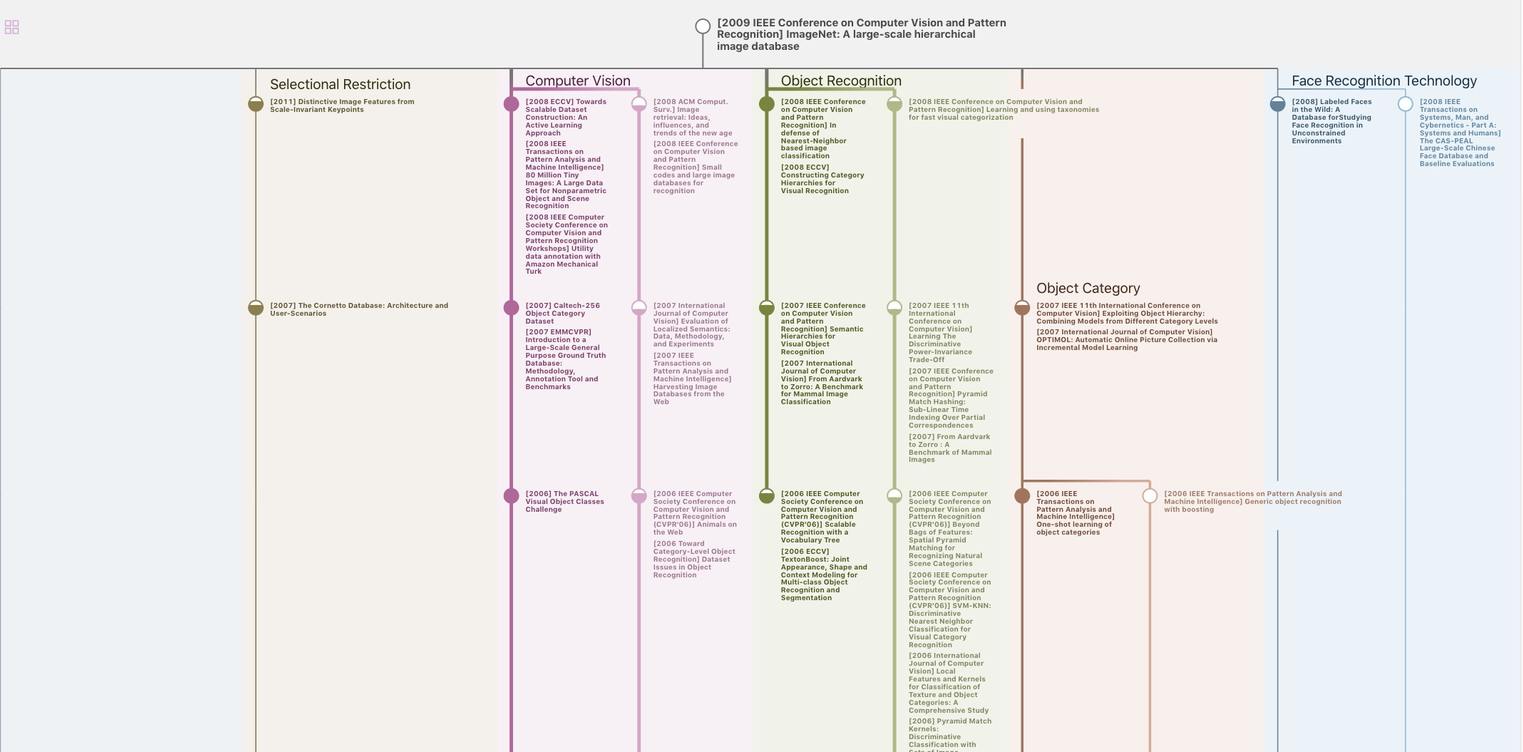
生成溯源树,研究论文发展脉络
Chat Paper
正在生成论文摘要