Towards explainable model extraction attacks
INTERNATIONAL JOURNAL OF INTELLIGENT SYSTEMS(2022)
摘要
One key factor able to boost the applications of artificial intelligence (AI) in security-sensitive domains is to leverage them responsibly, which is engaged in providing explanations for AI. To date, a plethora of explainable artificial intelligence (XAI) has been proposed to help users interpret model decisions. However, given its data-driven nature, the explanation itself is potentially susceptible to a high risk of exposing privacy. In this paper, we first show that the existing XAI is vulnerable to model extraction attacks and then present an XAI-aware dual-task model extraction attack (DTMEA). DTMEA can attack a target model with explanation services, that is, it can extract both the classification and explanation tasks of the target model. More specifically, the substitution model extracted by DTMEA is a multitask learning architecture, consisting of a sharing layer and two task-specific layers for classification and explanation. To reveal which explanation technologies are more vulnerable to expose privacy information, we conduct an empirical evaluation of four major explanation types in the benchmark data set. Experimental results show that the attack accuracy of DTMEA outperforms the predicted-only method with up to 1.25%, 1.53%, 9.25%, and 7.45% in MNIST, Fashion-MNIST, CIFAR-10, and CIFAR-100, respectively. By exposing the potential threats on explanation technologies, our research offers the insights to develop effective tools that are able to trade off security-sensitive relationships.
更多查看译文
关键词
black box,explainable artificial intelligence,label-only,model extraction attack
AI 理解论文
溯源树
样例
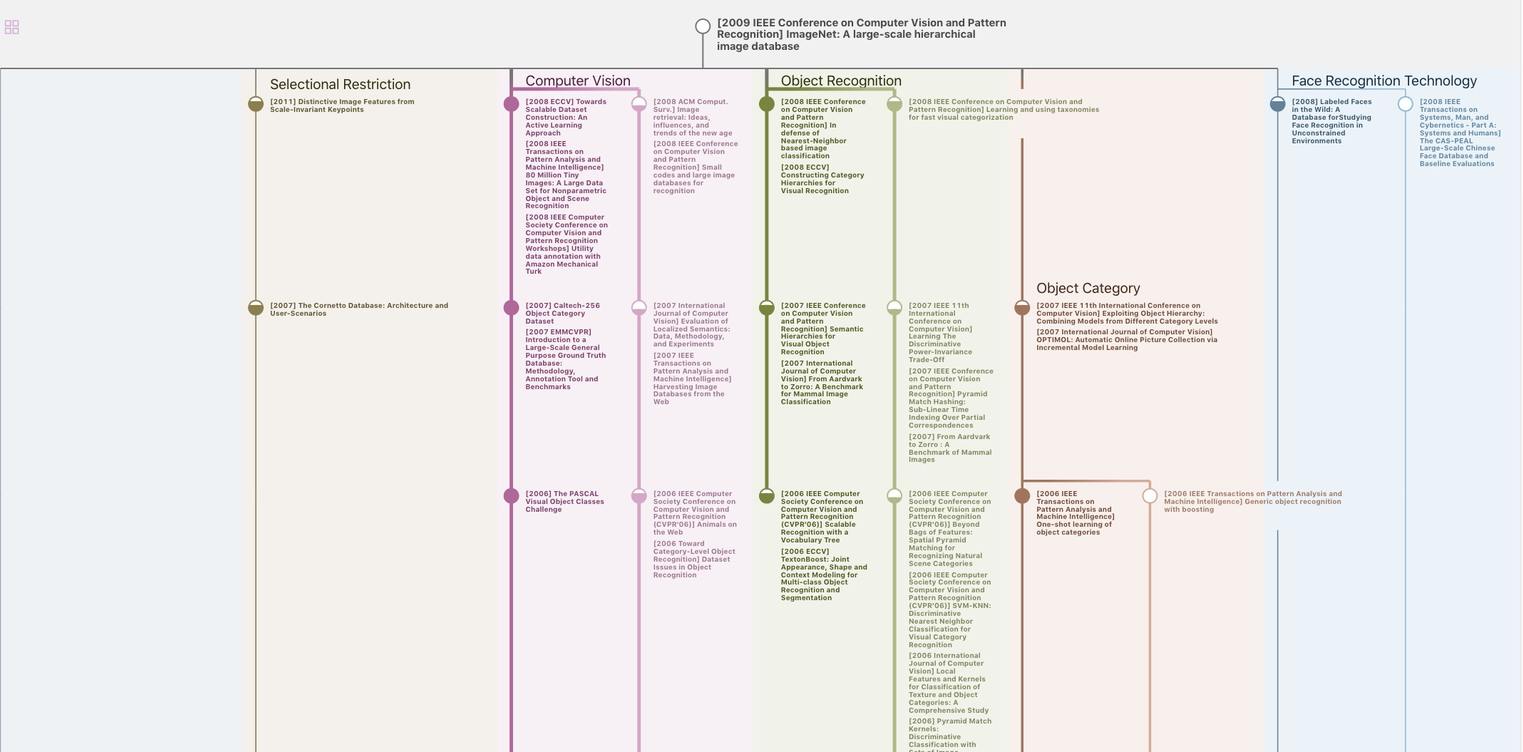
生成溯源树,研究论文发展脉络
Chat Paper
正在生成论文摘要