Multimodal multitask learning for predicting MCI to AD conversion using stacked polynomial attention network and adaptive exponential decay
SSRN Electronic Journal(2023)
摘要
Early identification and treatment of moderate cognitive impairment (MCI) can halt or postpone Alzheimer’s disease (AD) and preserve brain function. For prompt diagnosis and AD reversal, precise prediction in the early and late phases of MCI is essential. This research investigates multimodal framework-based multitask learning in the following situations: (1) Differentiating early mild cognitive impairment (eMCI) from late MCI and (2) predicting when an MCI patient would acquire AD. Clinical data and two radiomics features on three brain areas deduced from magnetic resonance imaging were investigated (MRI). We proposed an attention-based module, Stack Polynomial Attention Network (SPAN), to firmly encode clinical and radiomics data input characteristics for successful representation from a small dataset. To improve multimodal data learning, we computed a potent factor using adaptive exponential decay (AED). We used experiments from the Alzheimer’s Disease Neuroimaging Initiative (ADNI) cohort study, which included 249 eMCI and 427 lMCI participants at baseline visits. The proposed multimodal strategy yielded the best c-index score in time prediction of MCI to AD conversion (0.85) and the best accuracy in MCI-stage categorization ( 83.19% ). Moreover, our performance was equivalent to that of contemporary research.
更多查看译文
关键词
ad conversion,polynomial attention network,mci
AI 理解论文
溯源树
样例
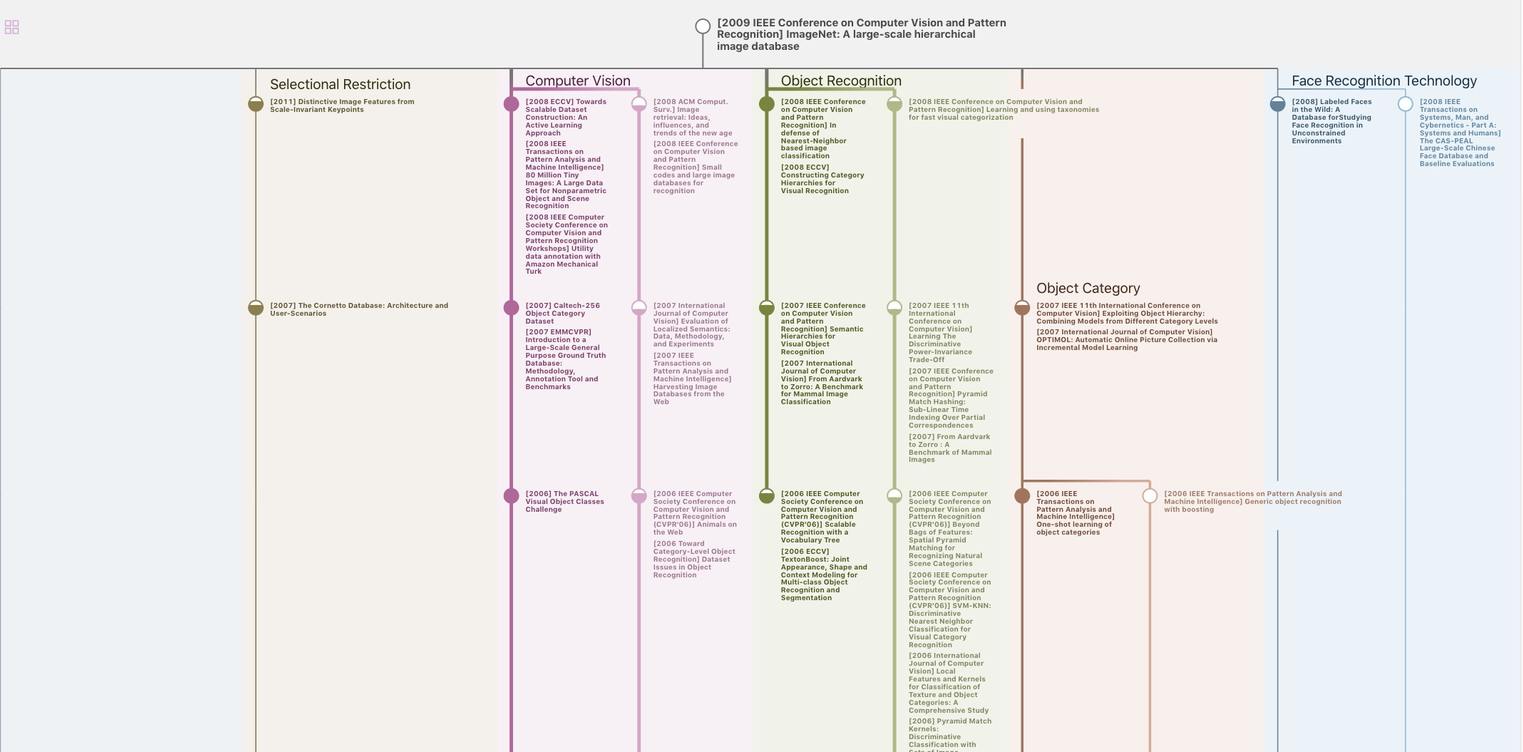
生成溯源树,研究论文发展脉络
Chat Paper
正在生成论文摘要