Learnable Depth-Sensitive Attention for Deep RGB-D Saliency Detection with Multi-modal Fusion Architecture Search
International Journal of Computer Vision(2022)
摘要
RGB-D salient object detection (SOD) is usually formulated as a problem of classification or regression over two modalities, i.e. , RGB and depth. Hence, effective RGB-D feature modeling and multi-modal feature fusion both play a vital role in RGB-D SOD. In this paper, we propose a depth-sensitive RGB feature modeling scheme using the depth-wise geometric prior of salient objects. In principle, the feature modeling scheme is carried out in a Depth-Sensitive Attention Module (DSAM), which leads to the RGB feature enhancement as well as the background distraction reduction by capturing the depth geometry prior. Furthermore, we extend and enhance the original DSAM to DSAMv2 by proposing a novel Depth Attention Generation Module (DAGM) to generate learnable depth attention maps for more robust depth-sensitive RGB feature extraction. Moreover, to perform effective multi-modal feature fusion, we further present an automatic neural architecture search approach for RGB-D SOD, which does well in finding out a feasible architecture from our specially designed multi-modal multi-scale search space. Extensive experiments on nine standard benchmarks have demonstrated the effectiveness of the proposed approach against the state-of-the-art. We name the enhanced learnable D epth- S ensitive A ttention and A utomatic multi-modal F usion framework DSA ^2 Fv2.
更多查看译文
关键词
RGB-D salient object detection,Depth-sensitive RGB feature,Multi-modal feature fusion
AI 理解论文
溯源树
样例
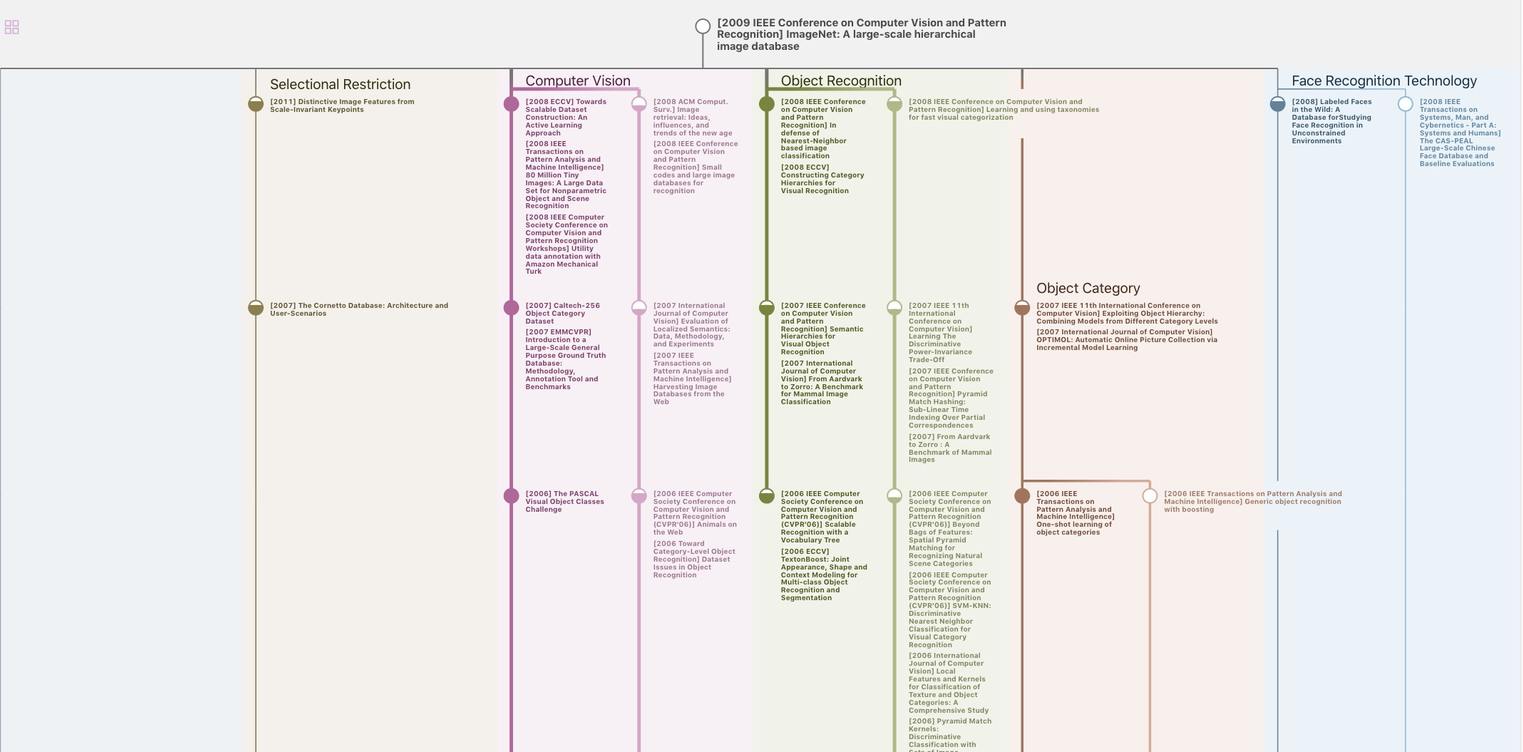
生成溯源树,研究论文发展脉络
Chat Paper
正在生成论文摘要