SCL-RAI: Span-based Contrastive Learning with Retrieval Augmented Inference for Unlabeled Entity Problem in NER.
arXiv (Cornell University)(2022)
摘要
Unlabeled Entity Problem (UEP) in Named Entity Recognition (NER) datasets seriously hinders the improvement of NER performance. This paper proposes SCL-RAI to cope with this problem. Firstly, we decrease the distance of span representations with the same label while increasing it for different ones via span-based contrastive learning, which relieves the ambiguity among entities and improves the robustness of the model over unlabeled entities. Then we propose retrieval augmented inference to mitigate the decision boundary shifting problem. Our method significantly outperforms the previous SOTA method by 4.21% and 8.64% F1-score on two real-world datasets.
更多查看译文
关键词
contrastive learning,unlabeled entity problem,retrieval augmented
AI 理解论文
溯源树
样例
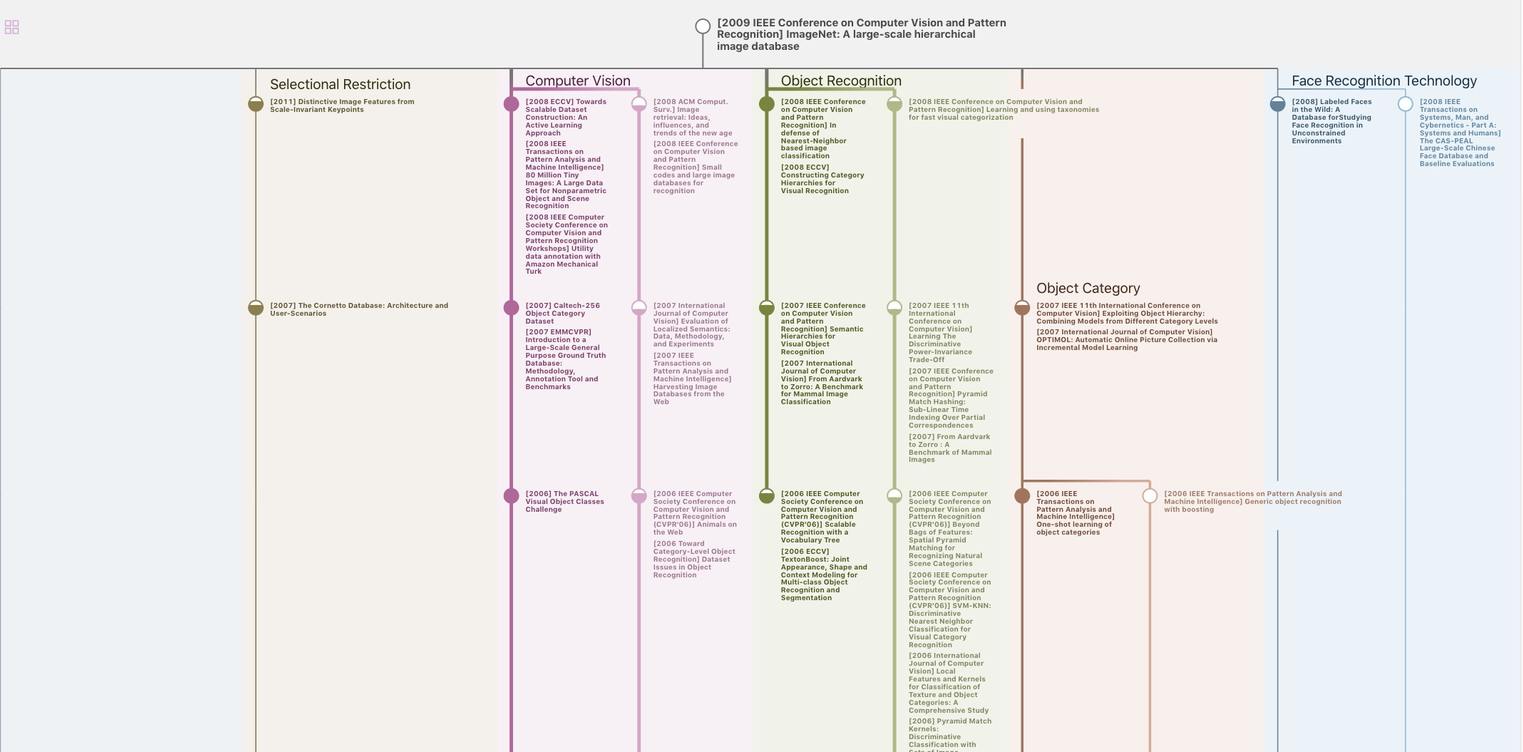
生成溯源树,研究论文发展脉络
Chat Paper
正在生成论文摘要