Learning to Deceive in Multi-Agent Hidden Role Games
arxiv(2022)
摘要
Deception is prevalent in human social settings. However, studies into the effect of deception on reinforcement learning algorithms have been limited to simplistic settings, restricting their applicability to complex real-world problems. This paper addresses this by introducing a new mixed competitive-cooperative multi-agent reinforcement learning (MARL) environment inspired by popular role-based deception games such as Werewolf, Avalon, and Among Us. The environment's unique challenge lies in the necessity to cooperate with other agents despite not knowing if they are friend or foe. Furthermore, we introduce a model of deception, which we call Bayesian belief manipulation (BBM) and demonstrate its effectiveness at deceiving other agents in this environment while also increasing the deceiving agent's performance.
更多查看译文
关键词
Machine learning, Deep reinforcement learning, Deception, Intrinsic motivation, Bayesian belief
AI 理解论文
溯源树
样例
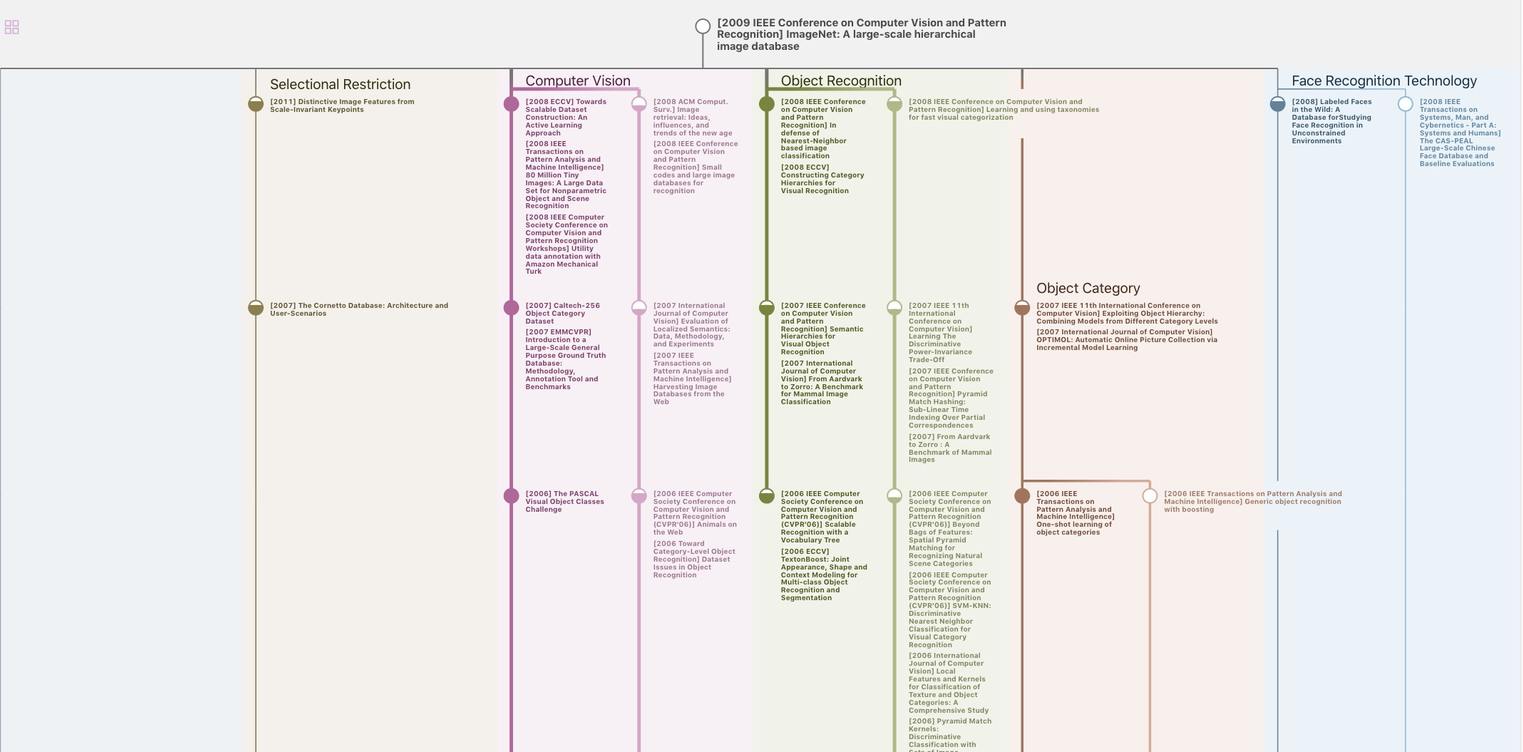
生成溯源树,研究论文发展脉络
Chat Paper
正在生成论文摘要