Evaluation of Model-Based PM 2.5 Estimates for Exposure Assessment during Wildfire Smoke Episodes in the Western U.S.
Environmental science & technology(2023)
摘要
Investigating the health impacts of wildfire smoke requires data on people's exposure to fine particulate matter (PM) across space and time. In recent years, it has become common to use machine learning models to fill gaps in monitoring data. However, it remains unclear how well these models are able to capture spikes in PM during and across wildfire events. Here, we evaluate the accuracy of two sets of high-coverage and high-resolution machine learning-derived PM data sets created by Di et al. and Reid et al. In general, the Reid estimates are more accurate than the Di estimates when compared to independent validation data from mobile smoke monitors deployed by the US Forest Service. However, both models tend to severely under-predict PM on high-pollution days. Our findings complement other recent studies calling for increased air pollution monitoring in the western US and support the inclusion of wildfire-specific monitoring observations and predictor variables in model-based estimates of PM. Lastly, we call for more rigorous error quantification of machine-learning derived exposure data sets, with special attention to extreme events.
更多查看译文
关键词
Air Pollution,Data Science,Environmental Health,Exposure Assessment,Validation,Wildfires
AI 理解论文
溯源树
样例
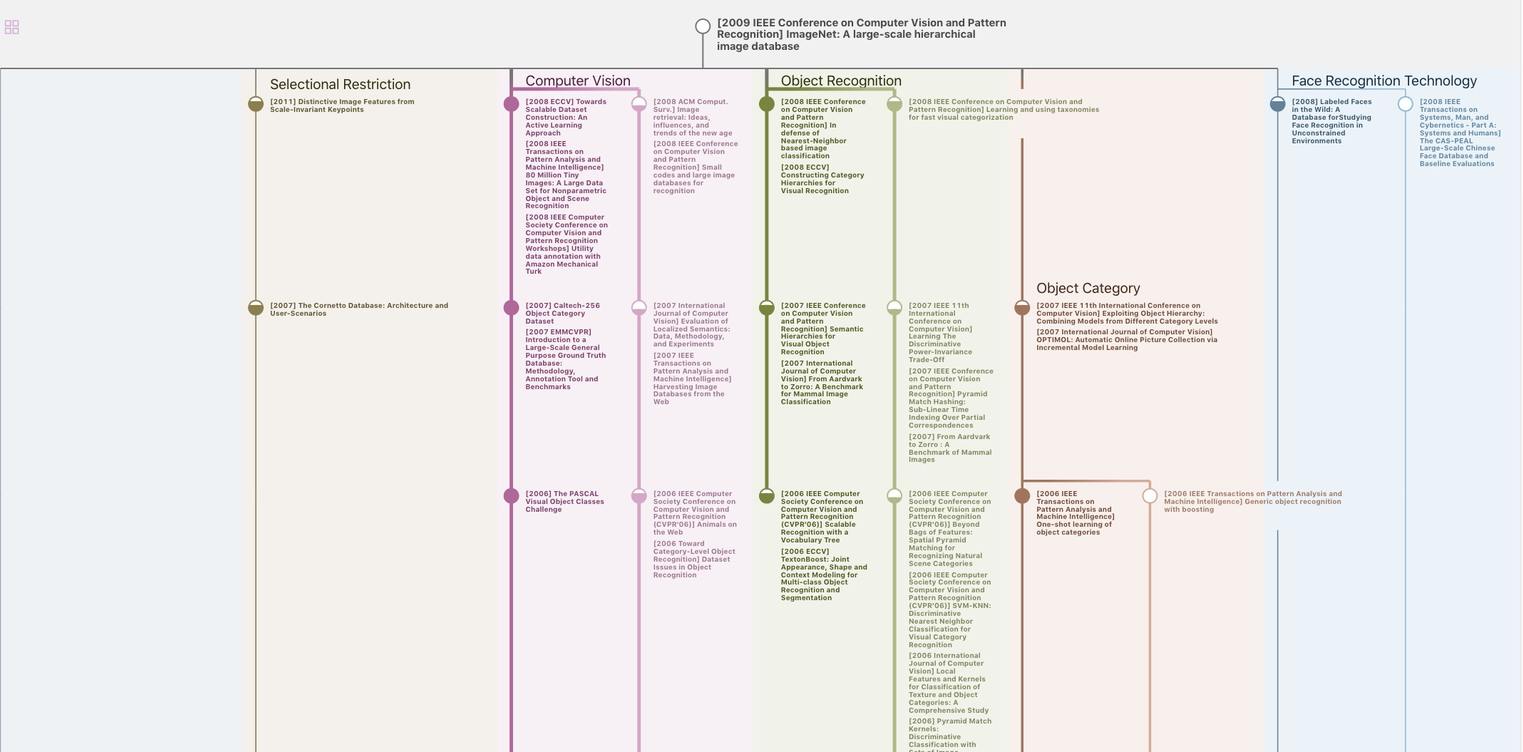
生成溯源树,研究论文发展脉络
Chat Paper
正在生成论文摘要