Semantic Segmentation In Learned Compressed Domain
2022 Picture Coding Symposium (PCS)(2022)
摘要
Most machine vision tasks (e.g., semantic segmentation) are based on images encoded and decoded by image compression algorithms (e.g., JPEG). However, these decoded images in the pixel domain introduce distortion, and they are optimized for human perception, making the performance of machine vision tasks suboptimal. In this paper, we propose a method based on the compressed domain to improve segmentation tasks. i) A dynamic and a static channel selection method are proposed to reduce the redundancy of compressed representations that are obtained by encoding. ii) Two different transform modules are explored and analyzed to help the compressed representation be transformed as the features in the segmentation network. The experimental results show that we can save up to 15.8% bitrates compared with a state-of-the-art compressed domain-based work while saving up to about 83.6% bitrates and 44.8% inference time compared with the pixel domain-based method.
更多查看译文
关键词
Image compression,Compressed domain,Channel selection,Deep learning
AI 理解论文
溯源树
样例
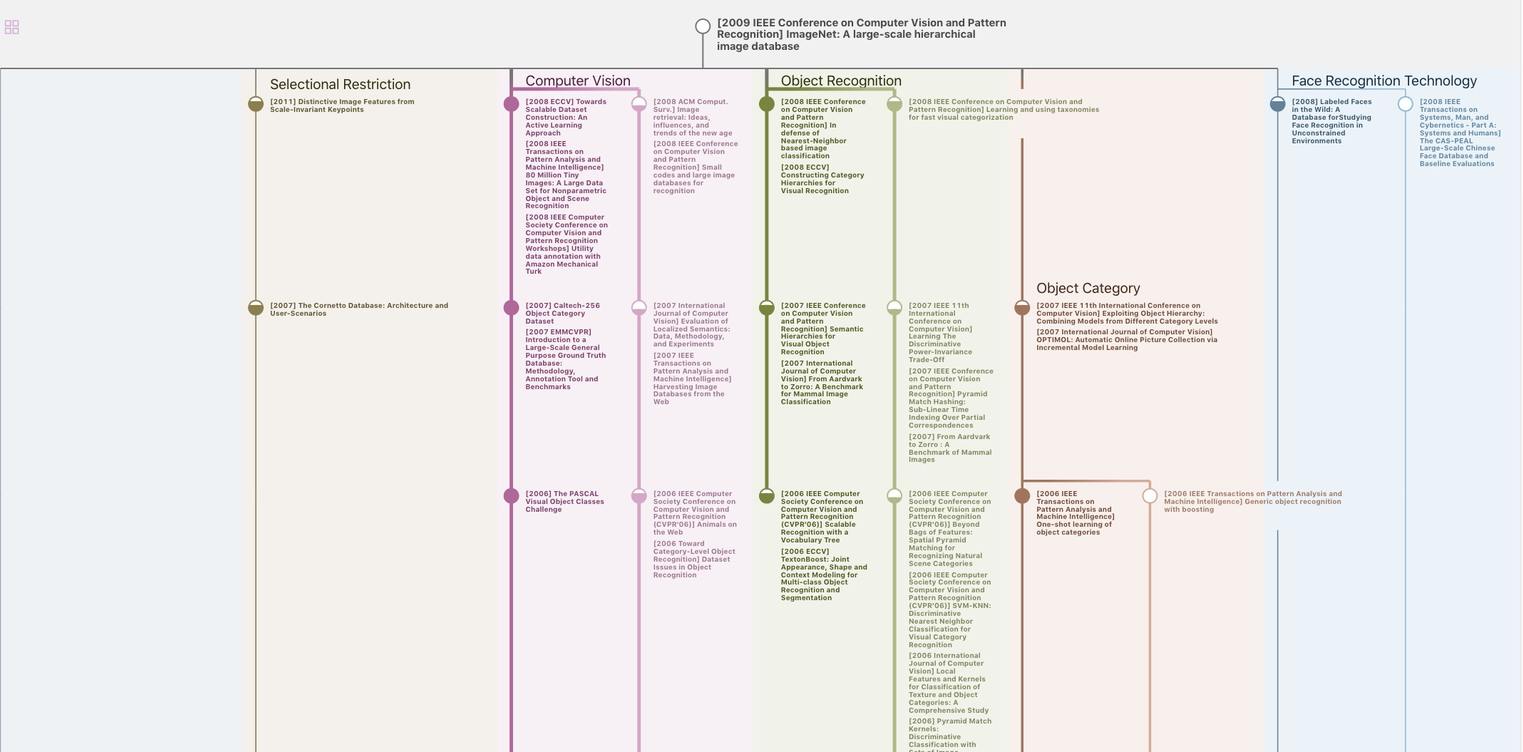
生成溯源树,研究论文发展脉络
Chat Paper
正在生成论文摘要