Continual Learning for Steganalysis
arxiv(2022)
摘要
To detect the existing steganographic algorithms, recent steganalysis methods usually train a Convolutional Neural Network (CNN) model on the dataset consisting of corresponding paired cover/stego-images. However, it is inefficient and impractical for those steganalysis tools to completely retrain the CNN model to make it effective against both the existing steganographic algorithms and a new emerging steganographic algorithm. Thus, existing steganalysis models usually lack dynamic extensibility for new steganographic algorithms, which limits their application in real-world scenarios. To address this issue, we propose an accurate parameter importance estimation (APIE) based-continual learning scheme for steganalysis. In this scheme, when a steganalysis model is trained on the new image dataset generated by the new steganographic algorithm, its network parameters are effectively and efficiently updated with sufficient consideration of their importance evaluated in the previous training process. This approach can guide the steganalysis model to learn the patterns of the new steganographic algorithm without significantly degrading the detectability against the previous steganographic algorithms. Experimental results demonstrate the proposed scheme has promising extensibility for new emerging steganographic algorithms.
更多查看译文
关键词
steganalysis,continual learning
AI 理解论文
溯源树
样例
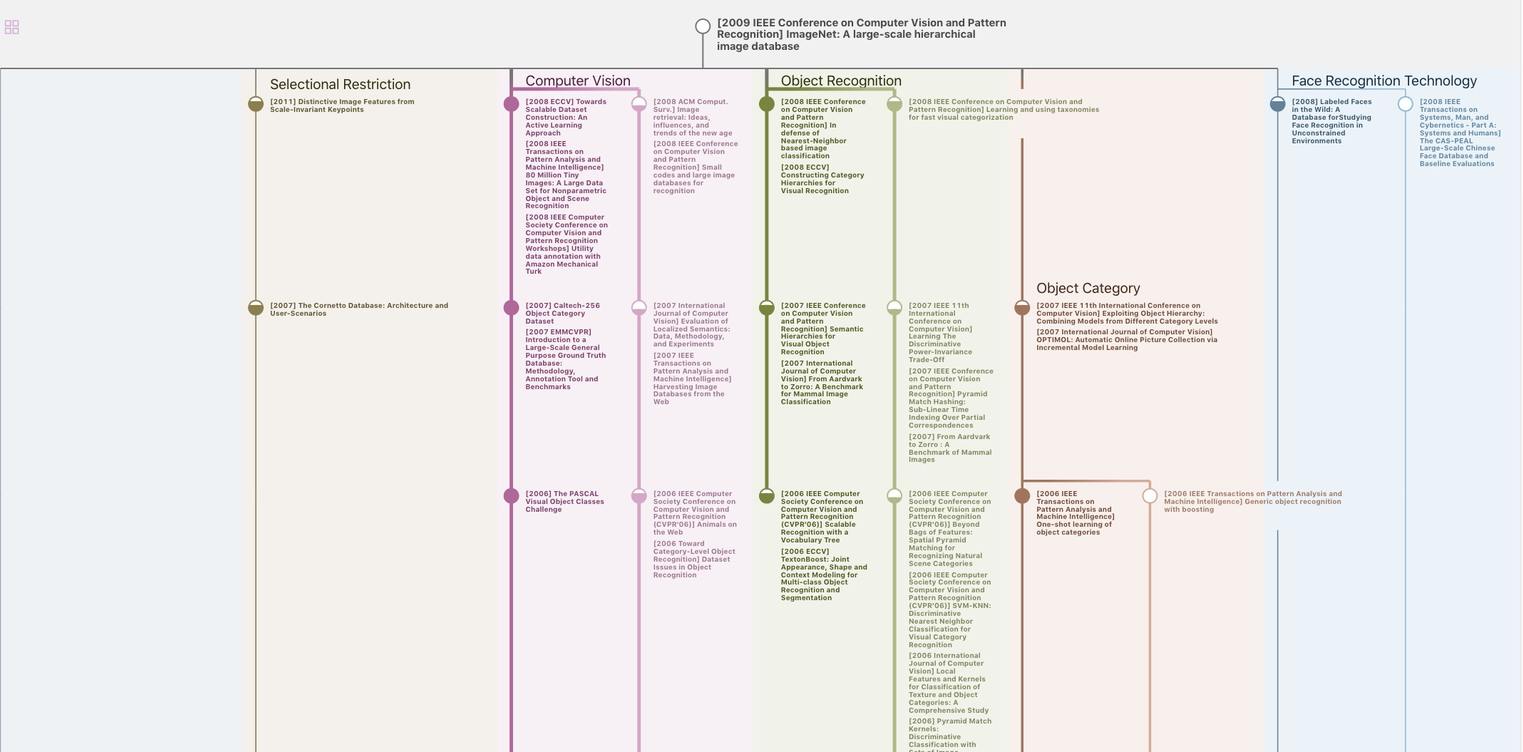
生成溯源树,研究论文发展脉络
Chat Paper
正在生成论文摘要