Quasi-supervised learning for super-resolution PET
COMPUTERIZED MEDICAL IMAGING AND GRAPHICS(2024)
摘要
Low resolution of positron emission tomography (PET) limits its diagnostic performance. Deep learning has been successfully applied to achieve super -resolution PET. However, commonly used supervised learning methods in this context require many pairs of low- and high -resolution (LR and HR) PET images. Although unsupervised learning utilizes unpaired images, the results are not as good as that obtained with supervised deep learning. In this paper, we propose a quasi -supervised learning method, which is a new type of weaklysupervised learning methods, to recover HR PET images from LR counterparts by leveraging similarity between unpaired LR and HR image patches. Specifically, LR image patches are taken from a patient as inputs, while the most similar HR patches from other patients are found as labels. The similarity between the matched HR and LR patches serves as a prior for network construction. Our proposed method can be implemented by designing a new network or modifying an existing network. As an example in this study, we have modified the cycle -consistent generative adversarial network (CycleGAN) for super -resolution PET. Our numerical and experimental results qualitatively and quantitatively show the merits of our method relative to the state-of-the-art methods. The code is publicly available at https://github.com/PigYang-ops/CycleGAN-QSDL.
更多查看译文
关键词
Weakly-supervised learning,Positron emission tomography (PET),Super-resolution,Unpaired data
AI 理解论文
溯源树
样例
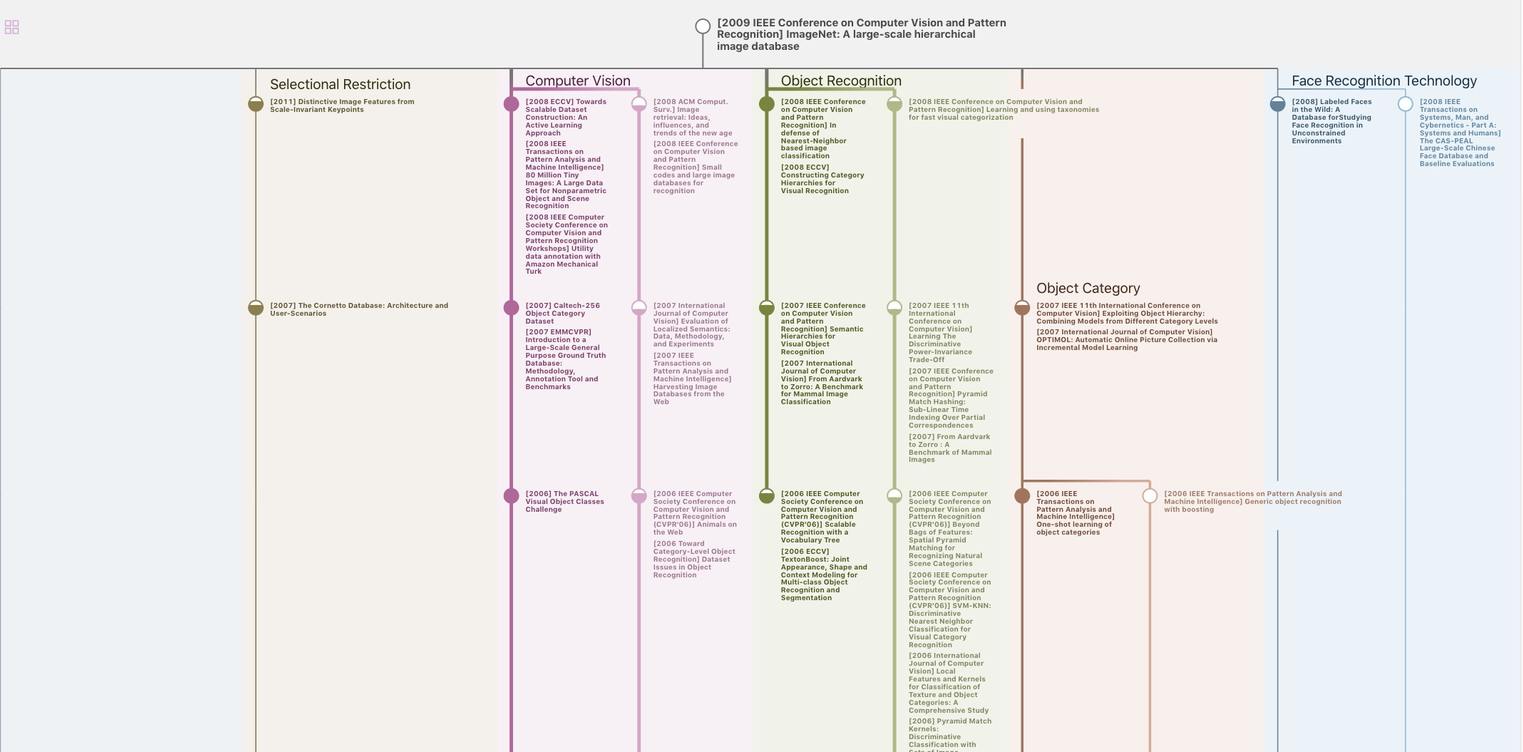
生成溯源树,研究论文发展脉络
Chat Paper
正在生成论文摘要