Impact of Sensor and Actuator Clock Offsets on Reinforcement Learning.
ACC(2022)
摘要
In this work, we investigate the effect of sensor-actuator clock offsets on reinforcement learning (RL) enabled cyber-physical systems. In particular, we consider an off-policy RL algorithm that receives data both from the system's sensors and actuators, and uses them to approximate a desired optimal control policy. Nevertheless, owing to timing mismatches, the control-state data obtained from these system components are inconsistent, hence creating the question of how robust RL will be. After an extensive analysis, we show that RL does retain its robustness, in an epsilon-delta sense; given that the sensor-actuator clock offsets are not arbitrarily large, and that the behavioral control input satisfies a Lipschitz continuity condition, RL converges epsilon-close to the desired optimal control policy. Simulations are carried out on a two-link manipulator, which clarify and verify theoretical findings.
更多查看译文
关键词
control-state data,robust RL,sensor-actuator clock offsets,optimal control policy,reinforcement learning enabled cyber-physical systems,off-policy RL algorithm,epsilon-delta sense,Lipschitz continuity condition,RL converge epsilon-close,two-link manipulator,cyber-physical system
AI 理解论文
溯源树
样例
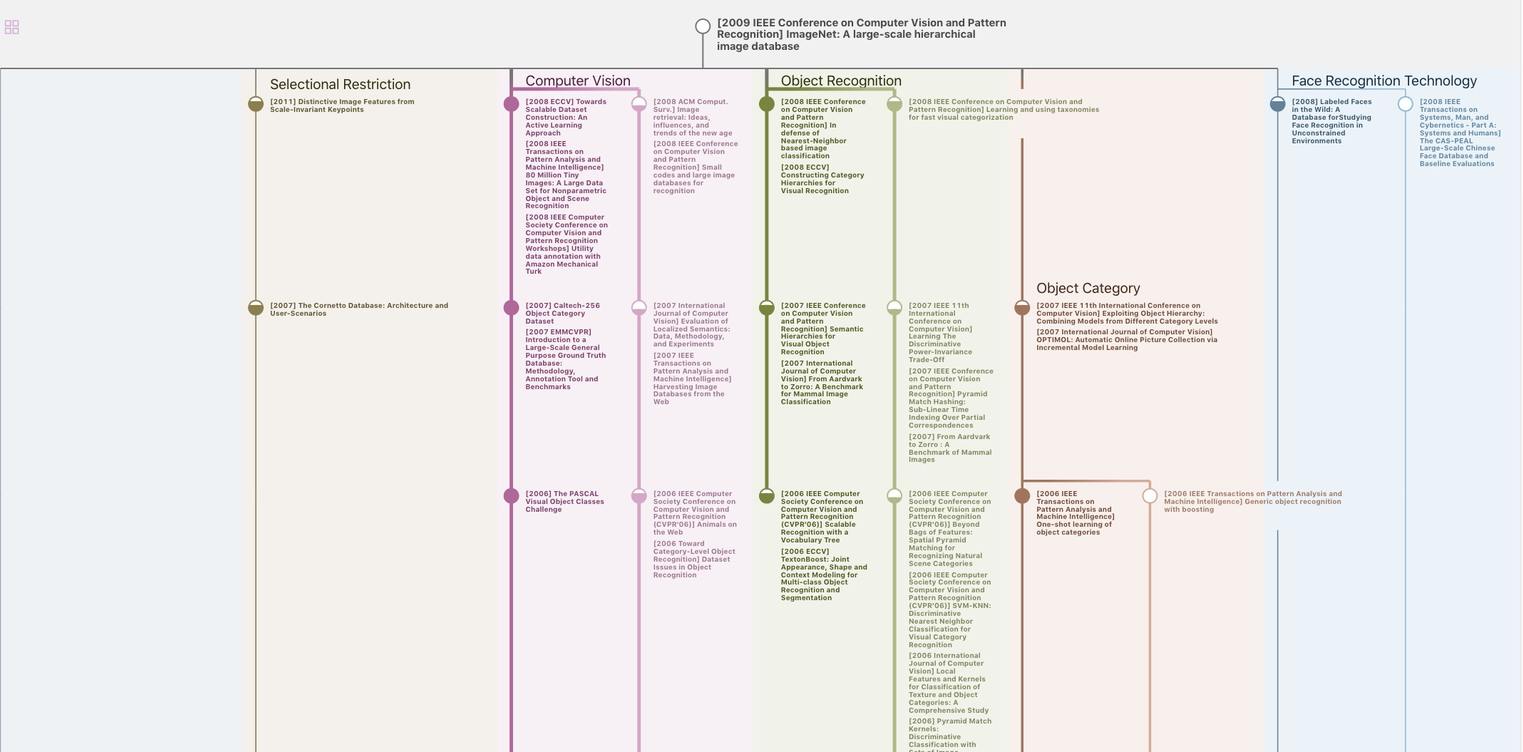
生成溯源树,研究论文发展脉络
Chat Paper
正在生成论文摘要