Machine learning Post-Minkowskian integrals
Journal of High Energy Physics(2023)
摘要
bstract We study a neural network framework for the numerical evaluation of Feynman loop integrals that are fundamental building blocks for perturbative computations of physical observables in gauge and gravity theories. We show that such a machine learning approach improves the convergence of the Monte Carlo algorithm for high-precision evaluation of multi-dimensional integrals compared to traditional algorithms. In particular, we use a neural network to improve the importance sampling. For a set of representative integrals appearing in the computation of the conservative dynamics for a compact binary system in General Relativity, we perform a quantitative comparison between the Monte Carlo integrators VEGAS and i-flow, an integrator based on neural network sampling.
更多查看译文
关键词
Scattering Amplitudes,Classical Theories of Gravity,Effective Field Theories
AI 理解论文
溯源树
样例
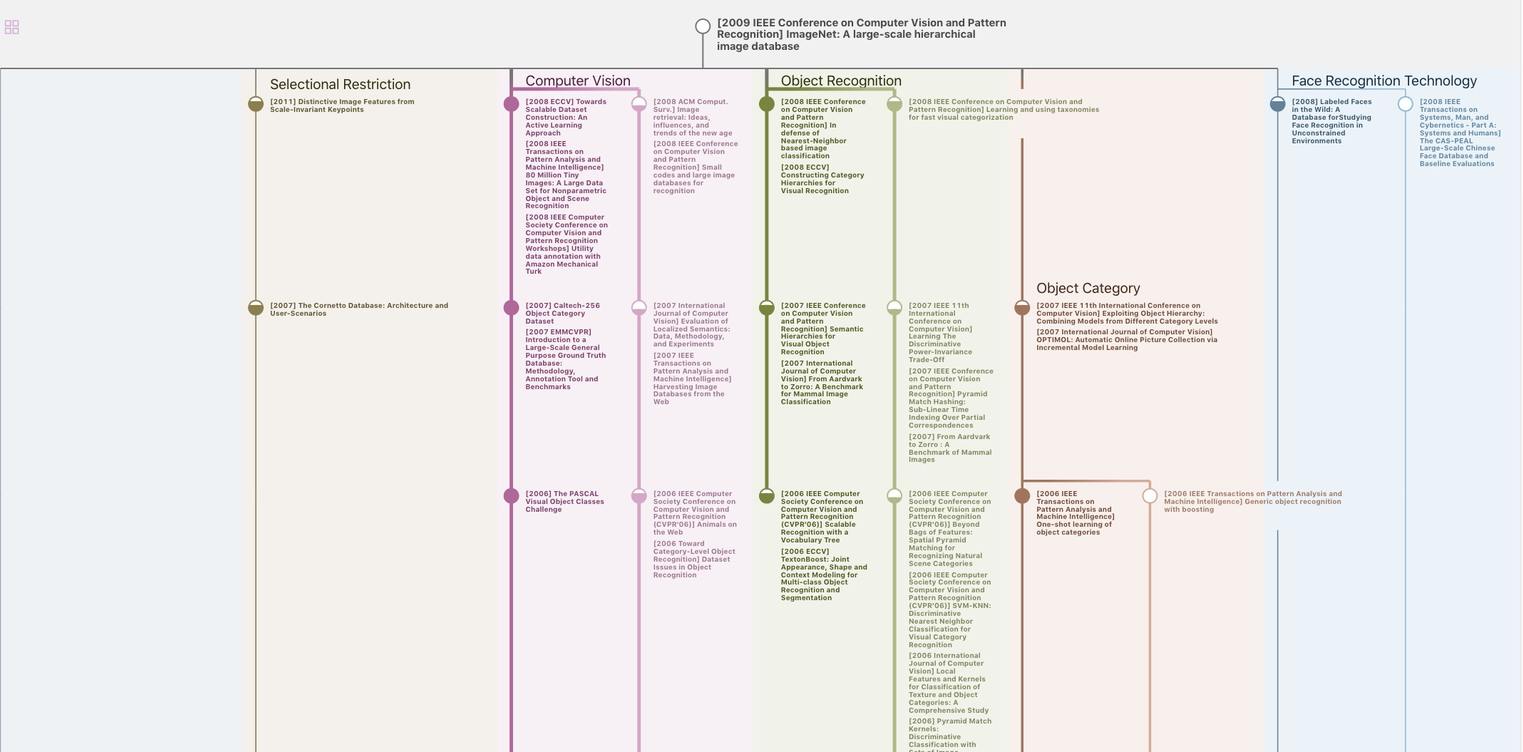
生成溯源树,研究论文发展脉络
Chat Paper
正在生成论文摘要