Optimizing an existing prediction model for quality of life one-year post-intensive care unit: An exploratory analysis
ACTA ANAESTHESIOLOGICA SCANDINAVICA(2022)
摘要
Background This study aimed to improve the PREPARE model, an existing linear regression prediction model for long-term quality of life (QoL) of intensive care unit (ICU) survivors by incorporating additional ICU data from patients' electronic health record (EHR) and bedside monitors. Methods The 1308 adult ICU patients, aged >= 16, admitted between July 2016 and January 2019 were included. Several regression-based machine learning models were fitted on a combination of patient-reported data and expert-selected EHR variables and bedside monitor data to predict change in QoL 1 year after ICU admission. Predictive performance was compared to a five-feature linear regression prediction model using only 24-hour data (R-2 = 0.54, mean square error (MSE) = 0.031, mean absolute error (MAE) = 0.128). Results The 67.9% of the included ICU survivors was male and the median age was 65.0 [IQR: 57.0-71.0]. Median length of stay (LOS) was 1 day [IQR 1.0-2.0]. The incorporation of the additional data pertaining to the entire ICU stay did not improve the predictive performance of the original linear regression model. The best performing machine learning model used seven features (R-2 = 0.52, MSE = 0.032, MAE = 0.125). Pre-ICU QoL, the presence of a cerebro vascular accident (CVA) upon admission and the highest temperature measured during the ICU stay were the most important contributors to predictive performance. Pre-ICU QoL's contribution to predictive performance far exceeded that of the other predictors. Conclusion Pre-ICU QoL was by far the most important predictor for change in QoL 1 year after ICU admission. The incorporation of the numerous additional features pertaining to the entire ICU stay did not improve predictive performance although the patients' LOS was relatively short.
更多查看译文
关键词
critical care, machine learning, prediction modeling, quality of life, survivors
AI 理解论文
溯源树
样例
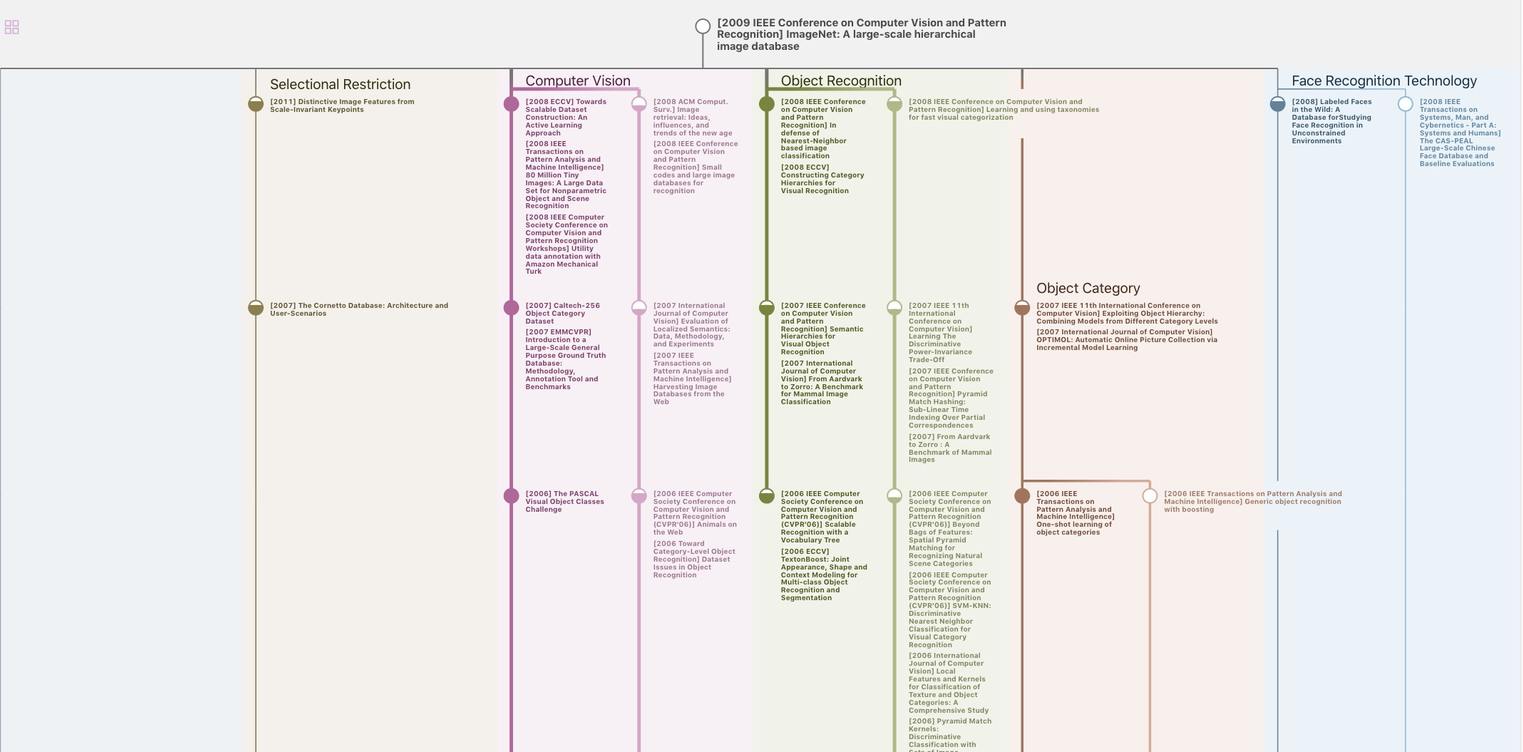
生成溯源树,研究论文发展脉络
Chat Paper
正在生成论文摘要