Predicting post-contrast information from contrast agent free cardiac MRI using machine learning: Challenges and methods
FRONTIERS IN CARDIOVASCULAR MEDICINE(2022)
摘要
ObjectivesCurrently, administering contrast agents is necessary for accurately visualizing and quantifying presence, location, and extent of myocardial infarction (MI) with cardiac magnetic resonance (CMR). In this study, our objective is to investigate and analyze pre- and post-contrast CMR images with the goal of predicting post-contrast information using pre-contrast information only. We propose methods and identify challenges. MethodsThe study population consists of 272 retrospectively selected CMR studies with diagnoses of MI (n = 108) and healthy controls (n = 164). We describe a pipeline for pre-processing this dataset for analysis. After data feature engineering, 722 cine short-axis (SAX) images and segmentation mask pairs were used for experimentation. This constitutes 506, 108, and 108 pairs for the training, validation, and testing sets, respectively. We use deep learning (DL) segmentation (UNet) and classification (ResNet50) models to discover the extent and location of the scar and classify between the ischemic cases and healthy cases (i.e., cases with no regional myocardial scar) from the pre-contrast cine SAX image frames, respectively. We then capture complex data patterns that represent subtle signal and functional changes in the cine SAX images due to MI using optical flow, rate of change of myocardial area, and radiomics data. We apply this dataset to explore two supervised learning methods, namely, the support vector machines (SVM) and the decision tree (DT) methods, to develop predictive models for classifying pre-contrast cine SAX images as being a case of MI or healthy. ResultsOverall, for the UNet segmentation model, the performance based on the mean Dice score for the test set (n = 108) is 0.75 (+/- 0.20) for the endocardium, 0.51 (+/- 0.21) for the epicardium and 0.20 (+/- 0.17) for the scar. For the classification task, the accuracy, F1 and precision scores of 0.68, 0.69, and 0.64, respectively, were achieved with the SVM model, and of 0.62, 0.63, and 0.72, respectively, with the DT model. ConclusionWe have presented some promising approaches involving DL, SVM, and DT methods in an attempt to accurately predict contrast information from non-contrast images. While our initial results are modest for this challenging task, this area of research still poses several open problems.
更多查看译文
关键词
CMR, contrast, contrast-free, deep learning, machine learning, support vector machines, decision tree
AI 理解论文
溯源树
样例
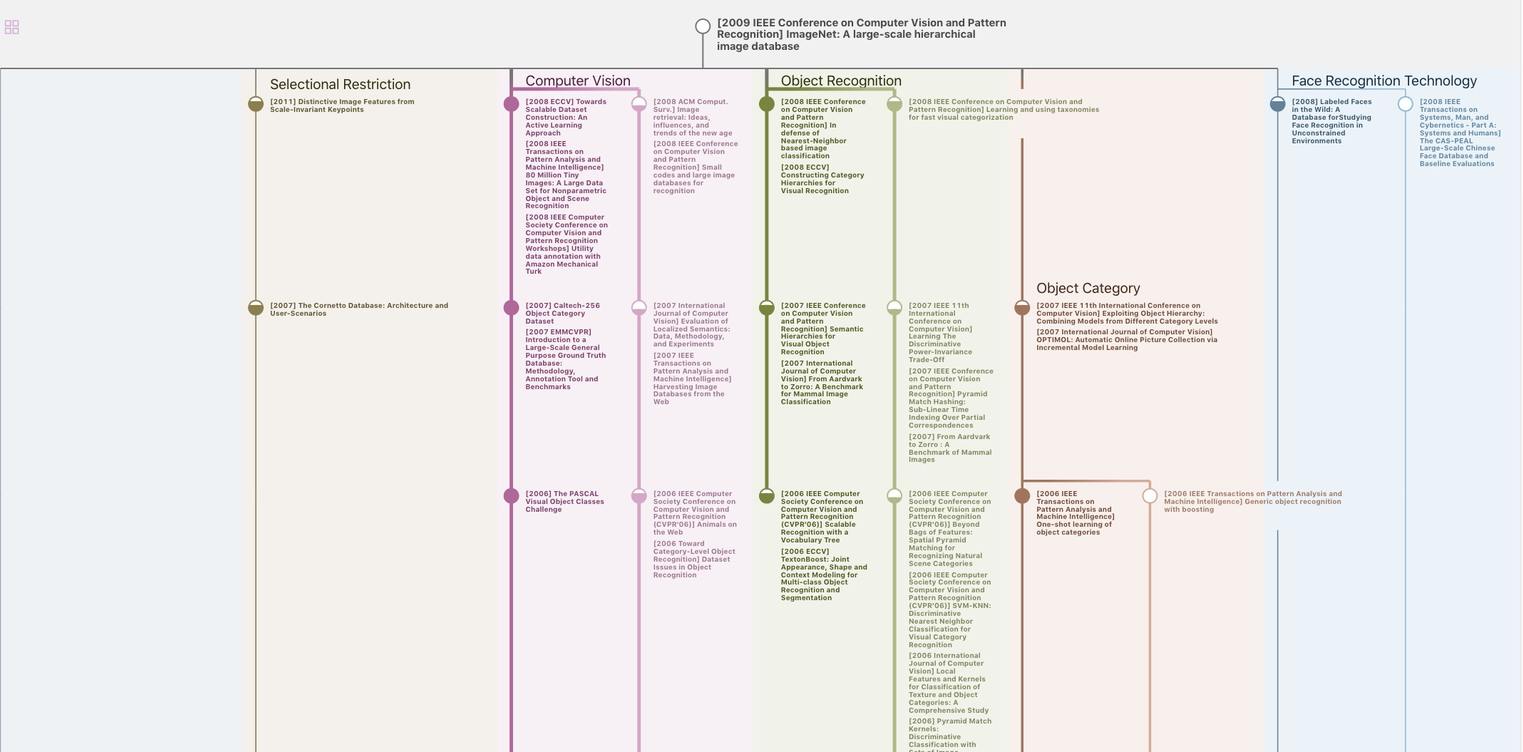
生成溯源树,研究论文发展脉络
Chat Paper
正在生成论文摘要