A novel adaptive cubic quasi‐newton optimizer for deep learning based medical image analysis tasks, validated on detection of COVID‐19 and segmentation for COVID‐19 lung infection, liver tumor, and optic disc/cup
Medical Physics(2022)
摘要
Background: Most of existing deep learning research in medical image analysis is focused on networks with stronger performance. These networks have achieved success, while their architectures are complex and even contain massive parameters ranging from thousands to millions in numbers. The nature of high dimension and nonconvex makes it easy to train a suboptimal model through the popular stochastic first-order optimizers, which only use gradient information. Purpose Our purpose is to design an adaptive cubic quasi-Newton optimizer, which could help to escape from suboptimal solution and improve the performance of deep neural networks on four medical image analysis tasks including: detection of COVID-19, COVID-19 lung infection segmentation, liver tumor segmentation, optic disc/cup segmentation. Methods: In this work, we introduce a novel adaptive cubic quasi-Newton optimizer with high-order moment (termed ACQN-H) for medical image analysis. The optimizer dynamically captures the curvature of the loss function by diagonally approximated Hessian and the norm of difference between previous two estimates, which helps to escape from saddle points more efficiently. In addition, to reduce the variance introduced by the stochastic nature of the problem, ACQN-H hires high-order moment through exponential moving average on iteratively calculated approximated Hessian matrix. Extensive experiments are performed to access the performance of ACQN-H. These include detection of COVID-19 using COVID-Net on dataset COVID-chestxray, which contains 16 565 training samples and 1841 test samples; COVID-19 lung infection segmentation using Inf-Net on COVID-CT, which contains 45, 5, and 5 computer tomography (CT) images for training, validation, and testing, respectively; liver tumor segmentation using ResUNet on LiTS2017, which consists of 50 622 abdominal scan images for training and 26 608 images for testing; optic disc/cup segmentation using MRNet on RIGA, which has 655 color fundus images for training and 95 for testing. The results are compared with commonly used stochastic first-order optimizers such as Adam, SGD, and AdaBound, and recently proposed stochastic quasi-Newton optimizer Apollo. In task detection of COVID-19, we use classification accuracy as the evaluation metric. For the other three medical image segmentation tasks, seven commonly used evaluation metrics are utilized, that is, Dice, structure measure, enhanced-alignment measure (EM), mean absolute error (MAE), intersection over union (IoU), true positive rate (TPR), and true negative rate. Results: Experiments on four tasks show that ACQN-H achieves improvements over other stochastic optimizers: (1) comparing with AdaBound, ACQN-H achieves 0.49%, 0.11%, and 0.70% higher accuracy on the COVID-chestxray dataset using network COVID-Net with VGG16, ResNet50 and DenseNet121 as backbones, respectively; (2) ACQN-H has the best scores in terms of evaluation metrics Dice, TPR, EM, and MAE on COVID-CT dataset using network Inf-Net. Particularly, ACQN-H achieves 1.0% better Dice as compared to Apollo; (3) ACQN-H achieves the best results on LiTS2017 dataset using network ResUNet, and outperforms Adam in terms of Dice by 2.3%; (4) ACQN-H improves the performance of network MRNet on RIGA dataset, and achieves 0.5% and 1.0% better scores on cup segmentation for Dice and IoU, respectively, compared with SGD. We also present fivefold validation results of four tasks. It can be found that the results on detection of COVID-19, liver tumor segmentation and optic disc/cup segmentation can achieve high performance with low variance. For COVID-19 lung infection segmentation, the variance on test set is much larger than on validation set, which may due to small size of dataset. Conclusions: The proposed optimizer ACQN-H has been validated on four medical image analysis tasks including: detection of COVID-19 using COVID-Net on COVID-chestxray, COVID-19 lung infection segmentation using Inf-Net on COVID-CT, liver tumor segmentation using ResUNet on LiTS2017, optic disc/cup segmentation using MRNet on RIGA. Experiments show that ACQN-H can achieve some performance improvement. Moreover, the work is expected to boost the performance of existing deep learning networks in medical image analysis.
更多查看译文
关键词
cubic quasi-Newton optimizer,high-order moment,medical image analysis
AI 理解论文
溯源树
样例
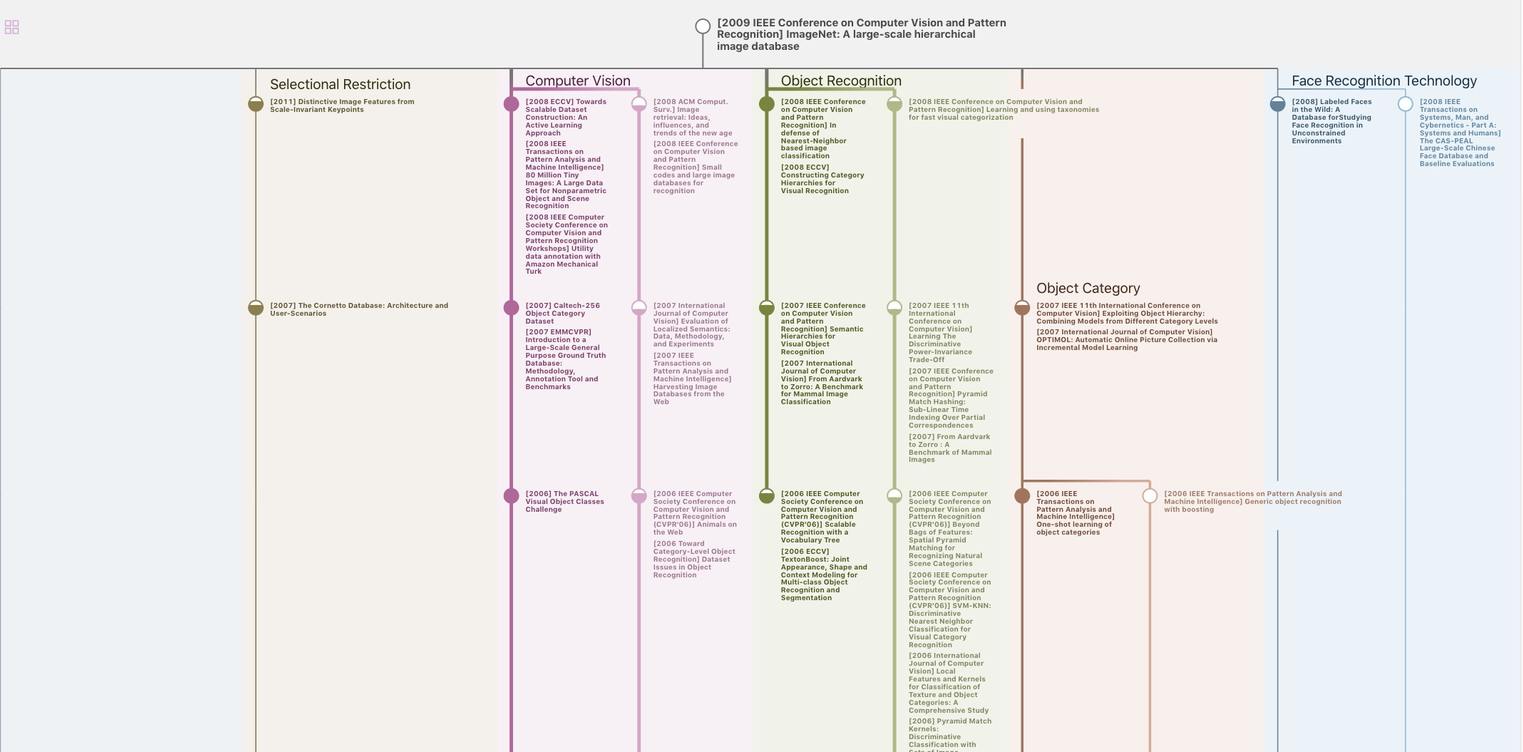
生成溯源树,研究论文发展脉络
Chat Paper
正在生成论文摘要