Pattern classification based on regional models
Applied Soft Computing(2022)
摘要
In a supervised setting, the global classification paradigm leverages the whole training data to produce a single class discriminative model. Alternatively, the local classification approach builds multiple base classifiers, each of them using a small subset of the training data. In this paper, we take a path to stand in-between the global and local approaches. We introduce a two-level clustering-based method in which base classifiers operate on a larger portion of the input space than in the traditional local paradigm. In particular, we first obtain a grained input representation by employing a Self-Organizing Map (SOM) to the inputs. We then apply a clustering algorithm (e.g., K-Means) to the SOM units to define input regions — a subset of input samples associated with a specific cluster of SOM units. We refer to this approach as regional classification. We demonstrate the effectiveness of regional classification on several benchmarks. Also, we study the impact of (1) adopting linear and nonlinear base classifiers (e.g., least squares support vector machines) and (2) using cluster validation indexes to determine the optimal number of clusters. Based on the experiments, the regional classification approach achieves competitive performance compared to its global and local counterparts, especially when equipped with linear base classifiers.
更多查看译文
关键词
Pattern classification,Local models,Regional models,Self-Organizing Maps,Least squares support vector machine
AI 理解论文
溯源树
样例
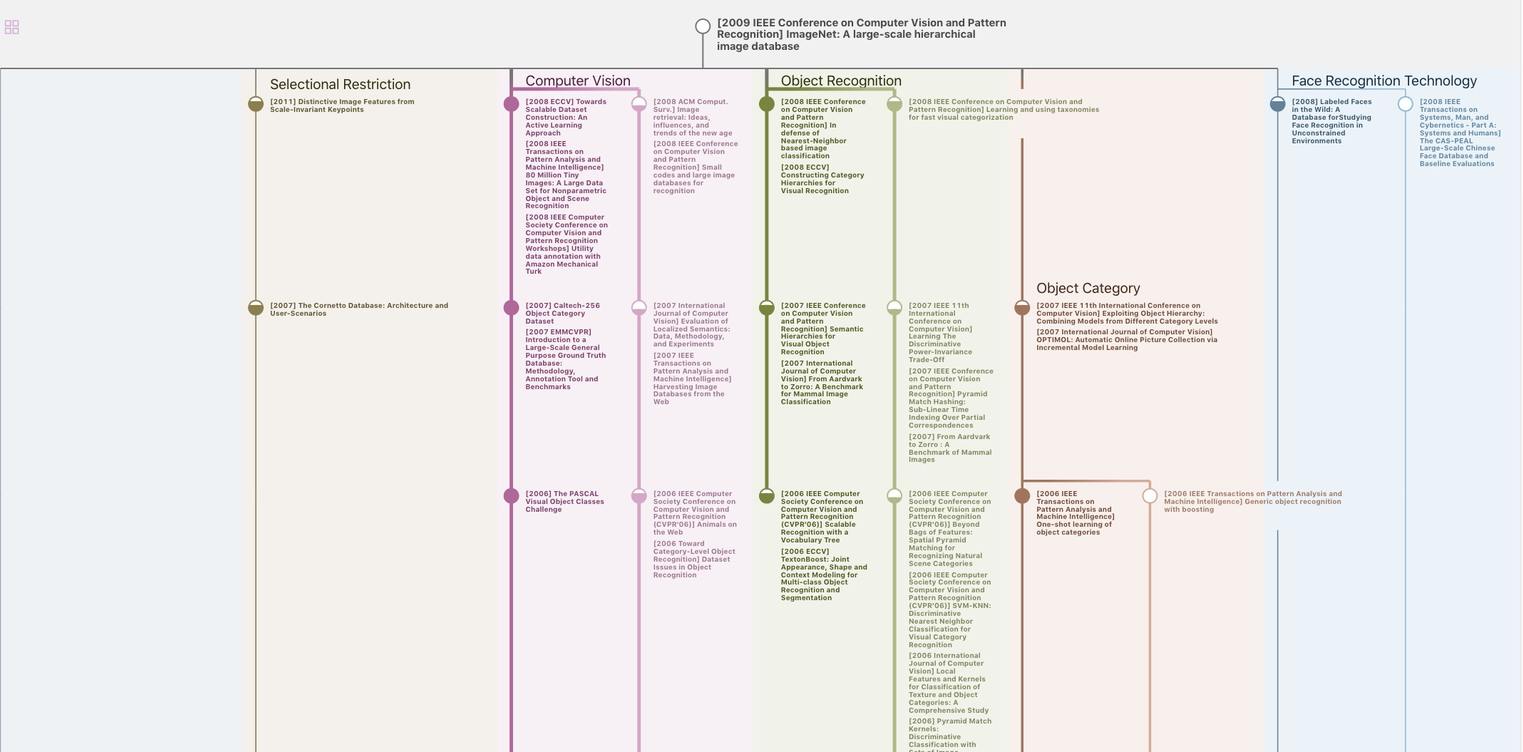
生成溯源树,研究论文发展脉络
Chat Paper
正在生成论文摘要