YOLO networks for polyp detection: A human-in-the-loop training approach
Current Directions in Biomedical Engineering(2022)
摘要
Abstract Introduction: Early detection of adenomas and polyps is one central goal of colonoscopic screening programs. As the adenoma detection rate (ADR) depends on the experience of the endoscopist, AI-based polyp detection systems can be used for real-time assistance. Hence, to support the physicians such AI-based systems using deep-convolutional neural networks (DCNNS) have been introduced in the past years. One disadvantage of these techniques is the need of a huge amount of labeled training data. Method: We investigate a "human-in-the-loop approach" to minimize the required time to generate labeled training data. The approach is evaluated within the training a YOLOv4 neural network to detect polyps in colonoscopic image data. The performance metrics of the neural network are evaluated on three public datasets. Results: The performance of the YOLO network increased from a precision of 0.88, recall of 0.83, F1 score of 0.86, and a F2 score of 0.86 to a precision of 0.91, recall of 0.87, F1 = of 0.89, and F2 = of 0.88. The interactive labelling of 1,000 images only takes one hours. Conclusion: The proposed "human-in-theloop approach" is capable of generating labelled image data in a minimum of time while increasing the performance metrics as well. For higher performance increase more data can now be labeled within this new approach.
更多查看译文
关键词
colonoscopy,polyp detection,yolo networks,deep learning
AI 理解论文
溯源树
样例
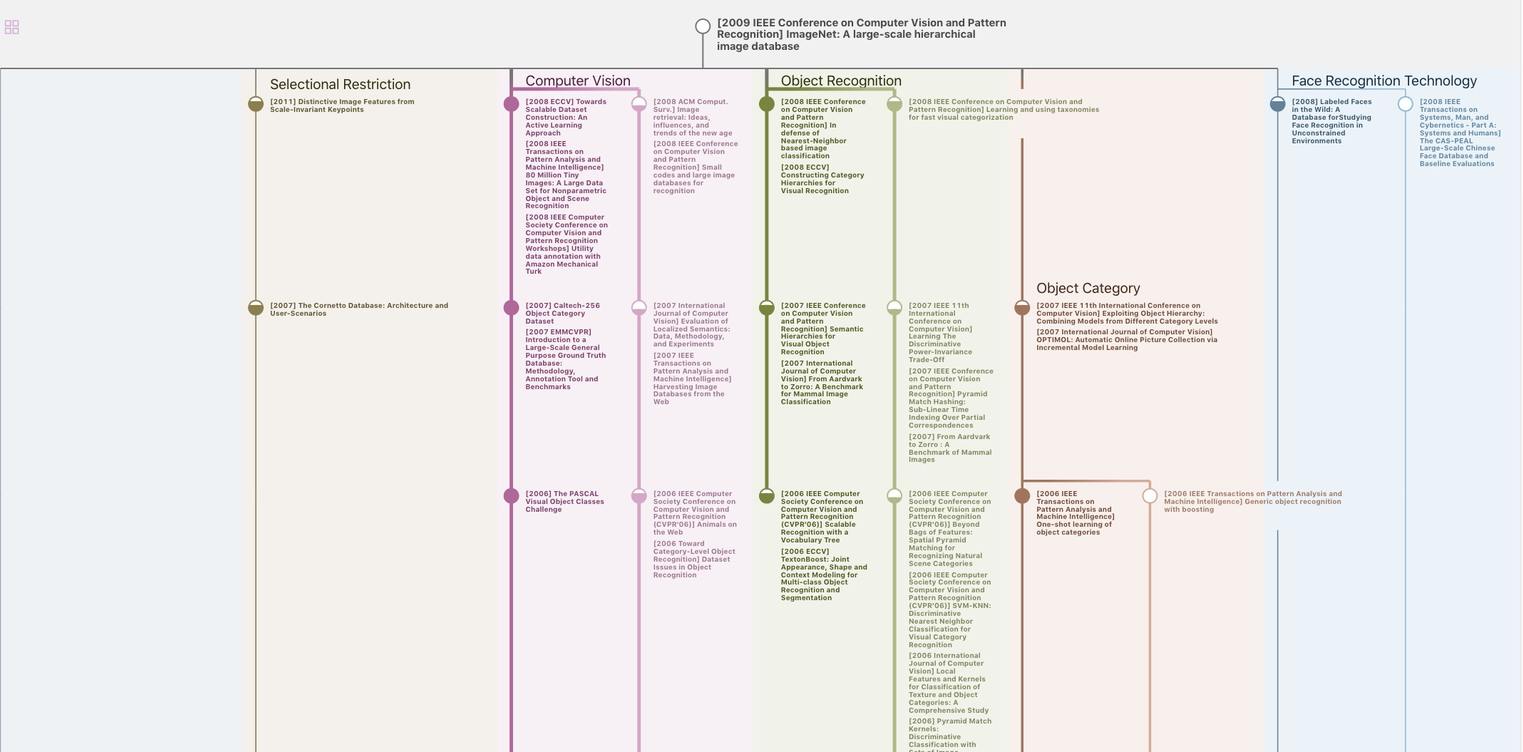
生成溯源树,研究论文发展脉络
Chat Paper
正在生成论文摘要