Learning Perturbations for Soft-Output Linear MIMO Demappers.
GLOBECOM(2022)
摘要
Tree-based demappers for multiple-input multiple-output (MIMO) detection such as the sphere decoder can achieve near-optimal performance but incur high computational cost due to their sequential nature. In this paper, we propose the perturbed linear demapper (PLM), which is a novel data-driven model for computing soft outputs in parallel. To achieve this, the PLM learns a distribution centered on an initial linear estimate and a log-likelihood ratio clipping parameter using end-to-end Bayesian optimization. Furthermore, we show that lattice-reduction can be naturally incorporated into the PLM pipeline, which allows to trade off computational cost against coded block error rate reduction. We find that the optimized PLM can achieve near maximum-likelihood (ML) performance in Rayleigh channels, making it an efficient alternative to tree-based demappers.
更多查看译文
关键词
Soft-output MIMO detection, Lattice Reduction, Subset Reduction, Bayesian Optimization
AI 理解论文
溯源树
样例
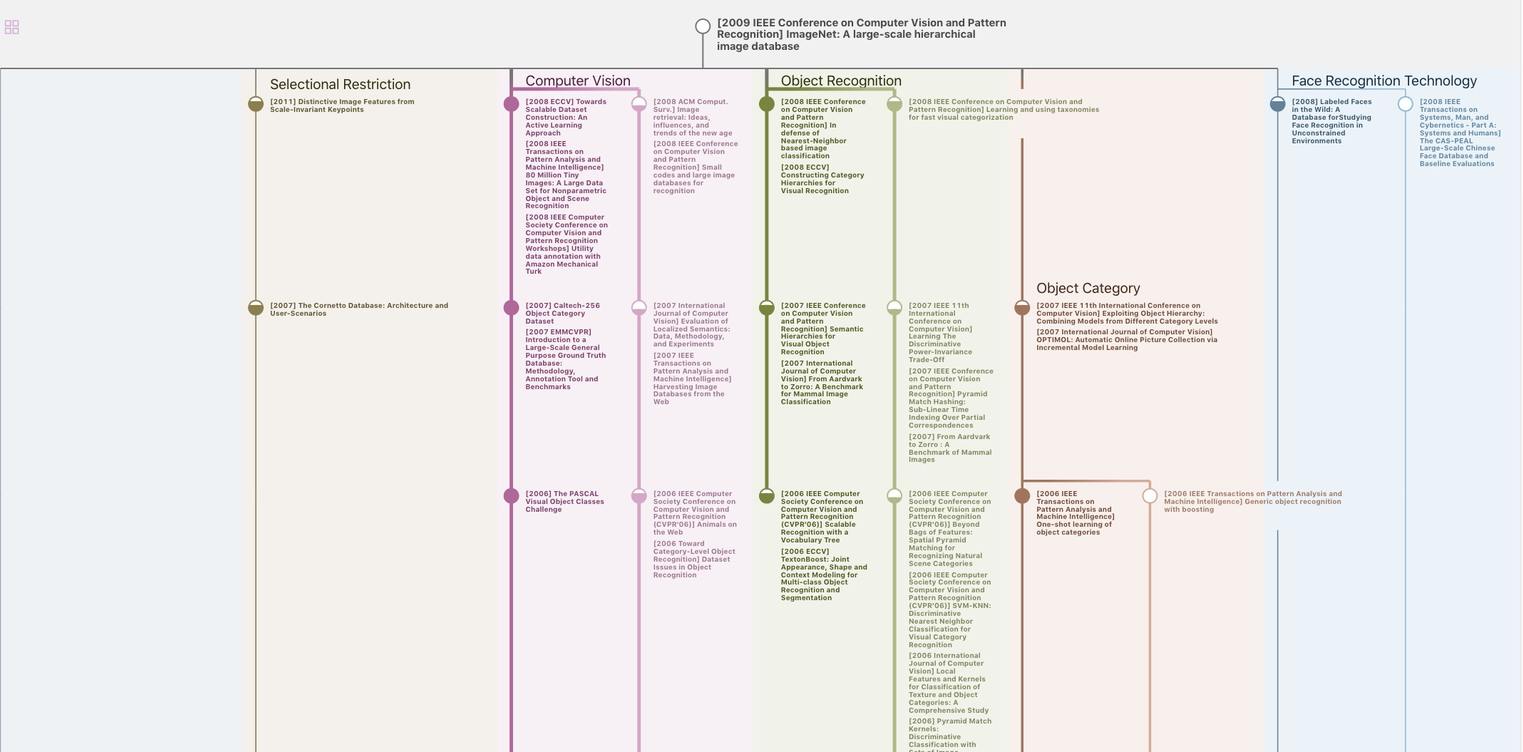
生成溯源树,研究论文发展脉络
Chat Paper
正在生成论文摘要