Unsupervised EHR-based Phenotyping via Matrix and Tensor Decompositions
arxiv(2022)
摘要
Computational phenotyping allows for unsupervised discovery of subgroups of patients as well as corresponding co-occurring medical conditions from electronic health records (EHR). Typically, EHR data contains demographic information, diagnoses and laboratory results. Discovering (novel) phenotypes has the potential to be of prognostic and therapeutic value. Providing medical practitioners with transparent and interpretable results is an important requirement and an essential part for advancing precision medicine. Low-rank data approximation methods such as matrix (e.g., non-negative matrix factorization) and tensor decompositions (e.g., CANDECOMP/PARAFAC) have demonstrated that they can provide such transparent and interpretable insights. Recent developments have adapted low-rank data approximation methods by incorporating different constraints and regularizations that facilitate interpretability further. In addition, they offer solutions for common challenges within EHR data such as high dimensionality, data sparsity and incompleteness. Especially extracting temporal phenotypes from longitudinal EHR has received much attention in recent years. In this paper, we provide a comprehensive review of low-rank approximation-based approaches for computational phenotyping. The existing literature is categorized into temporal vs. static phenotyping approaches based on matrix vs. tensor decompositions. Furthermore, we outline different approaches for the validation of phenotypes, i.e., the assessment of clinical significance.
更多查看译文
关键词
electronic health records (EHR),low-rank approximations,matrix,tensor decompositions,phenotyping,temporal phenotyping
AI 理解论文
溯源树
样例
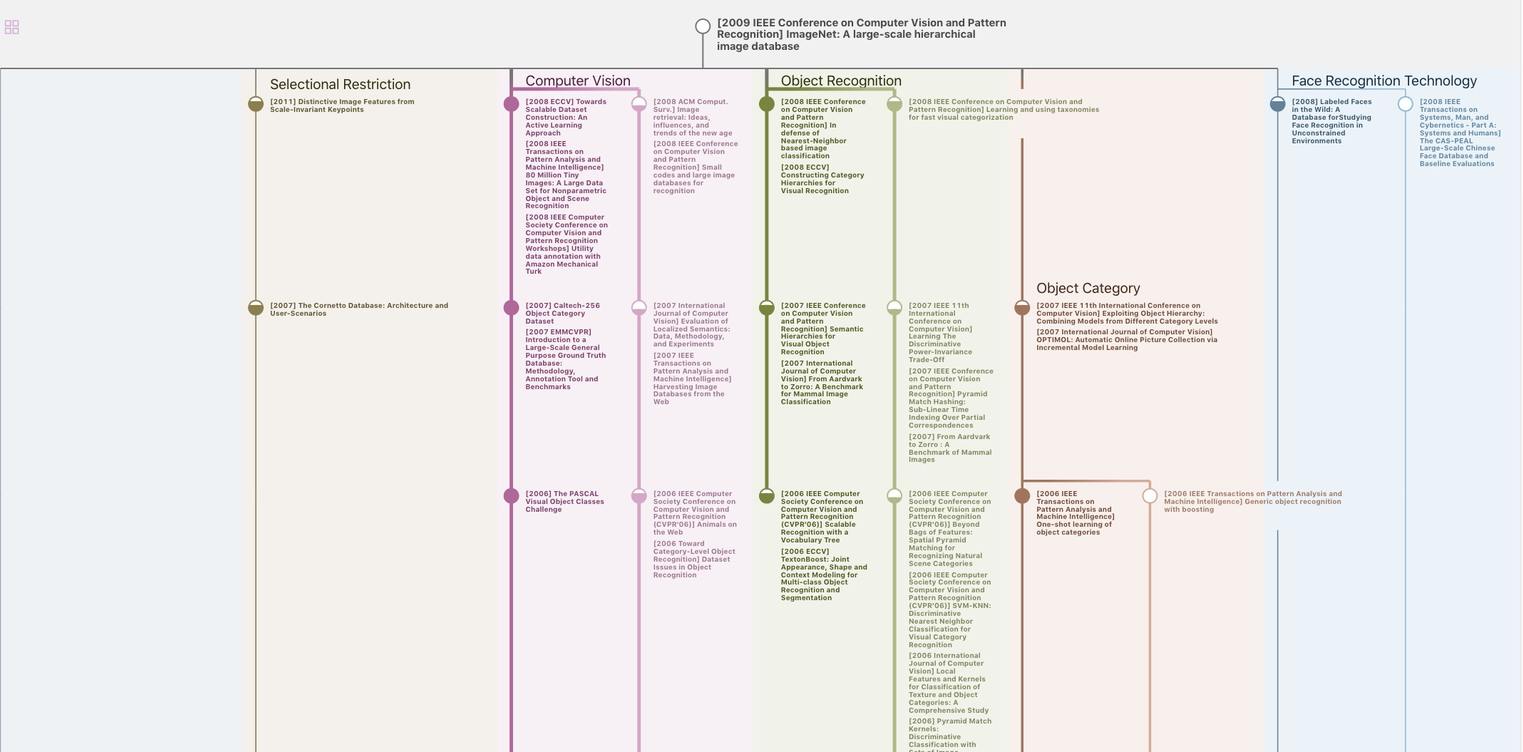
生成溯源树,研究论文发展脉络
Chat Paper
正在生成论文摘要