Tradeoffs in Resampling and Filtering for Imbalanced Classification
arxiv(2022)
摘要
Imbalanced classification problems are extremely common in natural language processing and are solved using a variety of resampling and filtering techniques, which often involve making decisions on how to select training data or decide which test examples should be labeled by the model. We examine the tradeoffs in model performance involved in choices of training sample and filter training and test data in heavily imbalanced token classification task and examine the relationship between the magnitude of these tradeoffs and the base rate of the phenomenon of interest. In experiments on sequence tagging to detect rare phenomena in English and Arabic texts, we find that different methods of selecting training data bring tradeoffs in effectiveness and efficiency. We also see that in highly imbalanced cases, filtering test data using first-pass retrieval models is as important for model performance as selecting training data. The base rate of a rare positive class has a clear effect on the magnitude of the changes in performance caused by the selection of training or test data. As the base rate increases, the differences brought about by those choices decreases.
更多查看译文
关键词
resampling,classification,filtering
AI 理解论文
溯源树
样例
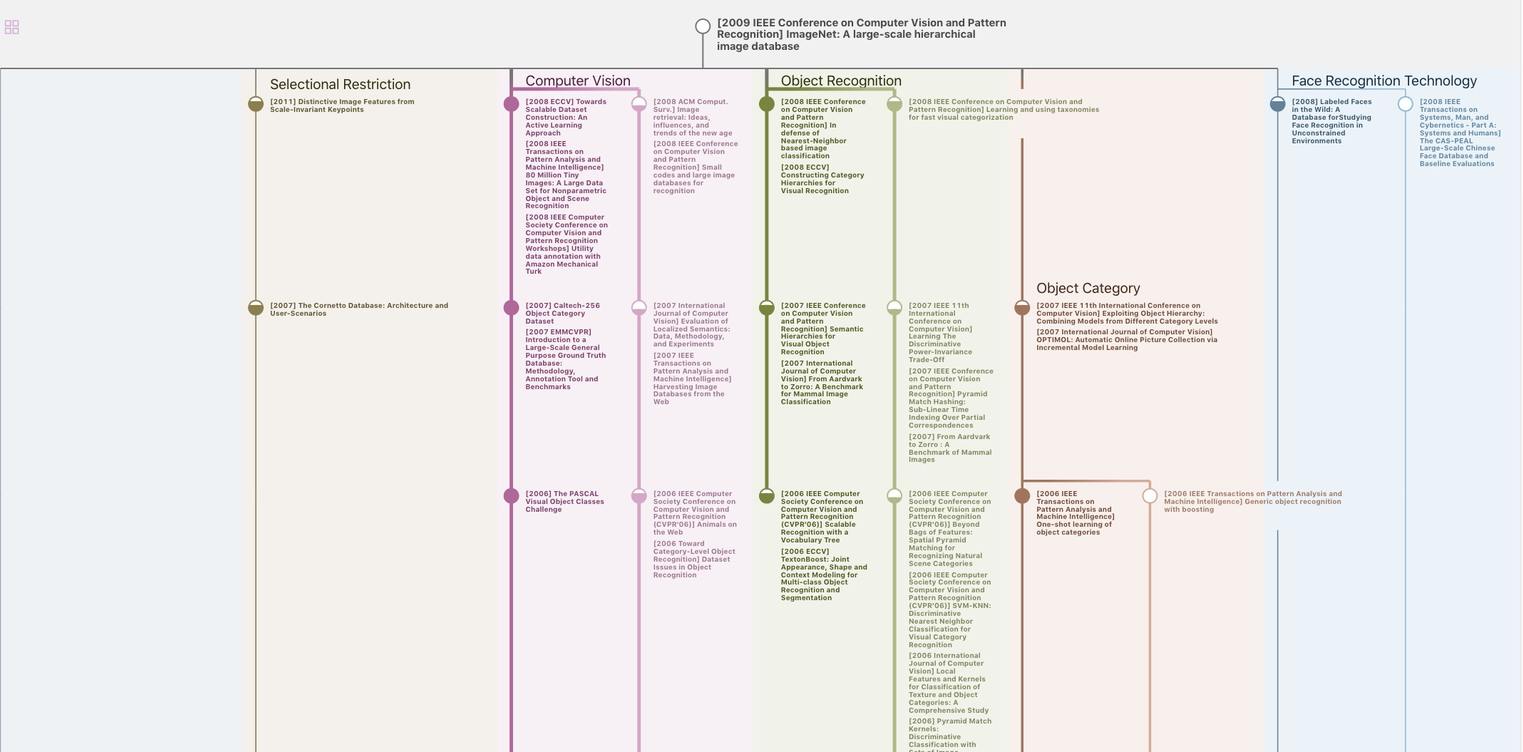
生成溯源树,研究论文发展脉络
Chat Paper
正在生成论文摘要