A general framework for the analysis of kernel-based tests
arxiv(2022)
摘要
Kernel-based tests provide a simple yet effective framework that use the theory of reproducing kernel Hilbert spaces to design non-parametric testing procedures. In this paper we propose new theoretical tools that can be used to study the asymptotic behaviour of kernel-based tests in several data scenarios, and in many different testing problems. Unlike current approaches, our methods avoid using lengthy $U$ and $V$ statistics expansions and limit theorems, that commonly appear in the literature, and works directly with random functionals on Hilbert spaces. Therefore, our framework leads to a much simpler and clean analysis of kernel tests, only requiring mild regularity conditions. Furthermore, we show that, in general, our analysis cannot be improved by proving that the regularity conditions required by our methods are both sufficient and necessary. To illustrate the effectiveness of our approach we present a new kernel-test for the conditional independence testing problem, as well as new analyses for already known kernel-based tests.
更多查看译文
关键词
tests,analysis,general framework,kernel-based
AI 理解论文
溯源树
样例
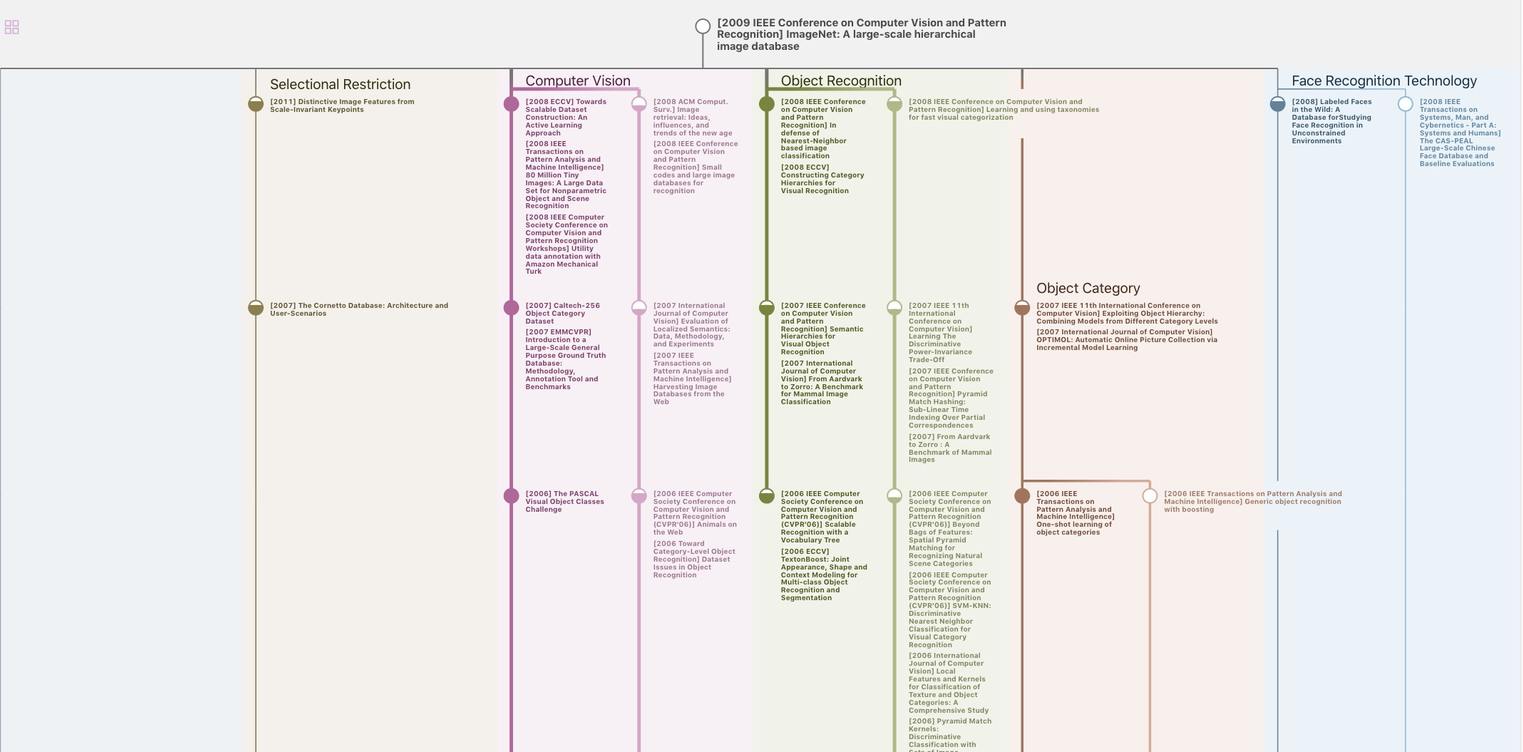
生成溯源树,研究论文发展脉络
Chat Paper
正在生成论文摘要