Autoregressive Predictive Coding: A Comprehensive Study
IEEE Journal of Selected Topics in Signal Processing(2022)
摘要
We review autoregressive predictive coding (APC), an approach to learn speech representation by predicting a future frame given the past frames. We present three different views of interpreting APC, and provide a historical account to the approach. To study the speech representation learned by APC, we use common speech tasks, such as automatic speech recognition and speaker verification, to demonstrate the utility of the learned representation. In addition, we design a suite of fine-grained tasks, including frame classification, segment classification, fundamental frequency tracking, and duration prediction, to probe the phonetic and prosodic content of the representation. The three views of the APC objective welcome various generalizations and algorithms to learn speech representations. Probing on the suite of fine-grained tasks suggests that APC makes a wide range of high-level speech information accessible in its learned representation.
更多查看译文
关键词
Automatic speech recognition,predictive coding,representation learning,self-supervised learning,speaker verification
AI 理解论文
溯源树
样例
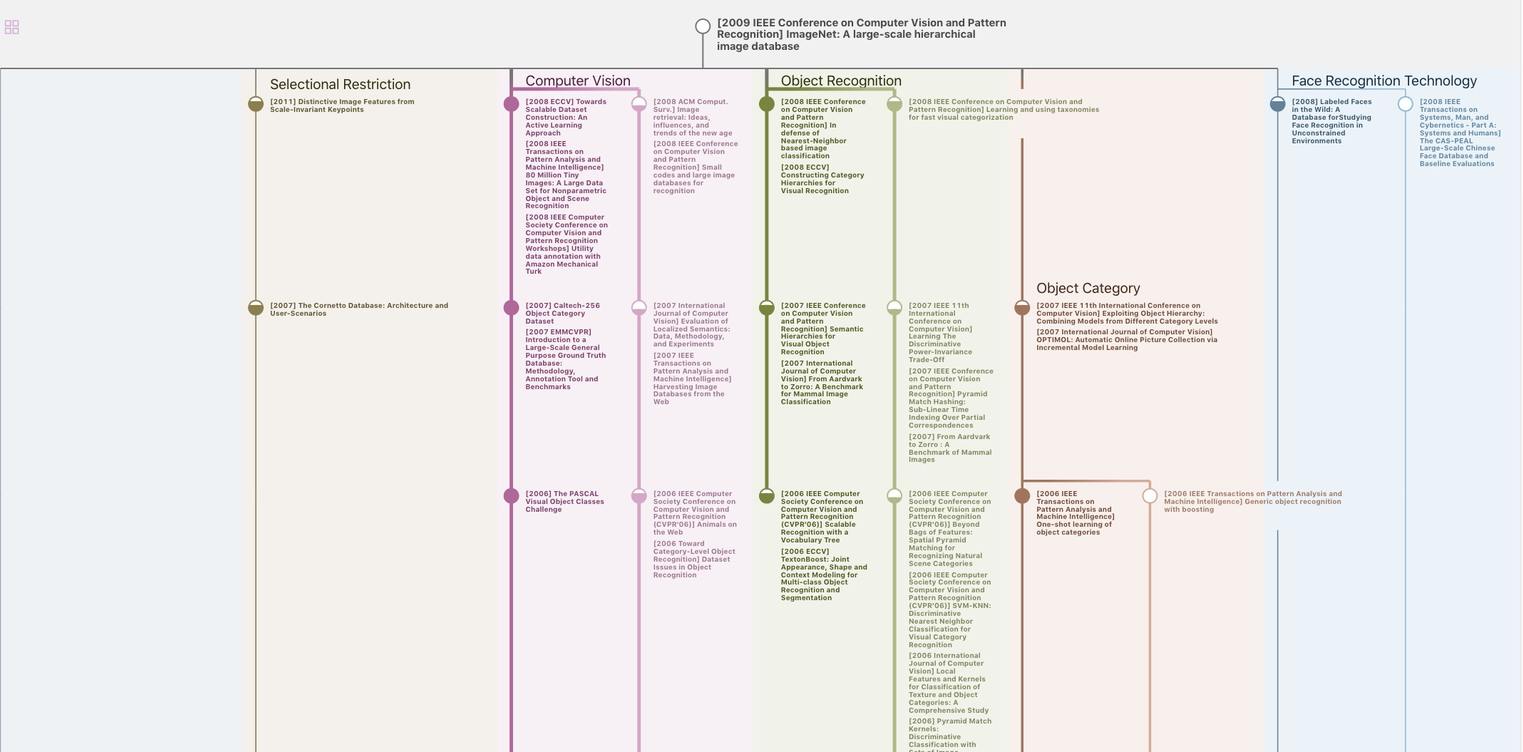
生成溯源树,研究论文发展脉络
Chat Paper
正在生成论文摘要