Assessing Alternative Imputation Strategies for Infrequently Missing Items on Multi-item Scales.
Communications in Statistics: Case Studies, Data Analysis and Applications(2022)
摘要
Health-science researchers often measure psychological constructs using multi-item scales and encounter missing items on some participants. Multiple imputation (MI) has emerged as an alternative to ad-hoc methods (e.g., mean substitution) for handling incomplete data on multi-item scales, appealingly reflecting available information while accounting for uncertainty due to missing values in a unified inferential framework. However, MI can be implemented in a variety of ways. When the number of variables to impute gets large, some strategies yield unstable estimates of quantities of interest while others are not technically feasible to implement. These considerations raise pragmatic questions about the extent to which ad-hoc procedures would yield statistical properties that are competitive with theoretically motivated methods. Drawing on an HIV study where depression and anxiety symptoms are measured with multi-item scales, this empirical investigation contrasts ad-hoc methods for handling missing items with various MI implementations that differ as to whether imputation is at the item-level or scale-level and how auxiliary variables are incorporated. While the findings are consistent with previous reports favoring item-level imputation when feasible to implement, we found only subtle differences in statistical properties across procedures, suggesting that weaknesses of ad-hoc procedures may be muted when missing data percentages are modest.
更多查看译文
关键词
Missing data,Multi-item scale,Multiple imputation
AI 理解论文
溯源树
样例
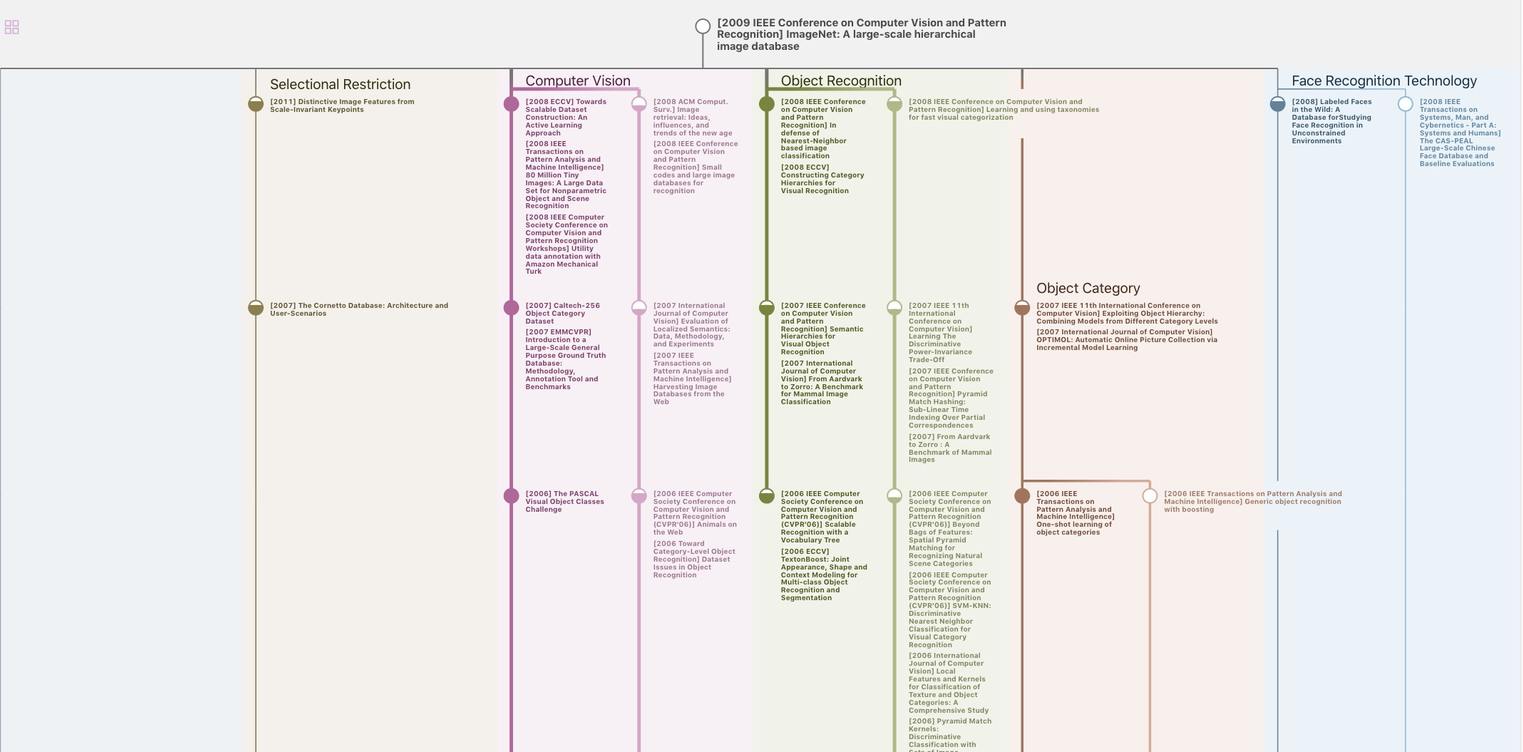
生成溯源树,研究论文发展脉络
Chat Paper
正在生成论文摘要