Improving RGB-D Point Cloud Registration by Learning Multi-scale Local Linear Transformation.
European Conference on Computer Vision(2022)
摘要
Point cloud registration aims at estimating the geometric transformation between two point cloud scans, in which point-wise correspondence estimation is the key to its success. In addition to previous methods that seek correspondences by hand-crafted or learnt geometric features, recent point cloud registration methods have tried to apply RGB-D data to achieve more accurate correspondence. However, it is not trivial to effectively fuse the geometric and visual information from these two distinctive modalities, especially for the registration problem. In this work, we propose a new Geometry-Aware Visual Feature Extractor (GAVE) that employs multi-scale local linear transformation to progressively fuse these two modalities, where the geometric features from the depth data act as the geometry-dependent convolution kernels to transform the visual features from the RGB data. The resultant visual-geometric features are in canonical feature spaces with alleviated visual dissimilarity caused by geometric changes, by which more reliable correspondence can be achieved. The proposed GAVE module can be readily plugged into recent RGB-D point cloud registration framework. Extensive experiments on 3D Match and ScanNet demonstrate that our method outperforms the state-of-the-art point cloud registration methods even without correspondence or pose supervision.
更多查看译文
关键词
Point cloud registration,Geometric-visual feature extractor,Local linear transformation
AI 理解论文
溯源树
样例
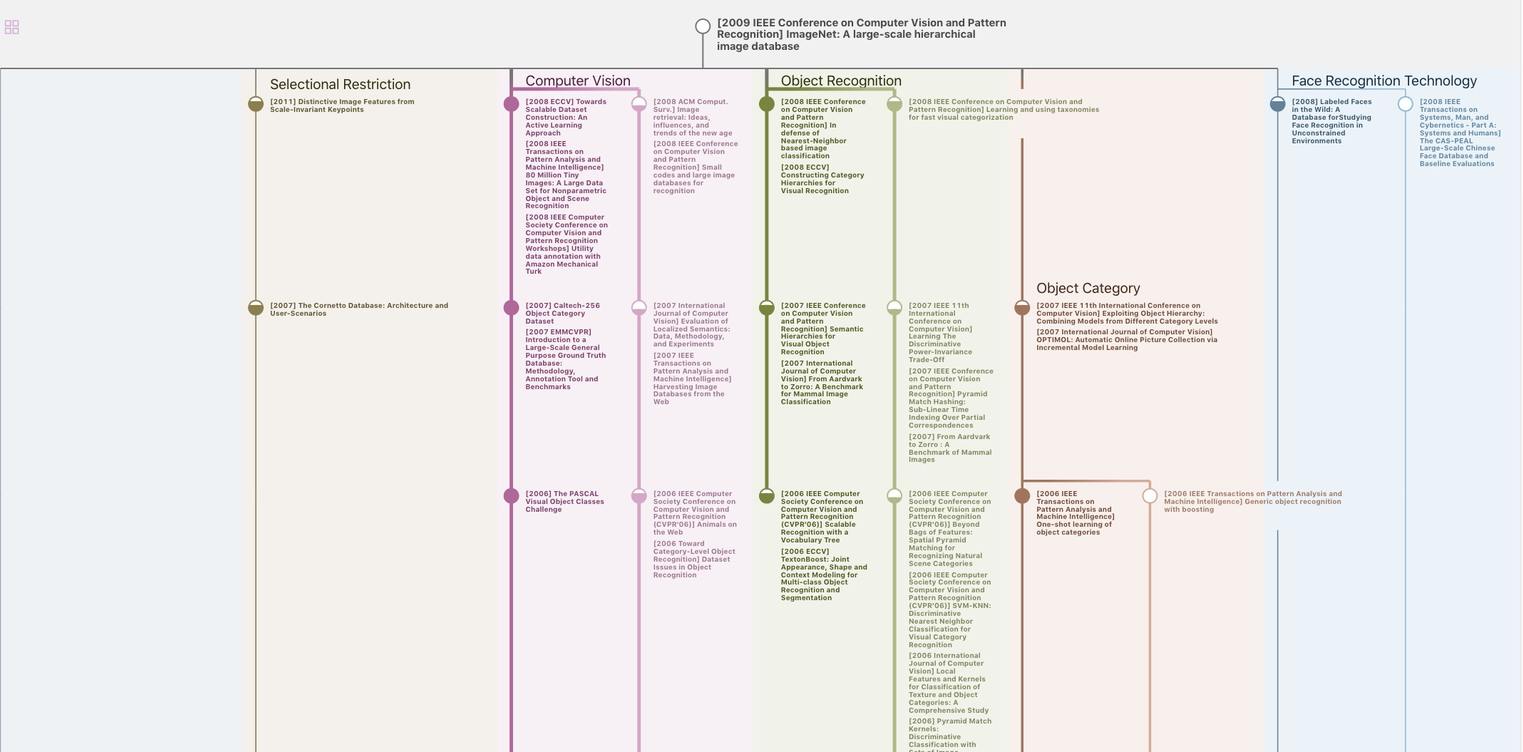
生成溯源树,研究论文发展脉络
Chat Paper
正在生成论文摘要