Non-readily identifiable data collaboration analysis for multiple datasets including personal information
arxiv(2023)
摘要
Multi-source data fusion, in which multiple data sources are jointly analyzed to obtain improved information, has attracted considerable research attention. Data confidentiality and cross-institutional communication are critical for the construction of a prediction model using datasets of multiple medical institutions. In such cases, data collaboration (DC) analysis by sharing dimensionality-reduced intermediate representations without iterative cross-institutional communications may be appropriate. Identifiability of the shared data is essential when analyzing data including personal information. In this study, the identifiability of the DC analysis is investigated. The results reveal that the shared intermediate representations are readily identifiable to the original data for supervised learning. This study then proposes a non-readily identifiable DC analysis only sharing non-readily identifiable data for multiple medical datasets including personal information. The proposed method solves identifiability concerns based on a random sample permutation, the concept of interpretable DC analysis, and usage of functions that cannot be reconstructed. In numerical experiments on medical datasets, the proposed method exhibits non-readily identifiability while maintaining a high recognition performance of the conventional DC analysis. The proposed method exhibits a nine percentage point improvement regarding the recognition performance over the local analysis that uses only local dataset for a hospital dataset.
更多查看译文
关键词
Privacy-preserving analysis,Multi-source data fusion,Data collaboration analysis,Personal information,Identifiability,Dimensionality reduction
AI 理解论文
溯源树
样例
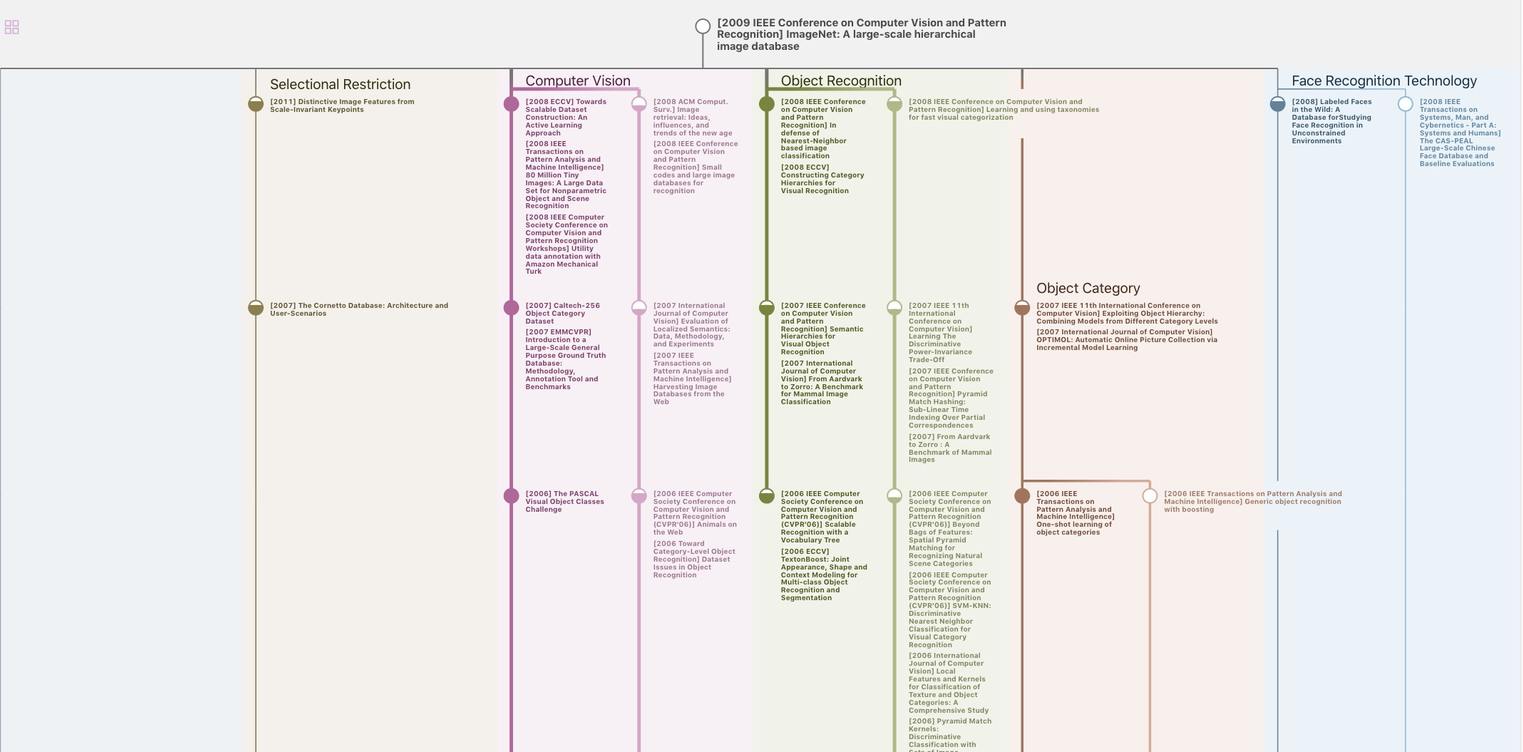
生成溯源树,研究论文发展脉络
Chat Paper
正在生成论文摘要