To Adapt or to Fine-Tune: A Case Study on Abstractive Summarization.
China National Conference on Chinese Computational Linguistics (CCL)(2022)
摘要
Recent advances in the field of abstractive summarization leverage pre-trained language models rather than train a model from scratch. However, such models are sluggish to train and accompanied by a massive overhead. Researchers have proposed a few lightweight alternatives such as smaller adapters to mitigate the drawbacks. Nonetheless, it remains uncertain whether using adapters benefits the task of summarization, in terms of improved efficiency without an unpleasant sacrifice in performance. In this work, we carry out multifaceted investigations on fine-tuning and adapters for summarization tasks with varying complexity: language, domain, and task transfer. In our experiments, fine-tuning a pre-trained language model generally attains a better performance than using adapters; the performance gap positively correlates with the amount of training data used. Notably, adapters exceed fine-tuning under extremely low-resource conditions. We further provide insights on multilinguality, model convergence, and robustness, hoping to shed light on the pragmatic choice of fine-tuning or adapters in abstractive summarization.
更多查看译文
关键词
abstractive summarization,fine-tune
AI 理解论文
溯源树
样例
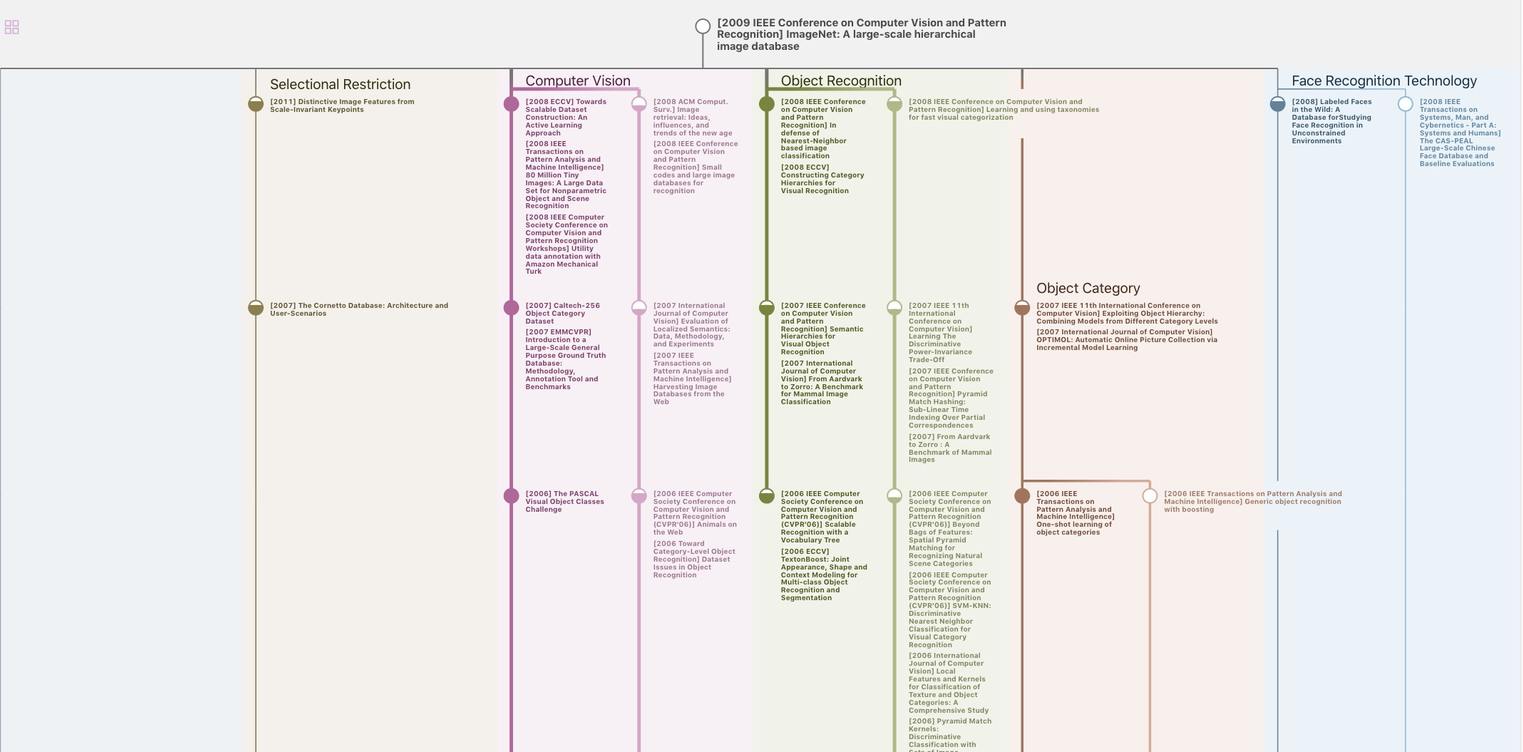
生成溯源树,研究论文发展脉络
Chat Paper
正在生成论文摘要