MultiCoNER: A Large-scale Multilingual Dataset for Complex Named Entity Recognition.
International Conference on Computational Linguistics(2022)
摘要
We present AnonData, a large multilingual dataset for Named Entity Recognition that covers 3 domains (Wiki sentences, questions, and search queries) across 11 languages, as well as multilingual and code-mixing subsets. This dataset is designed to represent contemporary challenges in NER, including low-context scenarios (short and uncased text), syntactically complex entities like movie titles, and long-tail entity distributions. The 26M token dataset is compiled from public resources using techniques such as heuristic-based sentence sampling, template extraction and slotting, and machine translation. We tested the performance of two NER models on our dataset: a baseline XLM-RoBERTa model, and a state-of-the-art NER GEMNET model that leverages gazetteers. The baseline achieves moderate performance (macro-F1=54%). GEMNET, which uses gazetteers, improvement significantly (average improvement of macro-F1=+30%) and demonstrates the difficulty of our dataset. AnonData poses challenges even for large pre-trained language models, and we believe that it can help further research in building robust NER systems.
更多查看译文
关键词
multilingual dataset,complex named entity
AI 理解论文
溯源树
样例
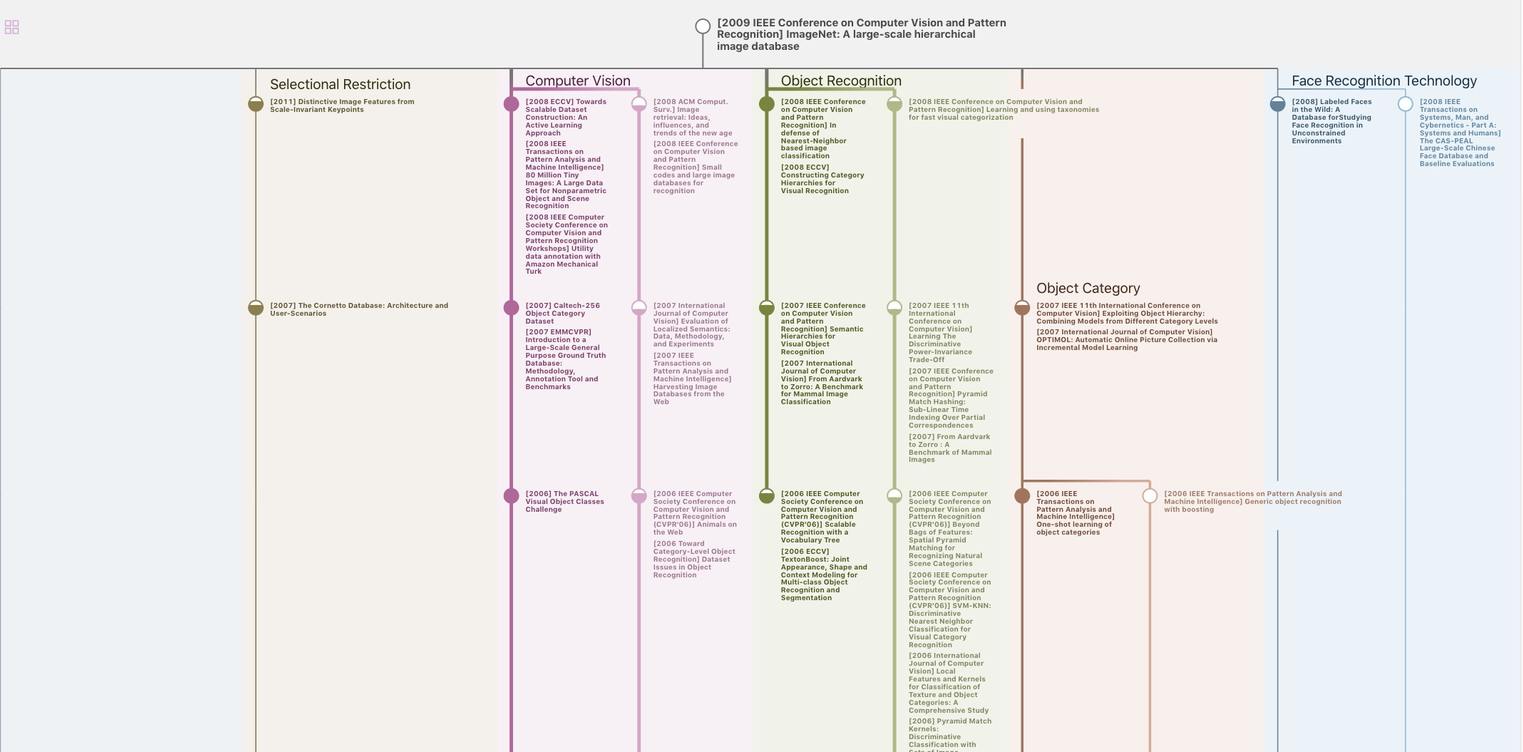
生成溯源树,研究论文发展脉络
Chat Paper
正在生成论文摘要