Machine learning models for photonic crystals band diagram prediction and gap optimisation
SSRN Electronic Journal(2022)
摘要
Data-driven methods of machine learning (ML) have attracted a lot of interest in various fields of physics. Inverse design and optimisation of structured optical metamaterials such as photonic crystals, metasurfaces, and other nanostructured components seem to benefit a lot from this approach in the nearest future. Here we develop several approaches to use ML methods to predict and optimise properties of photonic crystals (e.g. size of bandgaps) effectively. We use a dataset of 2D photonic crystals produced recently in [T.Christinsen et al., Nanophotonics 9, 4183 (2020)]. For improving performance of predictive models, we apply symmetry-aware augmentations and hybrid ML-solver approaches. As a result, considerable improvement in prediction accuracy could be achieved as compared to baseline models. For generative models, we apply variational autoencoders (VAEs) combined with predictor architecture, inspired by related works in chemical design realm. By using latent space optimisation, we achieve good results in the task of increasing bandgaps of photonic structures. The approach seems to be very promising and can be extended to 3D geometries.
更多查看译文
关键词
Photonic crystals,Photonic bandgap materials,Neural networks,Machine learning
AI 理解论文
溯源树
样例
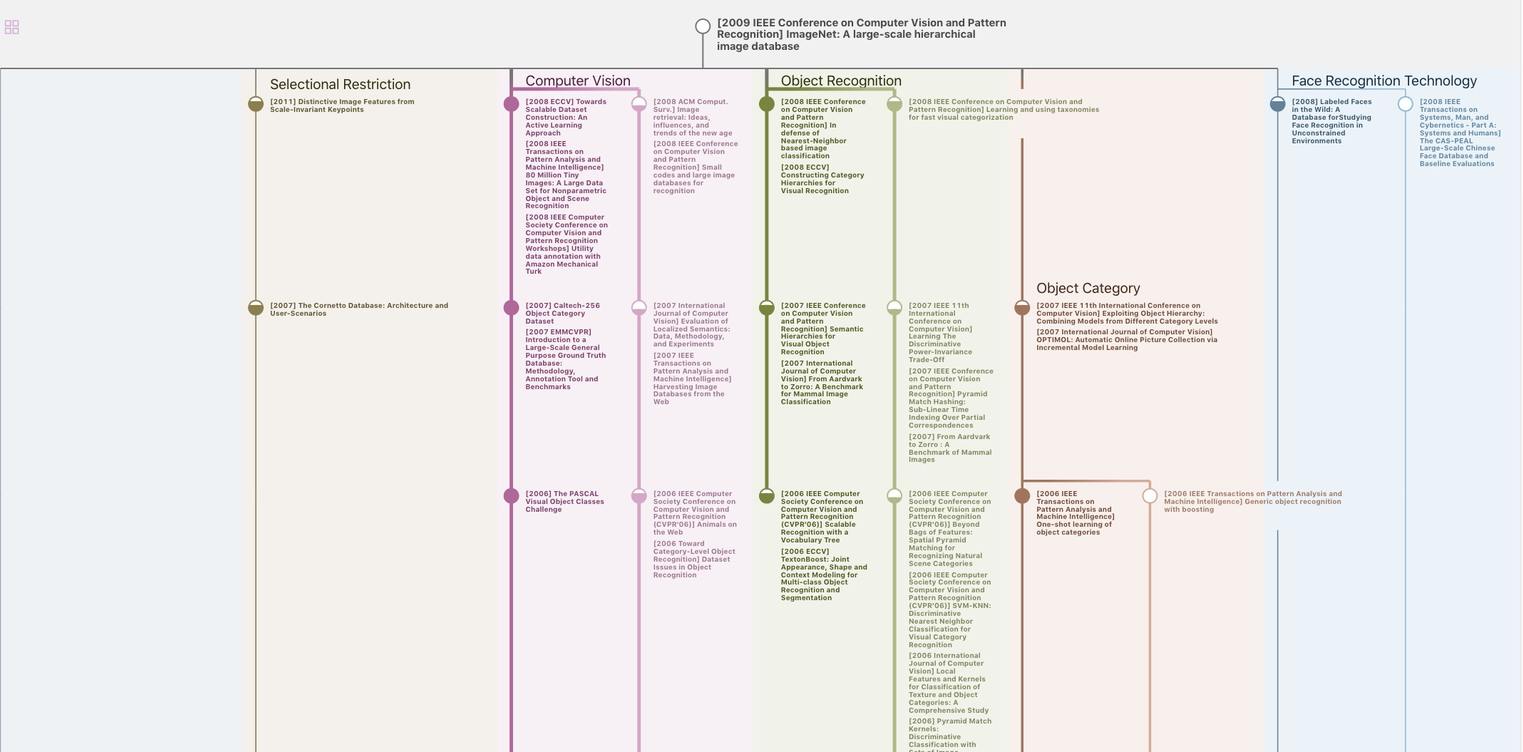
生成溯源树,研究论文发展脉络
Chat Paper
正在生成论文摘要