Improved seasonal prediction of harmful algal blooms in Lake Erie using large-scale climate indices
COMMUNICATIONS EARTH & ENVIRONMENT(2022)
摘要
Harmful Algal Blooms lead to multi-billion-dollar losses in the United States due to shellfish closures, fish mortalities, and reluctance to consume seafood. Therefore, an improved early seasonal prediction of harmful algal blooms severity is important. Conventional methods for harmful algal blooms prediction using nutrient loading as the primary driver have been found to be less accurate during extreme bloom years. Here we show that a machine learning approach using observed nutrient loading, and large-scale climate indices can improve the harmful algal blooms prediction in Lake Erie. Moreover, the seasonal prediction of harmful algal blooms can be completed by early June, before the expected peak in harmful algal bloom activity from July to October. This improved early seasonal prediction can provide timely information to policymakers for adopting proper planning and mitigation strategies such as restrictions in harvesting and help in monitoring toxins in shellfish to keep contaminated products off the market.
更多查看译文
关键词
harmful algal blooms,lake erie,seasonal prediction,large-scale
AI 理解论文
溯源树
样例
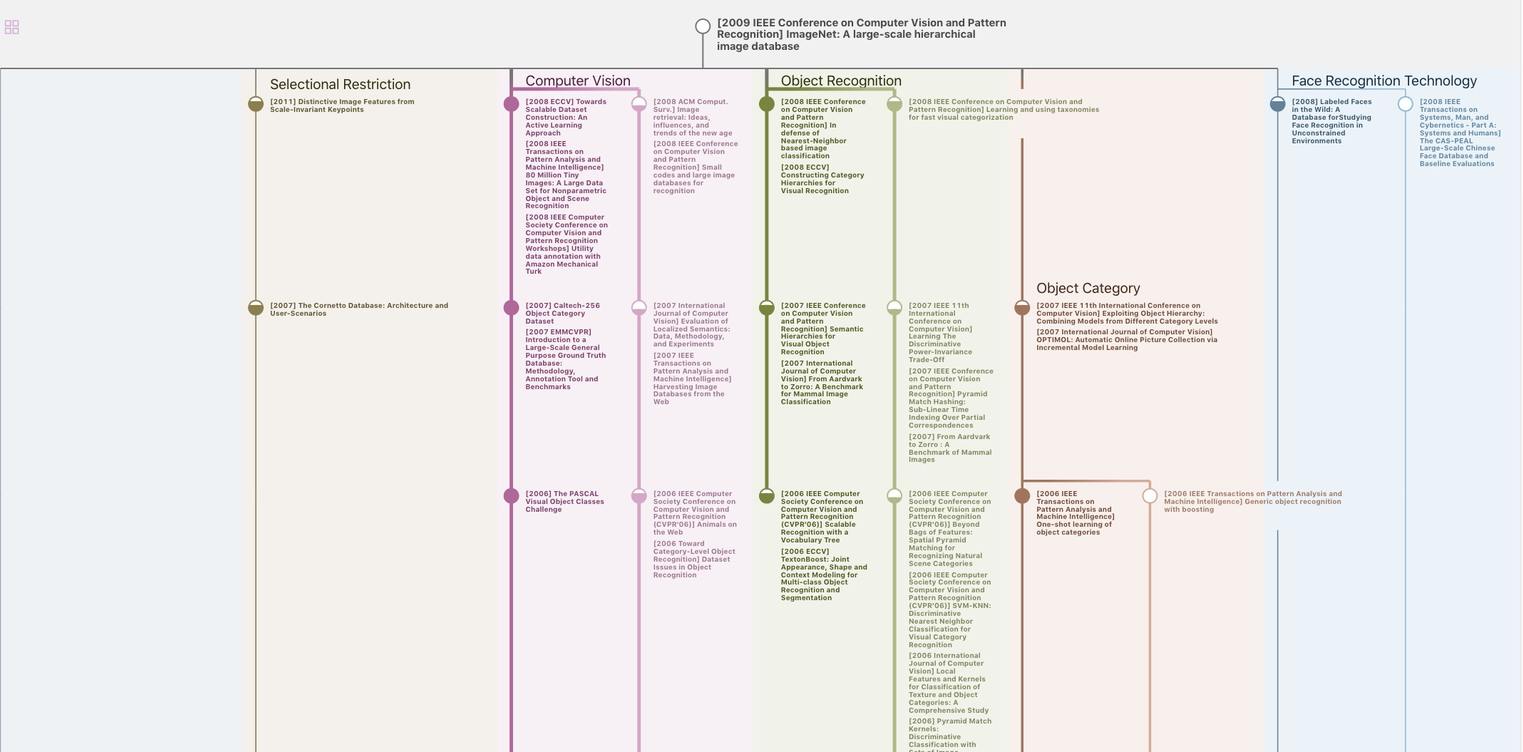
生成溯源树,研究论文发展脉络
Chat Paper
正在生成论文摘要