Joint Nonlocal, Spectral, and Similarity Low-Rank Priors for Hyperspectral-Multispectral Image Fusion
IEEE TRANSACTIONS ON GEOSCIENCE AND REMOTE SENSING(2022)
摘要
The fusion of a low-spatial-and-high-spectral resolution hyperspectral image (HSI) with a high-spatial-and-low-spectral resolution multispectral image (MSI) allows synthesizing a high-resolution image (HRI), supporting remote sensing applications, such as disaster management, material identification, and precision agriculture. Unlike existing variational methods using low-rank regularizations separately, we present an HSI-MSI fusion method promoting various low-rank regularizations jointly. Our method refines the HRI spatial and spectral correlations from the individual HSI and MSI data through the proper plug-and- play (PnP) of a nonlocal patch-based denoiser in the alternating direction method of multipliers (ADMM). Notably, we consider the nonlocal self-similarity, the spectral low-rank, and introduce a rank- one similarity prior. Furthermore, we demonstrate via an extensive empirical study that the rank-one similarity prior is an inherent characteristic of the HRI. Simulations over standard benchmark datasets show the effectiveness of the proposed HSI-MSI fusion outperforming state-of-the-art methods, particularly in recovering low-contrast areas.
更多查看译文
关键词
Hyperspectral image-multispectral image (HSI-MSI) fusion,low-rank regularizations,nonlocal patch-based denoiser,plug-and-play with alternating direction method of multipliers (PnP-ADMM)
AI 理解论文
溯源树
样例
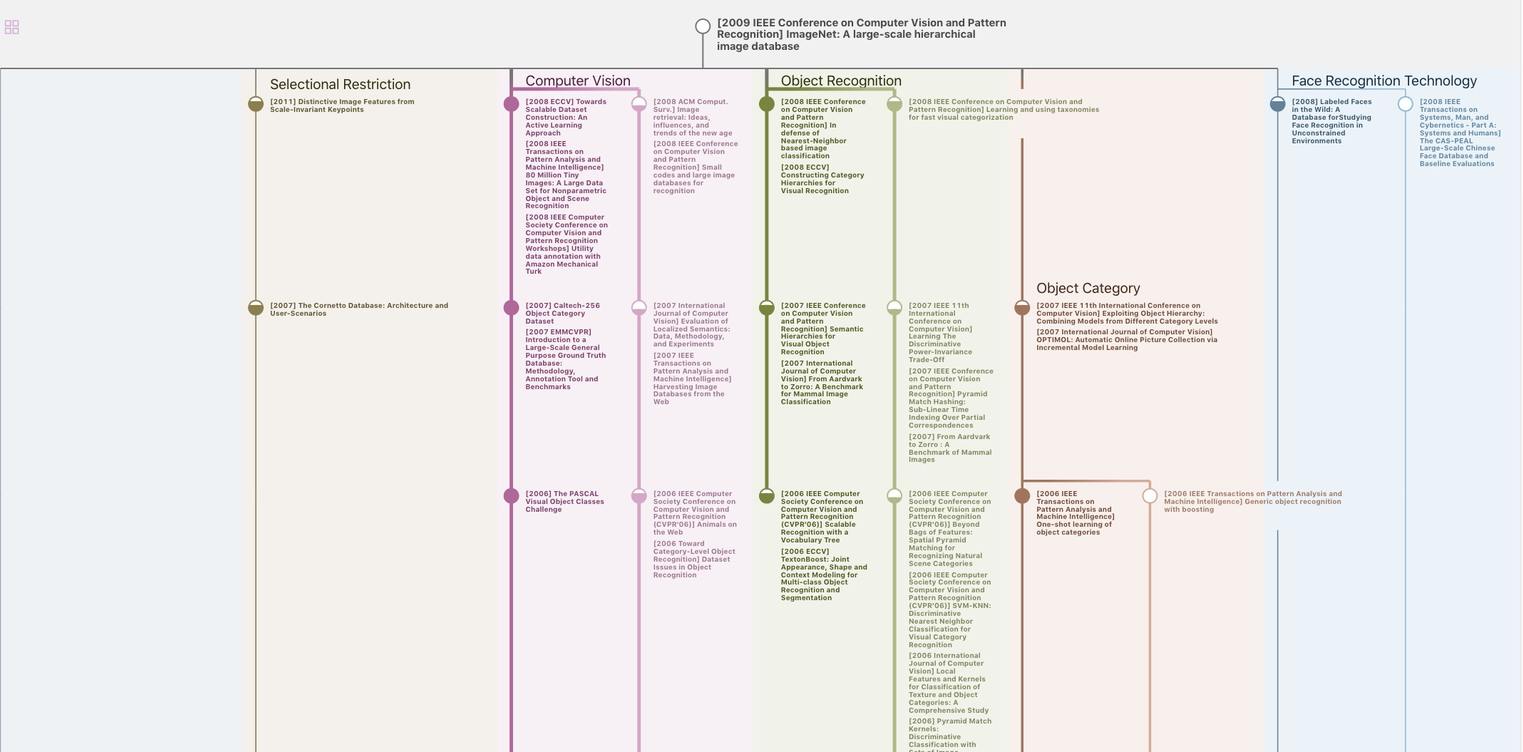
生成溯源树,研究论文发展脉络
Chat Paper
正在生成论文摘要