Coarse Retinal Lesion Annotations Refinement via Prototypical Learning
arxiv(2022)
摘要
Deep-learning-based approaches for retinal lesion segmentation often require an abundant amount of precise pixel-wise annotated data. However, coarse annotations such as circles or ellipses for outlining the lesion area can be six times more efficient than pixel-level annotation. Therefore, this paper proposes an annotation refinement network to convert a coarse annotation into a pixel-level segmentation mask. Our main novelty is the application of the prototype learning paradigm to enhance the generalization ability across different datasets or types of lesions. We also introduce a prototype weighing module to handle challenging cases where the lesion is overly small. The proposed method was trained on the publicly available IDRiD dataset and then generalized to the public DDR and our real-world private datasets. Experiments show that our approach substantially improved the initial coarse mask and outperformed the non-prototypical baseline by a large margin. Moreover, we demonstrate the usefulness of the prototype weighing module in both cross-dataset and cross-class settings.
更多查看译文
关键词
Prototypical learning,Retina lesion segmentation,Coarse annotation refinement
AI 理解论文
溯源树
样例
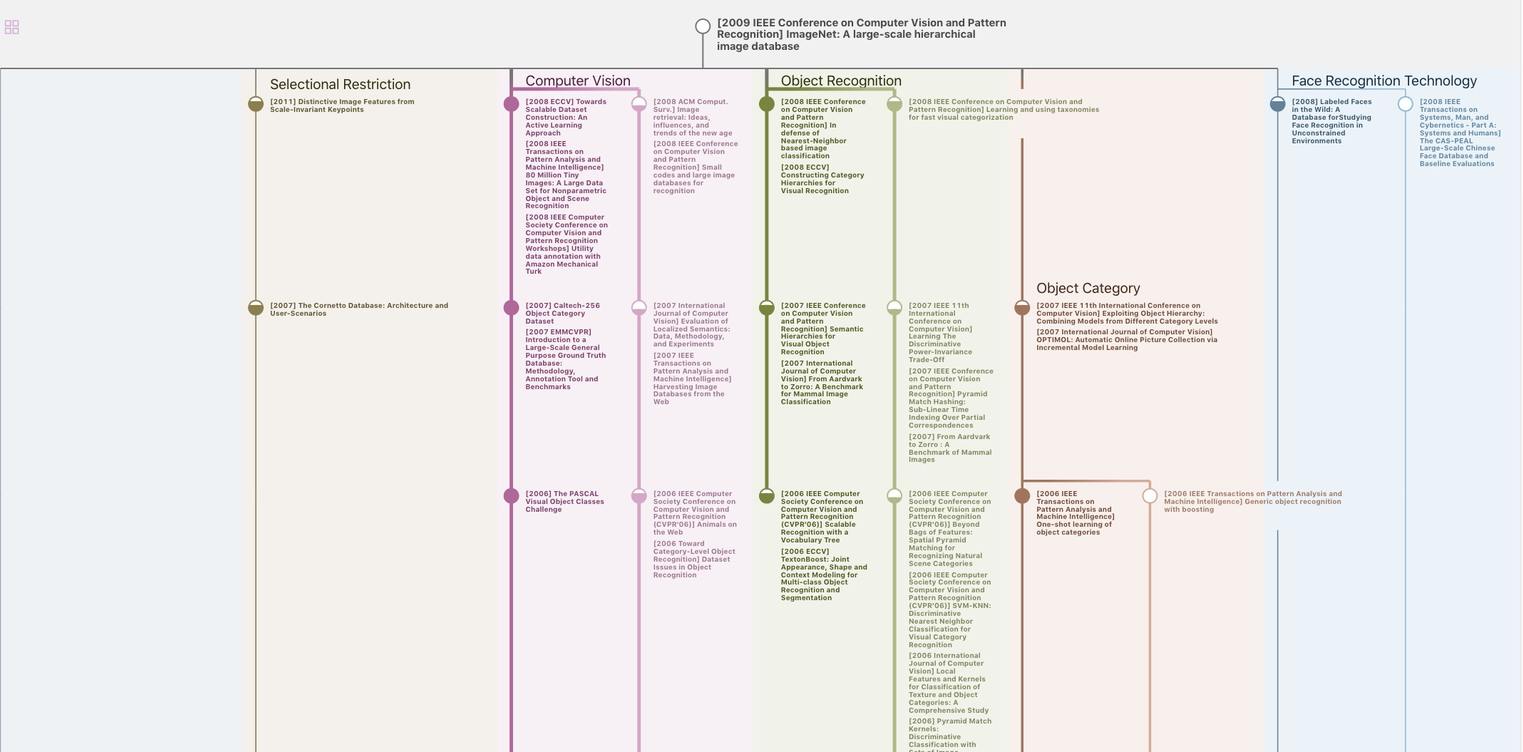
生成溯源树,研究论文发展脉络
Chat Paper
正在生成论文摘要