A Unified Primal-Dual Algorithm Framework for Inequality Constrained Problems
arxiv(2023)
摘要
In this paper, we propose a unified primal-dual algorithm framework based on the augmented Lagrangian function for composite convex problems with conic inequality constraints. The new framework is highly versatile. First, it not only covers many existing algorithms such as PDHG, Chambolle–Pock, GDA, OGDA and linearized ALM, but also guides us to design a new efficient algorithm called Semi-OGDA (SOGDA). Second, it enables us to study the role of the augmented penalty term in the convergence analysis. Interestingly, a properly selected penalty not only improves the numerical performance of the above methods, but also theoretically enables the convergence of algorithms like PDHG and SOGDA. Under properly designed step sizes and penalty term, our unified framework preserves the 𝒪(1/N) ergodic convergence while not requiring any prior knowledge about the magnitude of the optimal Lagrangian multiplier. Linear convergence rate for affine equality constrained problem is also obtained given appropriate conditions. Finally, numerical experiments on linear programming, ℓ _1 minimization problem, and multi-block basis pursuit problem demonstrate the efficiency of our methods.
更多查看译文
关键词
inequality constrained
AI 理解论文
溯源树
样例
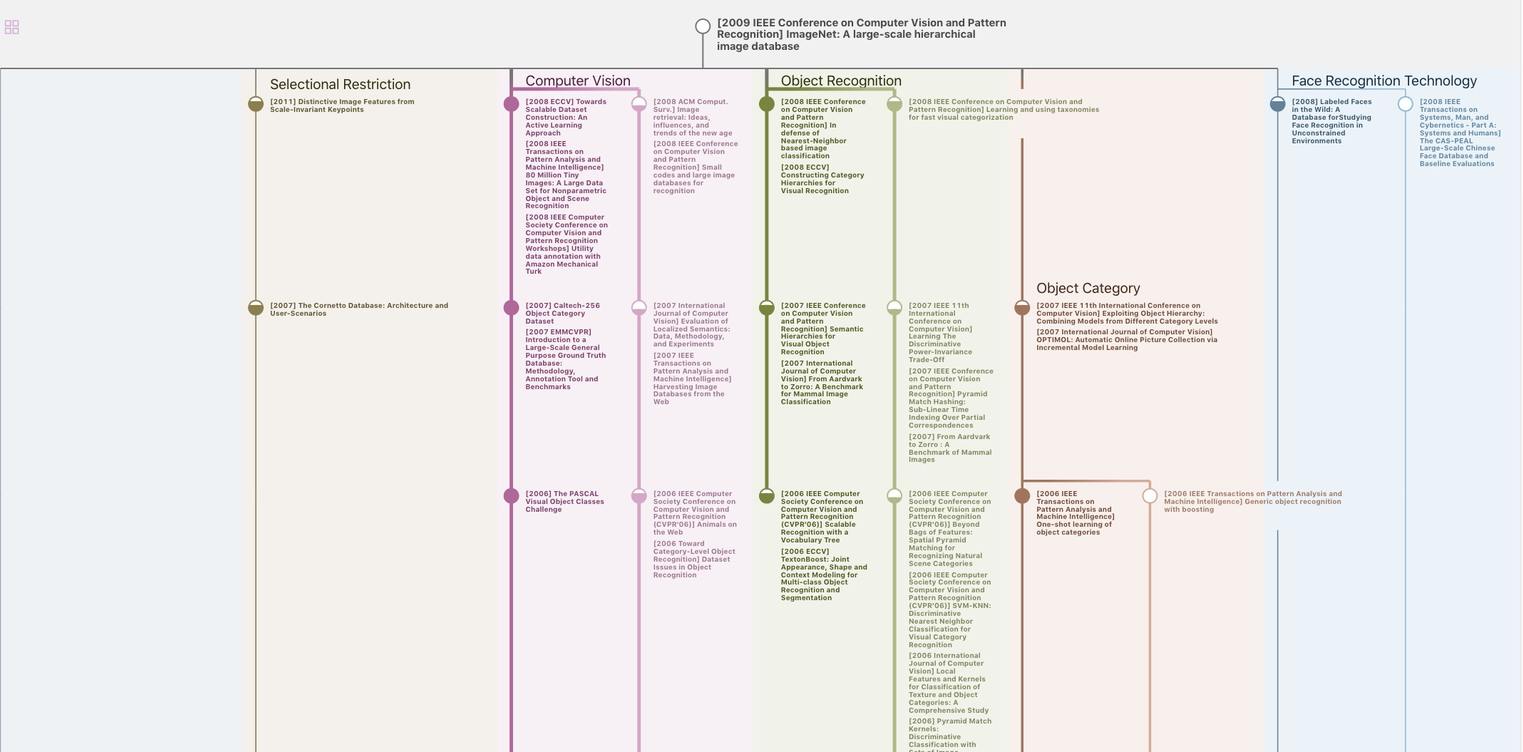
生成溯源树,研究论文发展脉络
Chat Paper
正在生成论文摘要