Multiresolution categorical regression for interpretable cell-type annotation
BIOMETRICS(2023)
摘要
In many categorical response regression applications, the response categories admit a multiresolution structure. That is, subsets of the response categories may naturally be combined into coarser response categories. In such applications, practitioners are often interested in estimating the resolution at which a predictor affects the response category probabilities. In this paper, we propose a method for fitting the multinomial logistic regression model in high dimensions that addresses this problem in a unified and data-driven way. Our method allows practitioners to identify which predictors distinguish between coarse categories but not fine categories, which predictors distinguish between fine categories, and which predictors are irrelevant. For model fitting, we propose a scalable algorithm that can be applied when the coarse categories are defined by either overlapping or nonoverlapping sets of fine categories. Statistical properties of our method reveal that it can take advantage of this multiresolution structure in a way existing estimators cannot. We use our method to model cell-type probabilities as a function of a cell's gene expression profile (i.e., cell-type annotation). Our fitted model provides novel biological insights which may be useful for future automated and manual cell-type annotation methodology.
更多查看译文
关键词
categorical response regression,cell-type annotation,convex optimization multinomial logistic regression,multiresolution learning,single-cell RNA-seq
AI 理解论文
溯源树
样例
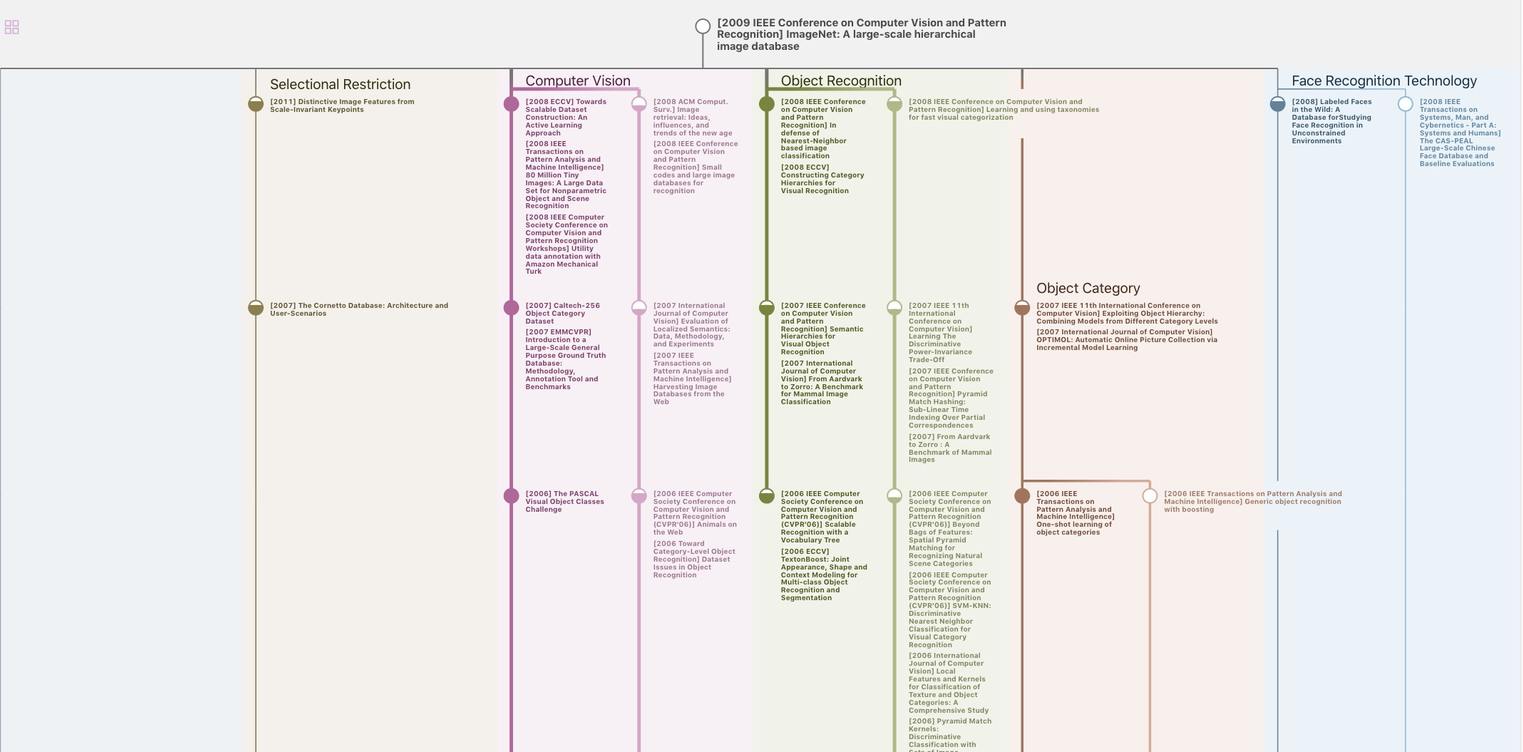
生成溯源树,研究论文发展脉络
Chat Paper
正在生成论文摘要