Supporting College Choice Among International Students through Collaborative Filtering
International journal of artificial intelligence in education(2022)
摘要
In this paper we use historic score-reporting records and test-taker metadata to inform data-driven recommendations that support international students in their choice of undergraduate institutions for study in the United States. We investigate the use of Structural Topic Modeling (STM) as a context-aware, probabilistic recommendation method that uses test-takers’ selections and metadata to model the latent space of college preferences. We present the model results from two perspectives: 1) to understand the impact of TOEFL score and test year on test-takers’ preferences and choices and 2) to recommend to the test-taker additional undergraduate institutions for application consideration. We find that TOEFL scores can explain variance in the probability that test-takers belong to certain preference-groups and, by accounting for this, our system adjusts recommendations based on student score. We also find that the inclusion of year, while not significantly altering recommendations, does enable us to capture minor changes in the relative popularity of similar institutions. The performance of this model demonstrates the utility of this approach for providing students with personalized college recommendations and offers a useful baseline approach that can be extended with additional data sources.
更多查看译文
关键词
Collaborative filtering,International education,Recommender systems,Structural topic modeling,Undergraduate education
AI 理解论文
溯源树
样例
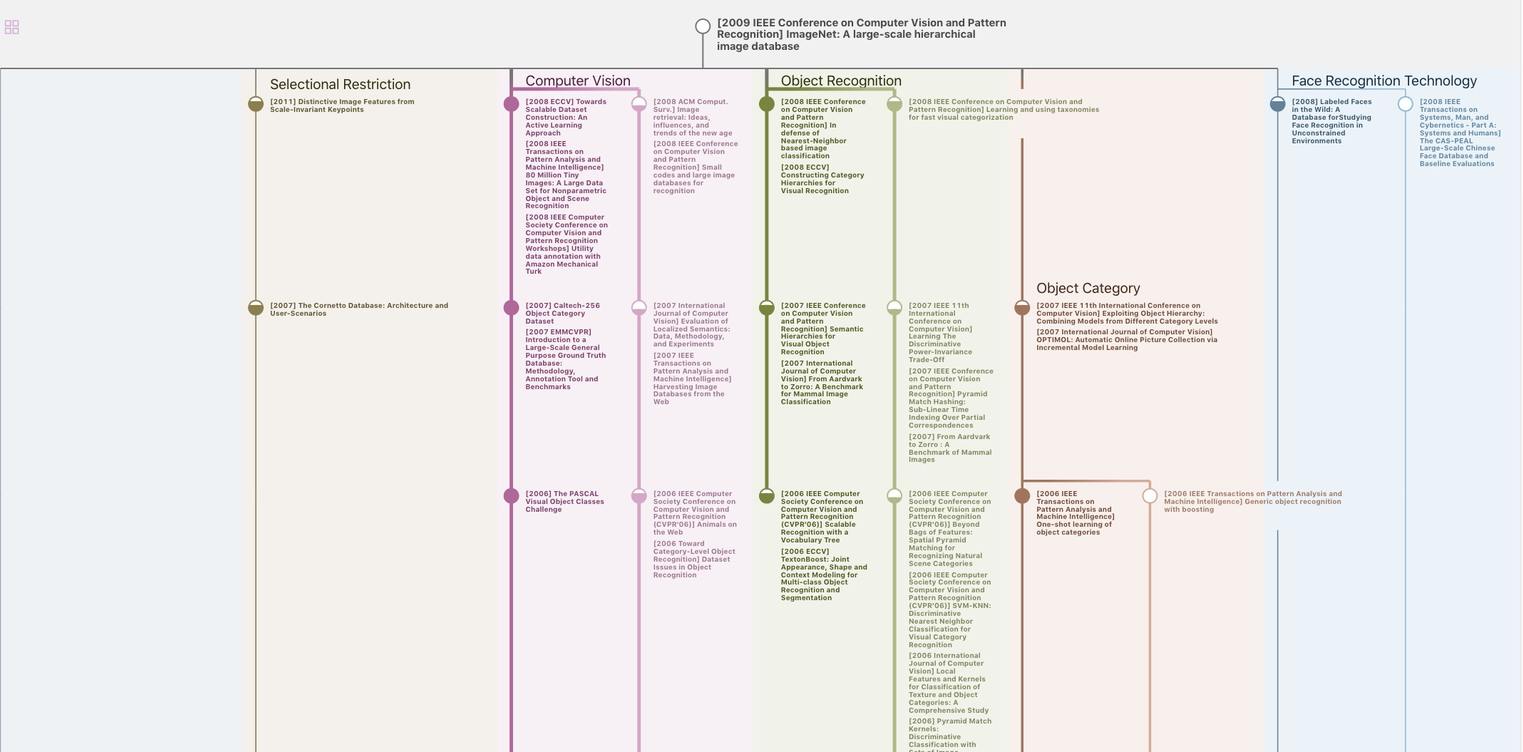
生成溯源树,研究论文发展脉络
Chat Paper
正在生成论文摘要