Prediction and motif analysis of 2'-O-methylation using a hybrid deep learning model from RNA primary sequence and nanopore signals
Current Bioinformatics(2022)
摘要
Background: 2’-O-Methylation (2’-O-Me) is a post-transcriptional RNA modification that occurs in the ribose sugar moiety of all four nucleotides and is abundant in both coding and non-coding RNAs. Accurate prediction of each subtype of 2’-O-Me (Am, Cm, Gm, Um) helps understand their role in RNA metabolism and function. Objective: To build models that can predict each subtype of 2’-O-Me from RNA sequence and nanopore signals and to exploit the model interpretability for sequence motif mining. Methods: We first propose a novel deep learning model DeepNm to better capture the sequence features of each subtype with a multi-scale framework. Based on DeepNm, we continue to propose HybridNm, which combines sequences and nanopore signals through a dual-path framework. The nanopore signal-derived features are first passed through a convolutional layer and then merged with sequence features extracted from different scales for final classification. Results: A 5-fold cross-validation process on Nm-seq data shows that DeepNm outperforms two state-of-the-art 2’-O-Me predictors. After incorporating nanopore signal-derived features, HybridNm further achieved significant improvements. Through model interpretation, we not only identified subtype-specific motifs, but also revealed shared motifs between subtypes. In addition, Cm, Gm, and Um shared motifs with the well-studied m6A RNA methylation, suggesting a potential interplay among different RNA modifications and the complex nature of epitranscriptome regulation. Conclusion: The proposed frameworks can be useful tools to predict 2’-O-Me subtypes accurately and reveal specific sequence patterns.
更多查看译文
关键词
2'-O-Methylation,site prediction,nanopore RNA sequencing,RNA methylation,epitranscriptome,Deep Learning
AI 理解论文
溯源树
样例
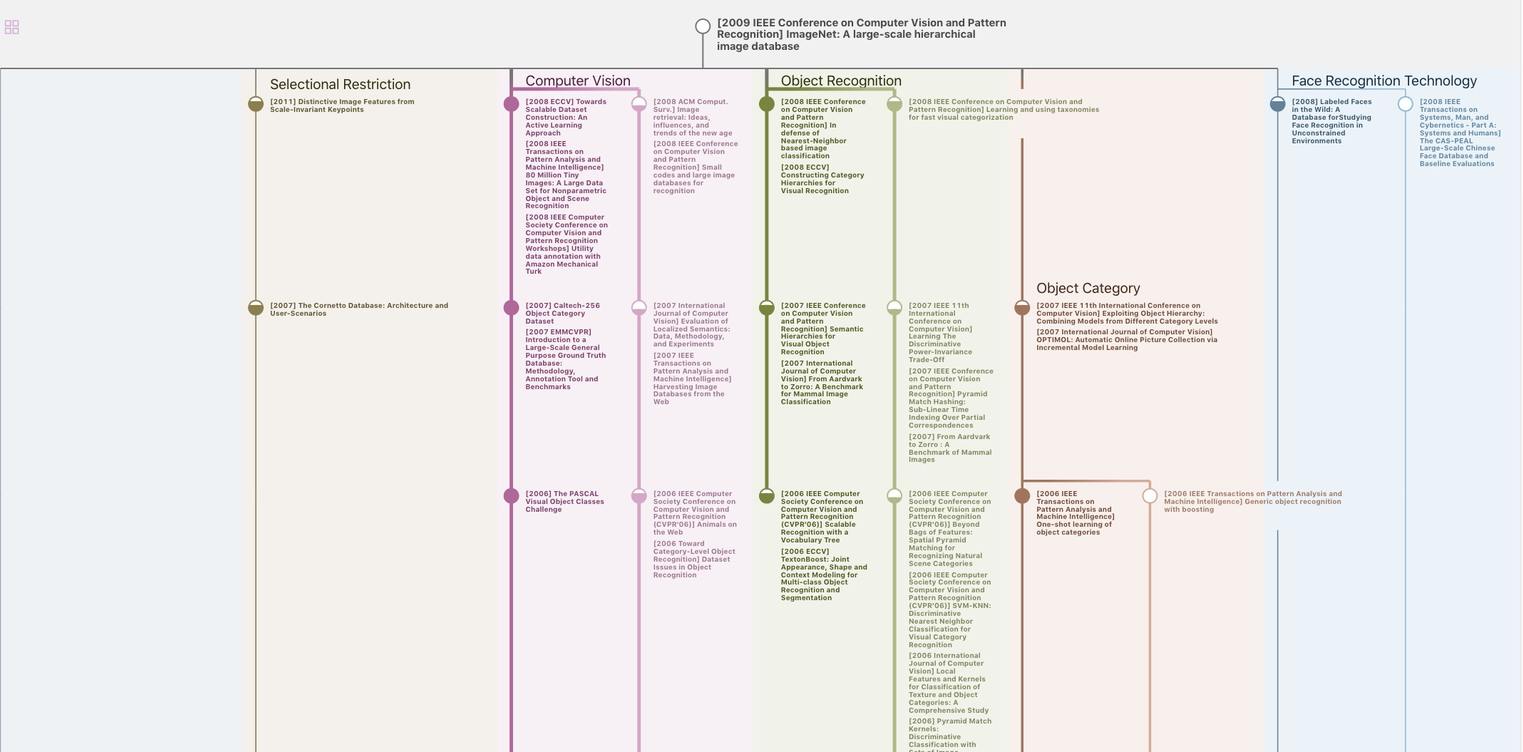
生成溯源树,研究论文发展脉络
Chat Paper
正在生成论文摘要