TBD3: Thresholding-Based Dynamic Depression Detection from Social Media for Low-Resource Users
user-61447a76e55422cecdaf7d19(2022)
摘要
Social media are heavily used by many users to share their mental health concerns and diagnoses. This trend has turned social media into a large-scale resource for researchers focused on detecting mental health conditions. Social media usage varies considerably across individuals. Thus, classification of patterns, including detecting signs of depression, must account for such variation. We address the disparity in classification effectiveness for users with little activity (e.g., new users). Our evaluation, performed on a large-scale dataset, shows considerable detection discrepancy based on user posting frequency. For instance, the F1 detection score of users with an above-median versus below-median number of posts is greater than double (0.803 vs 0.365) using a conventional CNN-based model; similar results were observed on lexical and transformer-based classifiers. To complement this evaluation, we propose a dynamic thresholding technique that adjusts the classifier's sensitivity as a function of the number of posts a user has. This technique alone reduces the margin between users with many and few posts, on average, by 45% across all methods and increases overall performance, on average, by 33%. These findings emphasize the importance of evaluating and tuning natural language systems for potentially vulnerable populations.
更多查看译文
关键词
depression detection, social media, thresholding, mental health, early risk detection
AI 理解论文
溯源树
样例
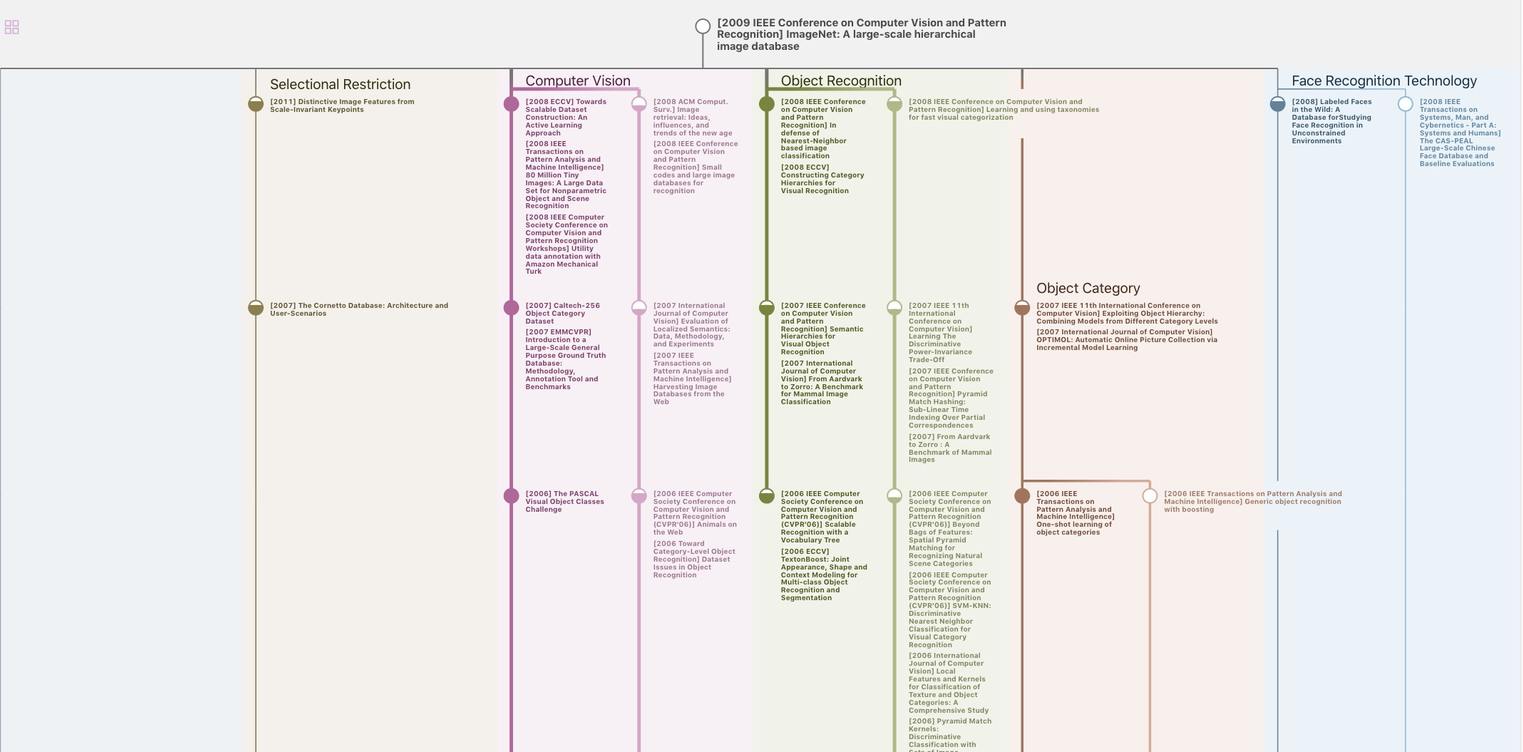
生成溯源树,研究论文发展脉络
Chat Paper
正在生成论文摘要