Weakly and Semi-Supervised Detection, Segmentation and Tracking of Table Grapes with Limited and Noisy Data
arxiv(2023)
摘要
Detection, segmentation and tracking of fruits and vegetables are three fundamental tasks for precision agriculture, enabling robotic harvesting and yield estimation applications. However, modern algorithms are data hungry and it is not always possible to gather enough data to apply the best performing supervised approaches. Since data collection is an expensive and cumbersome task, the enabling technologies for using computer vision in agriculture are often out of reach for small businesses. Following previous work in this context, where we proposed an initial weakly supervised solution to reduce the data needed to get state-of-the-art detection and segmentation in precision agriculture applications, here we improve that system and explore the problem of tracking fruits in orchards. We present the case of vineyards of table grapes in southern Lazio (Italy) since grapes are a difficult fruit to segment due to occlusion, color and general illumination conditions. We consider the case in which there is some initial labelled data that could work as source data (\eg wine grape data), but it is considerably different from the target data (e.g. table grape data). To improve detection and segmentation on the target data, we propose to train the segmentation algorithm with a weak bounding box label, while for tracking we leverage 3D Structure from Motion algorithms to generate new labels from already labelled samples. Finally, the two systems are combined in a full semi-supervised approach. Comparisons with state-of-the-art supervised solutions show how our methods are able to train new models that achieve high performances with few labelled images and with very simple labelling.
更多查看译文
关键词
grapes,segmentation,detection,tracking,noisy data,semi-supervised
AI 理解论文
溯源树
样例
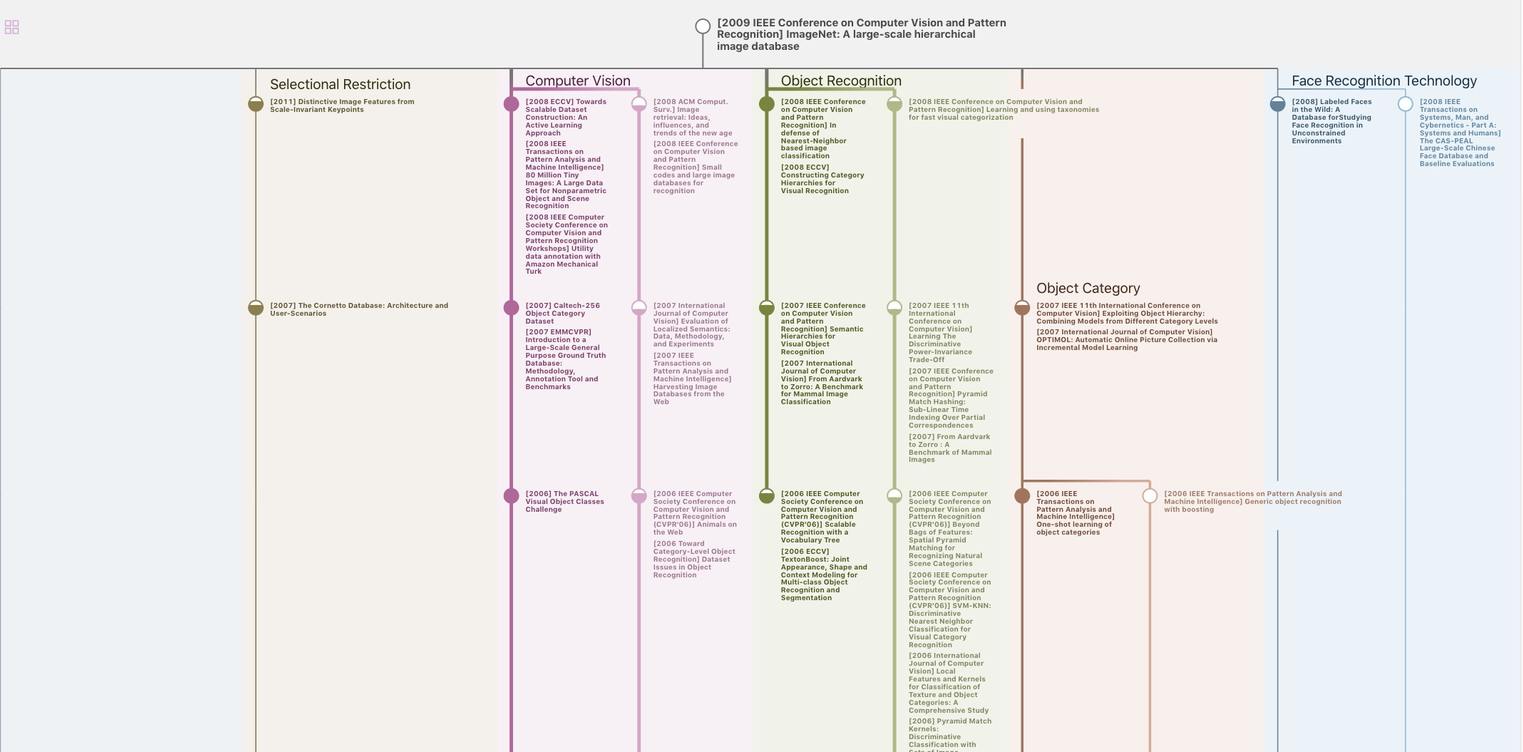
生成溯源树,研究论文发展脉络
Chat Paper
正在生成论文摘要