Randomised Composition and Small-Bias Minimax
2022 IEEE 63rd Annual Symposium on Foundations of Computer Science (FOCS)(2022)
摘要
We prove
1
two results about randomised query complexity $\mathbf{R}(f)$. First, we introduce a linearised complexity measure LR and show that it satisfies an inner-optimal composition theorem: $\mathbf{R}(f^{\circ} g)\geq\Omega(\mathbf{R}(f)\mathbf{L R}(g))$ for all partial f and g, and moreover, LR is the largest possible measure with this property. In particular, LR can be polynomially larger than previous measures that satisfy an inner composition theorem, such as the max-conflict complexity of Gavinsky, Lee, Santha, and Sanyal (ICALP 2019). Our second result addresses a question of Yao (FOCS 1977). He asked if $\epsilon$-error expected query complexity $\overline{\mathbf{R}}_{\epsilon}(f)$ admits a distributional characterisation relative to some hard input distribution. Vereshchagin (TCS 1998) answered this question affirmatively in the bounded-error case. We show that an analogous theorem fails in the small-bias case $\epsilon=1/2-o(1)$.
1
This is an extended abstract. For the full version of this article, please refer to [BDBGM22].
更多查看译文
AI 理解论文
溯源树
样例
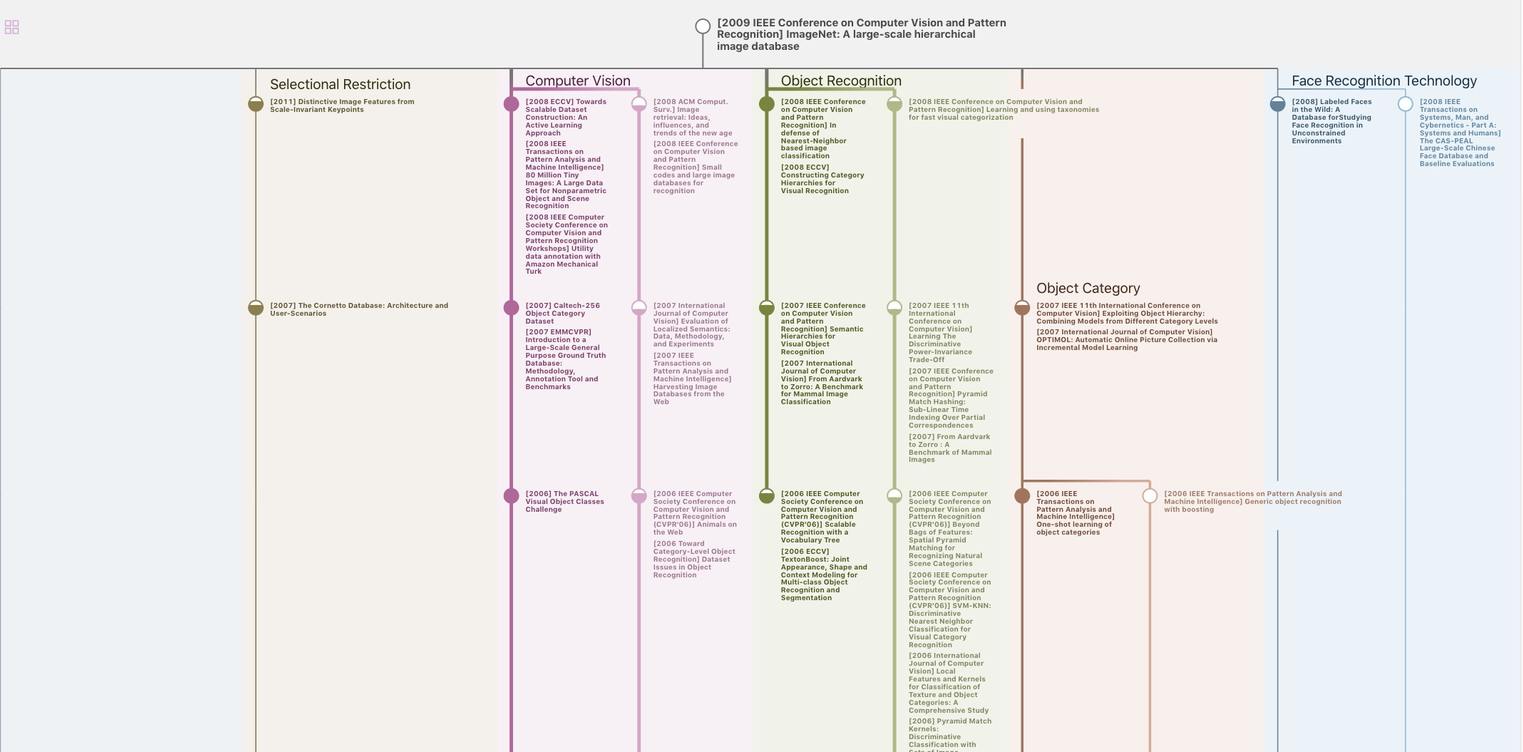
生成溯源树,研究论文发展脉络
Chat Paper
正在生成论文摘要