Using dorsal surface for individual identification of dairy calves through 3D deep learning algorithms
Computers and Electronics in Agriculture(2022)
摘要
Advances in machine learning techniques have allowed the development of computer vision systems (CVS) that can accurately predict several phenotypes of interest for livestock operations. In this context, 3D images taken from a top-down view are particularly useful for estimating body condition score, growth development, and body biometrics in cattle. Frequently, such CVS rely on identification (ID) systems, such as electronic tags, as a way to match animal ID and the predicted phenotype. However, the same 3D images used to predict body weight and other animal biometrics could be adopted for animal recognition as well. Such alternative would optimize CVS to recognize animal ID and monitor growth development simultaneously while leveraging the same hardware infrastructure. Furthermore, this strategy could be used to recognize animals with similar color patterns. Nonetheless, growing animals are continuously changing body shape, which could limit its use as an invariant feature for pattern recognition. Thus, the objectives of this study were: (1) to compare algorithms for different 3D object representations to identify individual animals; and (2) to evaluate how short-term changes in body shape due to animal growth affect the predictive performance of these algorithms. For objective 1, the algorithms were trained (n = 4,558) and tested (n = 1,139) using images from 38 Holstein calves. For objective 2, we designed three different experiments using images (n = 2,347) from five Holstein calves taken over six weeks during their growing period, always training and testing on different weeks. Each experiment evaluated how changing a different parameter of the image capturing procedure affected the predictive ability of the trained algorithms. In the first experiment, we varied the total number of images per animal in the training set; in the second experiment, we varied the number of weeks while keeping a fixed number of images in the training set; and in the third experiment, we skipped weeks between images in the training and test sets. The F1 score for objective (1) was up to 0.804 when testing with the last frames of each video, and up to 0.959 when using random frames for testing. For objective (2), the F1 score was up to 0.947 for the first experiment when using 130 images per animal; up to 0.979 for the second experiment when using all five weeks; and up to 0.917 when not skipping weeks between training and testing. These results show that deep learning algorithms can be used to identify individual animals through their dorsal area 3D surfaces, and, from our experiments using calves in their growing period, that they are robust enough to account for changes in body shape and size, making them a promising tool for animal recognition during growth.
更多查看译文
关键词
Growth,Calves,Animal traceability,Deep learning,Animal identification,3D neural networks
AI 理解论文
溯源树
样例
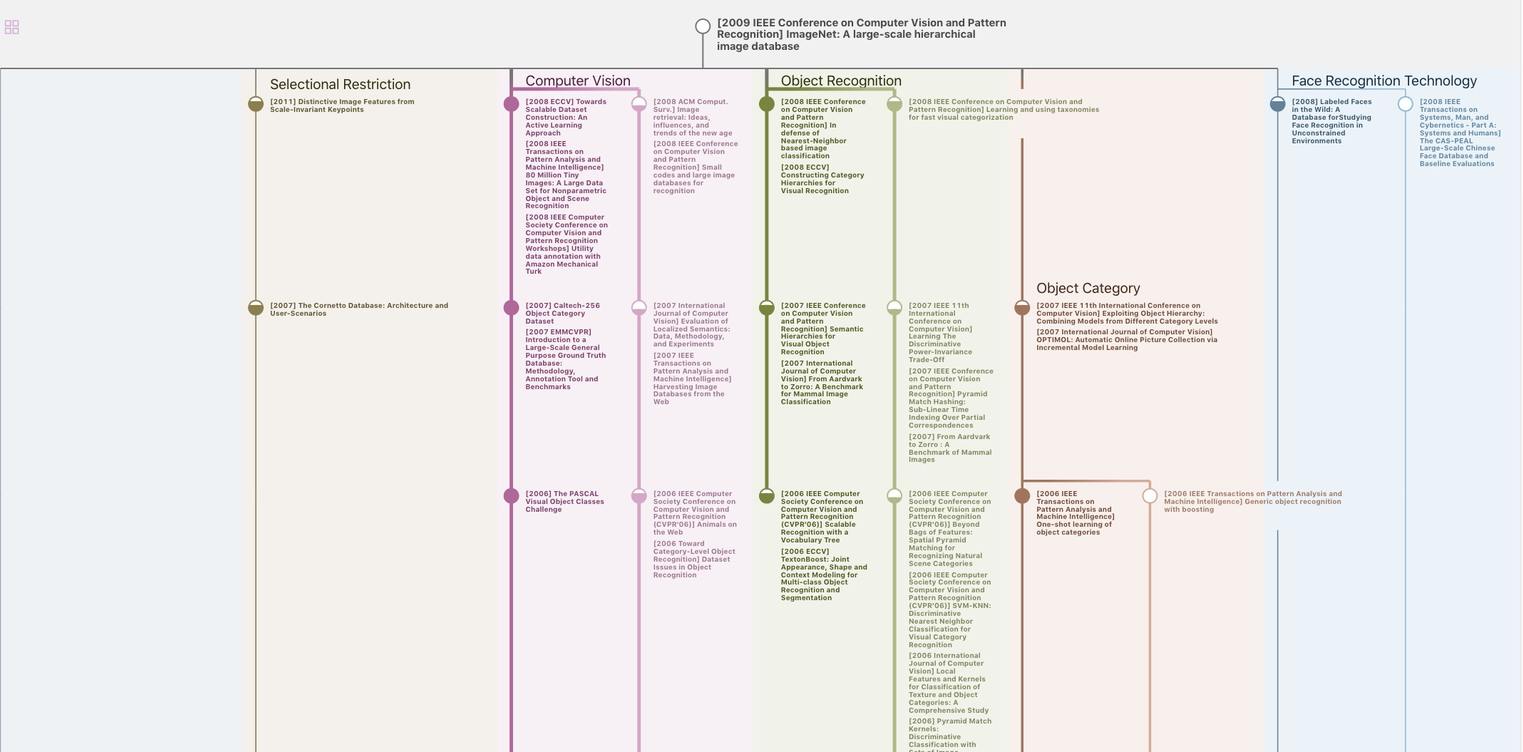
生成溯源树,研究论文发展脉络
Chat Paper
正在生成论文摘要