LUCID: Exposing Algorithmic Bias through Inverse Design
arxiv(2022)
摘要
AI systems can create, propagate, support, and automate bias in decision-making processes. To mitigate biased decisions, we both need to understand the origin of the bias and define what it means for an algorithm to make fair decisions. Most group fairness notions assess a model's equality of outcome by computing statistical metrics on the outputs. We argue that these output metrics encounter intrinsic obstacles and present a complementary approach that aligns with the increasing focus on equality of treatment. By Locating Unfairness through Canonical Inverse Design (LUCID), we generate a canonical set that shows the desired inputs for a model given a preferred output. The canonical set reveals the model's internal logic and exposes potential unethical biases by repeatedly interrogating the decision-making process. We evaluate LUCID on the UCI Adult and COMPAS data sets and find that some biases detected by a canonical set differ from those of output metrics. The results show that by shifting the focus towards equality of treatment and looking into the algorithm's internal workings, the canonical sets are a valuable addition to the toolbox of algorithmic fairness evaluation.
更多查看译文
关键词
inverse design,algorithmic bias
AI 理解论文
溯源树
样例
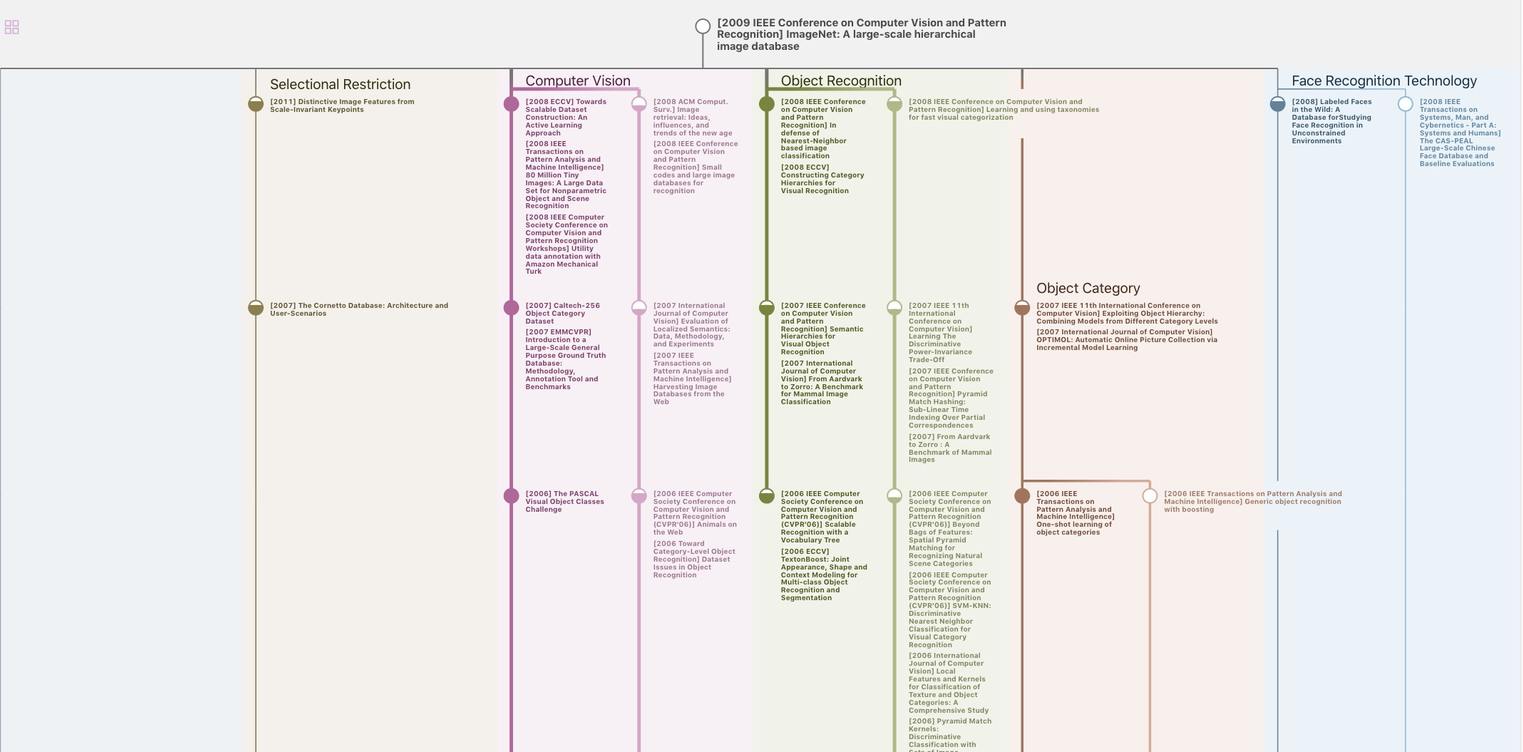
生成溯源树,研究论文发展脉络
Chat Paper
正在生成论文摘要