Symbolic Explanation of Affinity-Based Reinforcement Learning Agents with Markov Models
arxiv(2022)
摘要
The proliferation of artificial intelligence is increasingly dependent on model understanding. Understanding demands both an interpretation - a human reasoning about a model's behavior - and an explanation - a symbolic representation of the functioning of the model. Notwithstanding the imperative of transparency for safety, trust, and acceptance, the opacity of state-of-the-art reinforcement learning algorithms conceals the rudiments of their learned strategies. We have developed a policy regularization method that asserts the global intrinsic affinities of learned strategies. These affinities provide a means of reasoning about a policy's behavior, thus making it inherently interpretable. We have demonstrated our method in personalized prosperity management where individuals' spending behavior in time dictate their investment strategies, i.e. distinct spending personalities may have dissimilar associations with different investment classes. We now explain our model by reproducing the underlying prototypical policies with discretized Markov models. These global surrogates are symbolic representations of the prototypical policies.
更多查看译文
关键词
reinforcement,markov models,learning,affinity-based
AI 理解论文
溯源树
样例
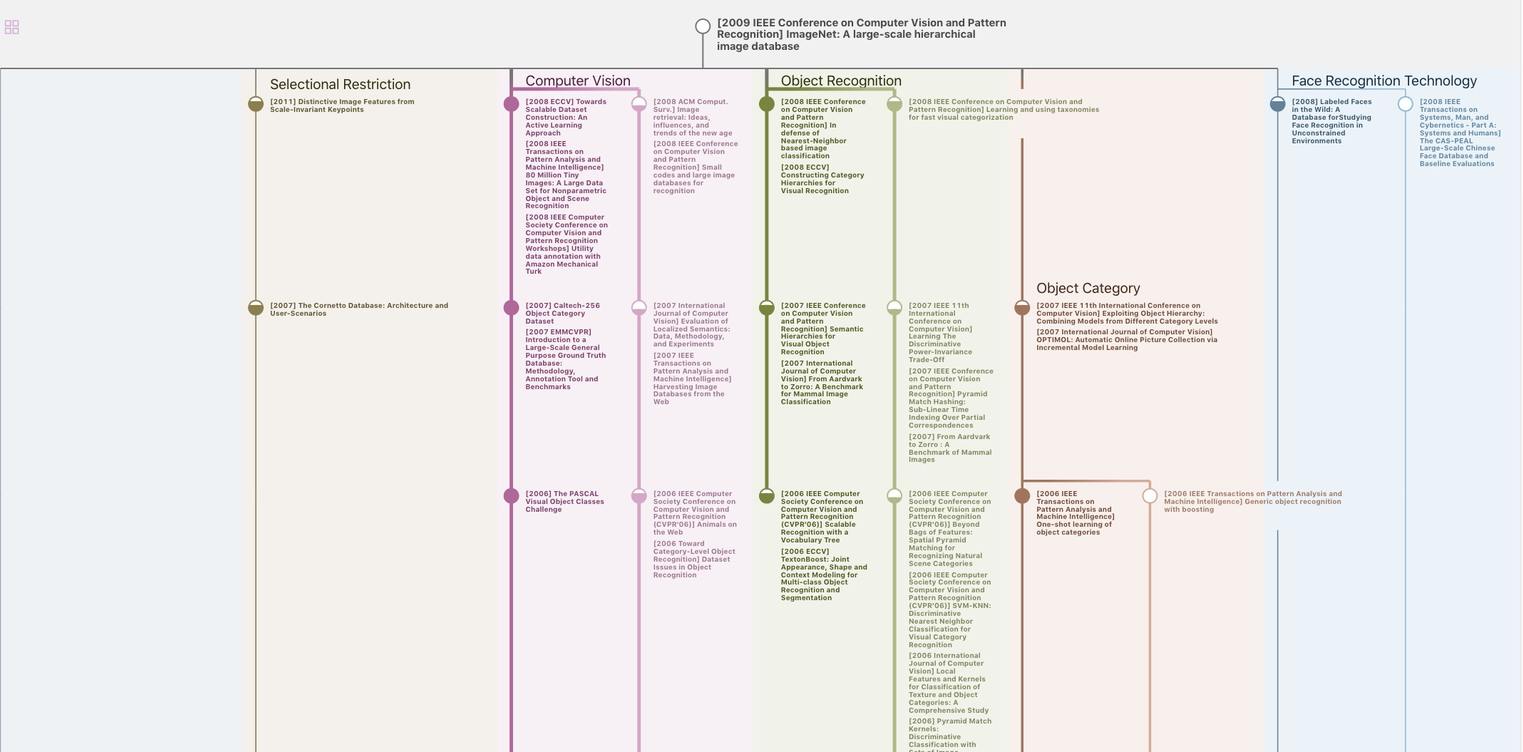
生成溯源树,研究论文发展脉络
Chat Paper
正在生成论文摘要