Toward Robust Graph Semi-Supervised Learning Against Extreme Data Scarcity.
arxiv(2024)
摘要
The success of graph neural networks (GNNs) in graph-based web mining highly relies on abundant human-annotated data, which is laborious to obtain in practice. When only a few labeled nodes are available, how to improve their robustness is key to achieving replicable and sustainable graph semi-supervised learning. Though self-training is powerful for semi-supervised learning, its application on graph-structured data may fail because 1) larger receptive fields are not leveraged to capture long-range node interactions, which exacerbates the difficulty of propagating feature-label patterns from labeled nodes to unlabeled nodes and 2) limited labeled data makes it challenging to learn well-separated decision boundaries for different node classes without explicitly capturing the underlying semantic structure. To address the challenges of capturing informative structural and semantic knowledge, we propose a new graph data augmentation framework, augmented graph self-training (AGST), which is built with two new (i.e., structural and semantic) augmentation modules on top of a decoupled GST backbone. In this work, we investigate whether this novel framework can learn a robust graph predictive model under the low-data context. We conduct comprehensive evaluations on semi-supervised node classification under different scenarios of limited labeled-node data. The experimental results demonstrate the unique contributions of the novel data augmentation framework for node classification with few labeled data.
更多查看译文
关键词
Data scarcity,graph neural networks (GNNs),robustness,self-training
AI 理解论文
溯源树
样例
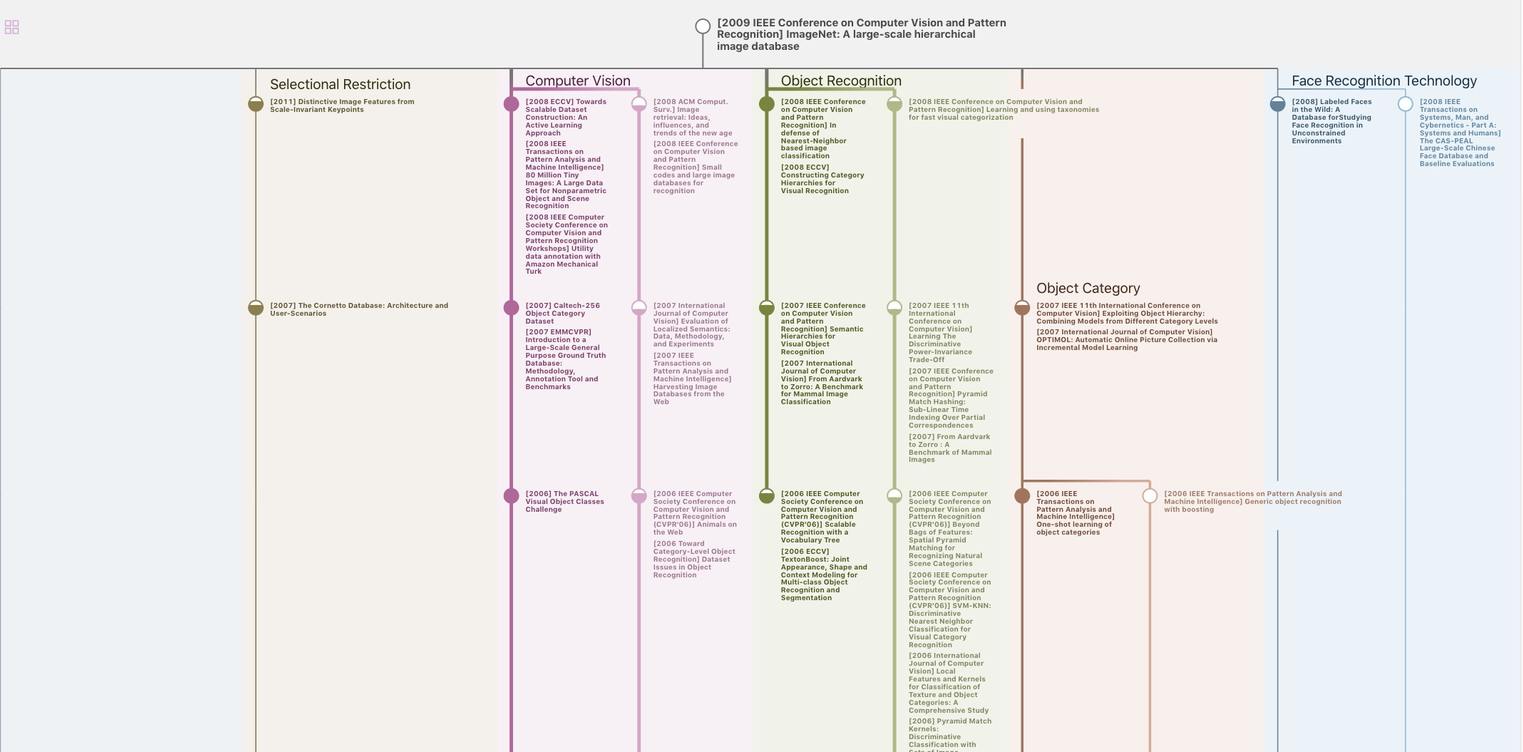
生成溯源树,研究论文发展脉络
Chat Paper
正在生成论文摘要