Social Diversity for ATL Repair
arxiv(2022)
摘要
Model transformations play an essential role in the Model-Driven Engineering paradigm. Writing a correct transformation program requires to be proficient with the source and target modeling languages, to have a clear understanding of the mapping between the elements of the two, as well as to master the transformation language to properly describe the transformation. Transformation programs are thus complex and error-prone, and finding and fixing errors in such programs typically involve a tedious and time-consuming effort by developers. In this paper, we propose a novel search-based approach to automatically repair transformation programs containing many semantic errors. To prevent the fitness plateaus and the single fitness peak limitations, we leverage the notion of social diversity to promote repair patches tackling errors that are less covered by the other patches of the population. We evaluate our approach on 71 semantically incorrect transformation programs written in ATL, and containing up to five semantic errors simultaneously. The evaluation shows that integrating social diversity when searching for repair patches allows to improve the quality of those patches and to speed up the convergence even when up to five semantic errors are involved.
更多查看译文
关键词
atl repair,social,diversity
AI 理解论文
溯源树
样例
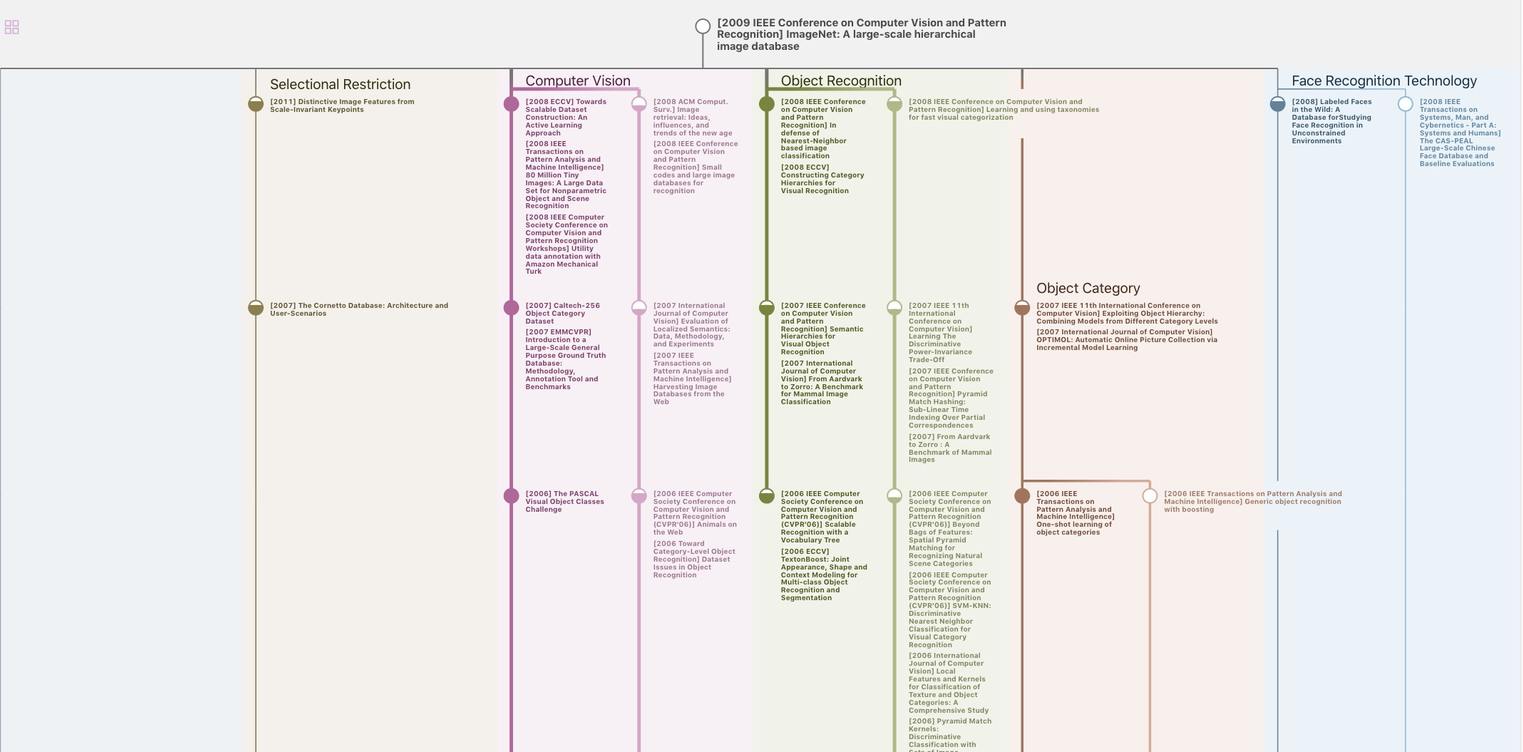
生成溯源树,研究论文发展脉络
Chat Paper
正在生成论文摘要