OOD-Probe: A Neural Interpretation of Out-of-Domain Generalization
arxiv(2022)
摘要
The ability to generalize out-of-domain (OOD) is an important goal for deep neural network development, and researchers have proposed many high-performing OOD generalization methods from various foundations. While many OOD algorithms perform well in various scenarios, these systems are evaluated as ``black-boxes''. Instead, we propose a flexible framework that evaluates OOD systems with finer granularity using a probing module that predicts the originating domain from intermediate representations. We find that representations always encode some information about the domain. While the layerwise encoding patterns remain largely stable across different OOD algorithms, they vary across the datasets. For example, the information about rotation (on RotatedMNIST) is the most visible on the lower layers, while the information about style (on VLCS and PACS) is the most visible on the middle layers. In addition, the high probing results correlate to the domain generalization performances, leading to further directions in developing OOD generalization systems.
更多查看译文
关键词
neural interpretation,ood-probe,out-of-domain
AI 理解论文
溯源树
样例
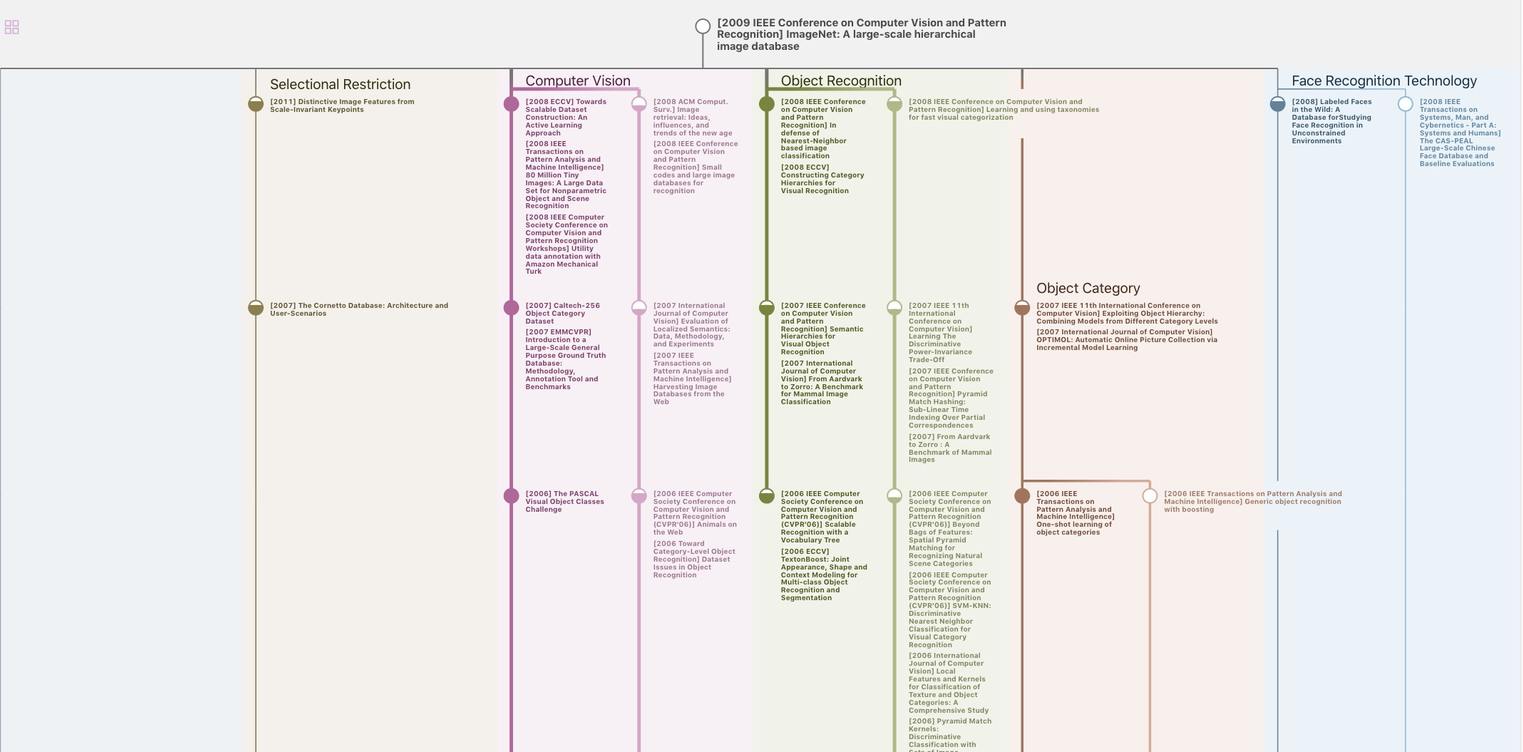
生成溯源树,研究论文发展脉络
Chat Paper
正在生成论文摘要