k-GCN-LSTM: A k-hop Graph Convolutional Network and Long Short-Term Memory for ship speed prediction
Physica A: Statistical Mechanics and its Applications(2022)
摘要
Ship speed information plays an important role in the maritime intelligent transportation system, and ship speed prediction has attracted extensive attention in maritime community. Previous studies suggest that ship speed is mainly affected by her previous movement status, regardless the interferences from neighboring locations. To address the issue, a novel deep learning framework is proposed to predict ship speed via the support of k-hop graph convolutional network (k-GCN) and long-short term memory (LSTM). Firstly, a ship network model is established with a graph network. The nodes in the proposed networks demonstrate ship trajectory segments, whilst the segments are connected to obtain edges. Secondly, the GCN is used to capture the spatial correlation between the nodes, and the LSTM is used to exploit spatial-temporal correlation among the nodes. Finally, the proposed k-GCN-LSTM model is used to predict ship speed, which are further verified on different real-world ship trajectory data against with other models. Results suggest that the proposed model obtains satisfied prediction performance in terms of typical error measurement indicators. The research findings help maritime traffic participants better avoid potential collisions and enhance maritime traffic safety. (C) 2022 Elsevier B.V. All rights reserved.
更多查看译文
关键词
Maritime intelligent traffic system,Ship speed prediction,Spatiotemporal correlation exploitation,GCN,LSTM,AIS data
AI 理解论文
溯源树
样例
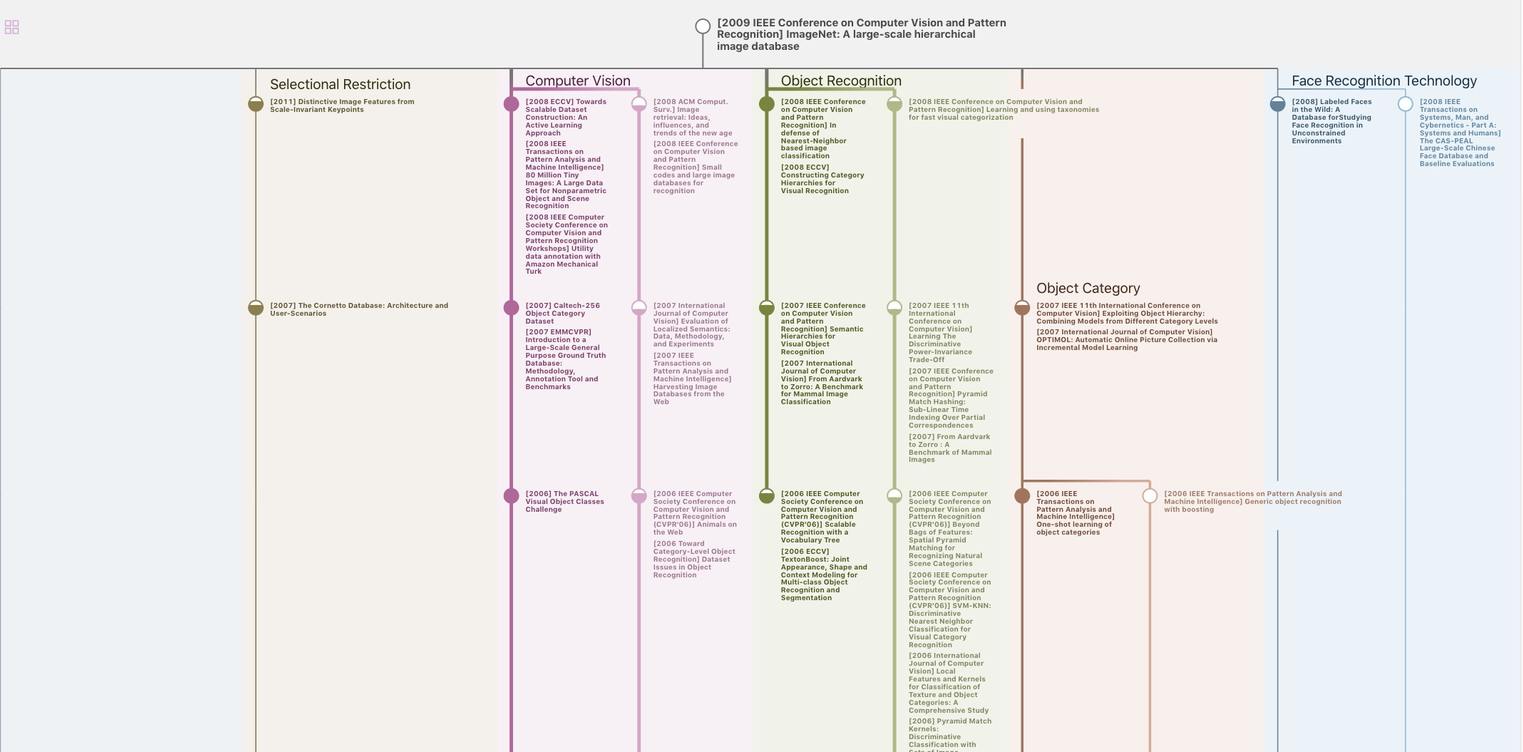
生成溯源树,研究论文发展脉络
Chat Paper
正在生成论文摘要