Multi-task learning neural networks for breath sound detection and classification in pervasive healthcare
Pervasive and Mobile Computing(2022)
摘要
With the emergence of many grave Chronic obstructive pulmonary diseases (COPDs) and the COVID-19 pandemic, there is a need for timely detection of abnormal respiratory sounds, such as deep and heavy breaths. Although numerous efficient pervasive healthcare systems have been proposed for tracking patients, few studies have focused on these breaths. This paper presents a method that supports physicians in monitoring in-hospital and at-home patients by monitoring their breath. The proposed method is based on three deep neural networks in audio analysis: RNNoise for noise suppression, SincNet - Convolutional Neural Network, and Residual Bidirectional Long Short-Term Memory for breath sound analysis at edge devices and centralized servers, respectively. We also developed a pervasive system with two configurations: (i) an edge architecture for in-hospital patients; and (ii) a central architecture for at-home ones. Furthermore, a dataset, named BreathSet, was collected from 27 COPD patients being treated at three hospitals in Vietnam to verify our proposed method. The experimental results demonstrated that our system efficiently detected and classified breath sounds with F1-scores of 90% and 91% for the tiny model version on low-cost edge devices, and 90% and 95% for the full model version on central servers, respectively. The proposed system was successfully implemented at hospitals to help physicians in monitoring respiratory patients in real time11Data and code: https://github.com/PTITLab/Multitask-Breath-Sound..
更多查看译文
关键词
Pervasive deep learning,SincNet-CNN,Residual BiLSTM,RNNoise,Breath sound detection and classification,BreathSet dataset
AI 理解论文
溯源树
样例
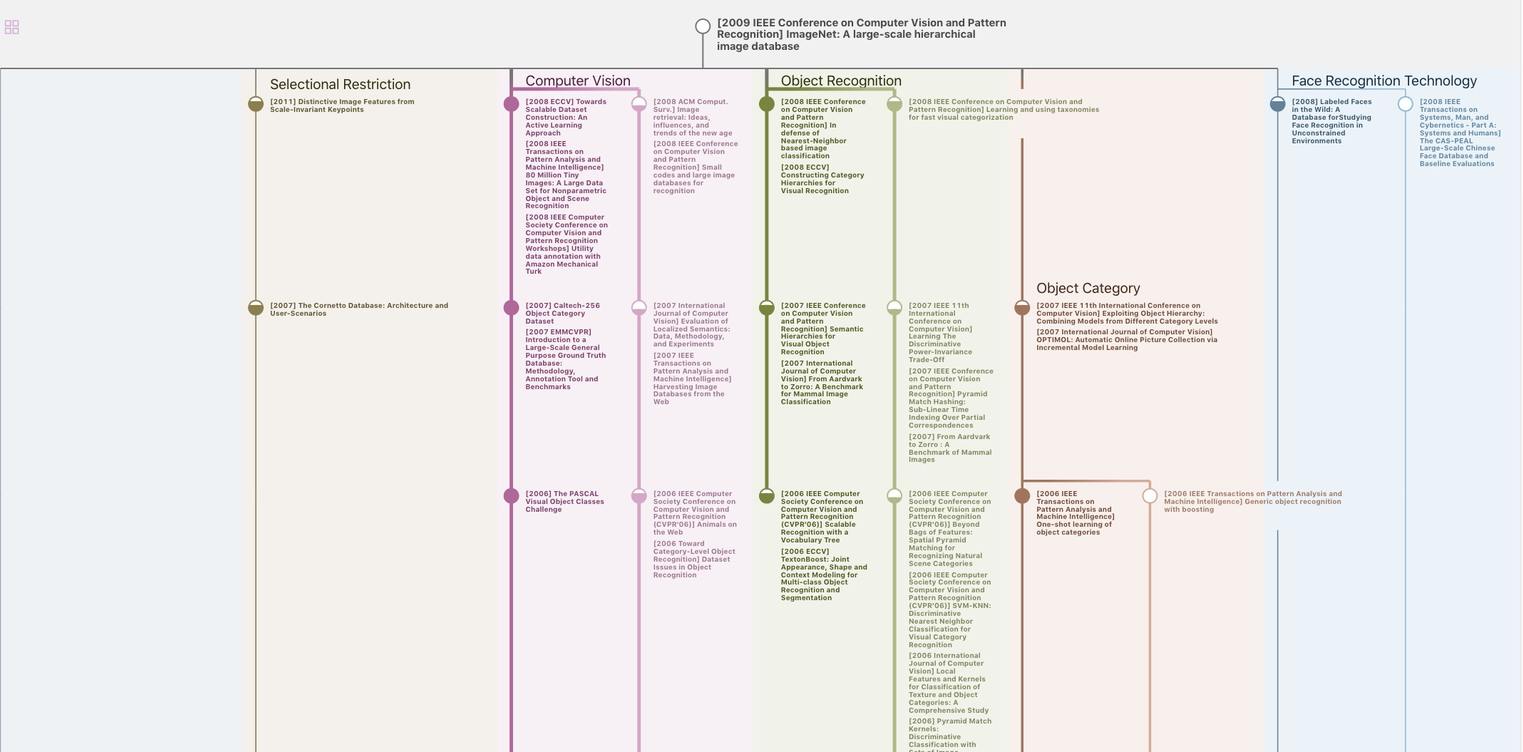
生成溯源树,研究论文发展脉络
Chat Paper
正在生成论文摘要