Multiple Diseases and Pests Detection Based on Federated Learning and Improved Faster R-CNN
IEEE TRANSACTIONS ON INSTRUMENTATION AND MEASUREMENT(2022)
摘要
Traditional disease and pest detection technology employ cloud-based deep learning, which facing the pressures, such as high data storage and communication costs, unbalanced and insufficient data from orchards, diversity of pests and diseases, and complex detection environments. In this article, we propose a multiple pest detection technique based on federated learning (FL) and improved faster region convolutional neural network (R-CNN). As the new distributed computing model, FL can derive a shared model integrating the advantages of data from all parties without uploading local data and also reduces the communication cost. A restriction M is added to the FL algorithm to ensure the convergence of the model and improve the training speed. According to the original faster R-CNN network, ResNet-101 is used instead of VGG-16 to construct the base convolutional layer to maintain the original structure of small-sized targets and improve the detection speed. Then, the multisize fusion of feature maps from different convolutional layers is performed to improve the detection accuracy of multisize multiple pests and diseases. Finally, a soft-nonmaximum suppression (NMS) algorithm is proposed to solve the apple obscured problem after the region proposal network (RPN). The experimental results show that the improved faster R-CNN can achieve an average accuracy of 90.27% on multiple pest detection, and the detection time is only 0.05 s per image. After using FL, the mean average precision (mAP) of the model reached 89.34% and the model training speed was improved by 59%.
更多查看译文
关键词
Disease and pest detection,faster region convolutional neural network (R-CNN),federated learning (FL),multiscale integration,object detection
AI 理解论文
溯源树
样例
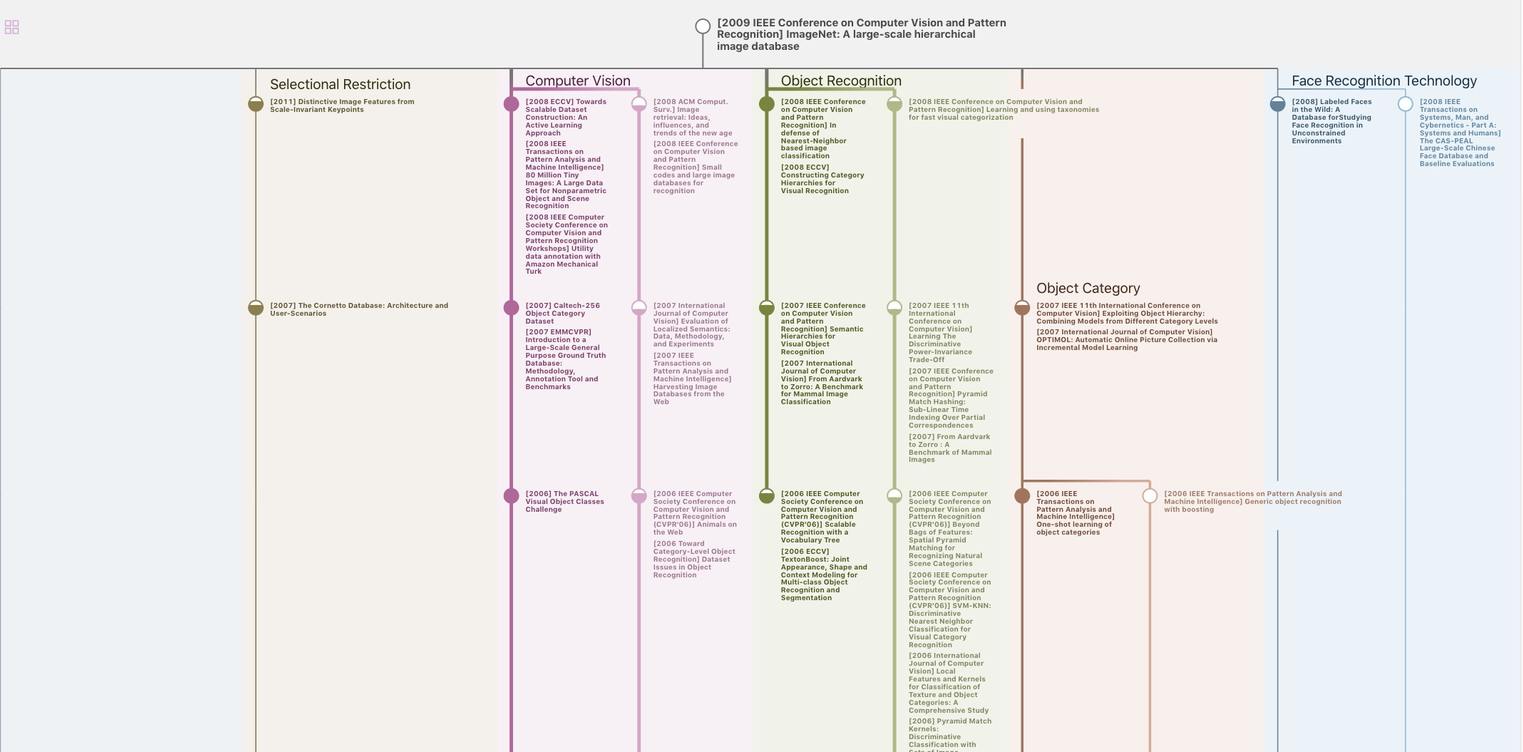
生成溯源树,研究论文发展脉络
Chat Paper
正在生成论文摘要