Robust Sparse Weighted Classification for Crowdsourcing.
IEEE Transactions on Knowledge and Data Engineering(2023)
摘要
Data collected from nature is usually unlabeled, and it is difficult to be used directly. This issue is well addressed by crowdsourcing, which provides a reasonable way for effectively using these unlabeled data. Generally, workers in crowdsourcing tasks are not professionals, so it is hard to obtain high-quality labels. To address this issue, a robust sparse weighted classification algorithm is proposed, which try to adjust the samples that are not correctly classified in the original lables as much as possible. Specifically, we evalute the ability of different workers(indicator weight matrix) to accurately label different samples by fitting the real data matrix to its weighted reconstruction matrix. And then, l(2,1)-norm and worker labeling ability similarity matrix are added, and negative effects of some bad workers are eliminated through the row sparsity property of l(2,1)-norm. Finally, the optimal indicator weight matrix is obtained by optimizing the two matrices in the objective function simultaneously. Therefore, the obtained optimal indicator weight matrix takes the similarity of worker labeling ability into consideration, and infers all the predicted labels. The results on synthetic and real data sets demonstrate that our algorithm is superior to other state-of-the-art methods.
更多查看译文
关键词
Crowdsourcing, classification, sparse learning
AI 理解论文
溯源树
样例
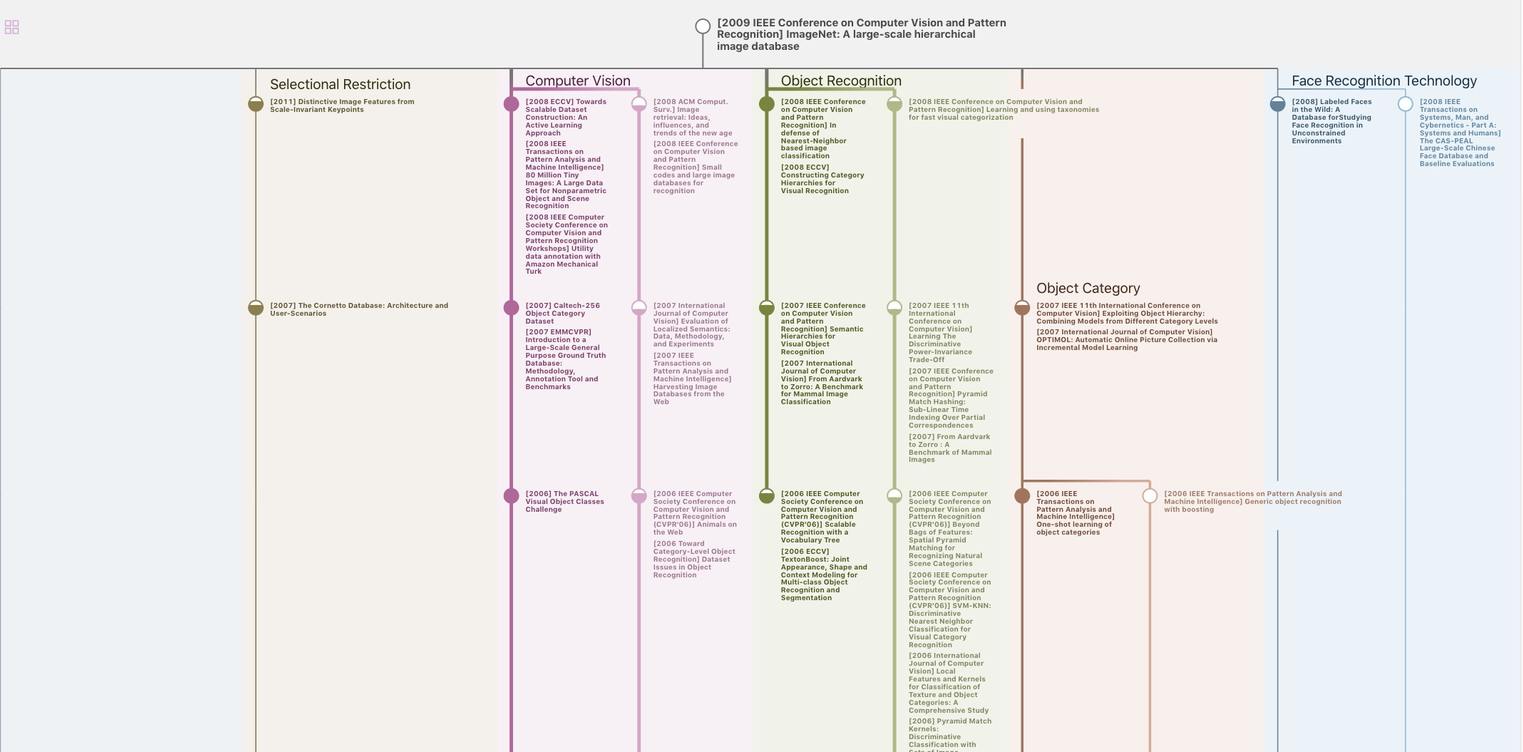
生成溯源树,研究论文发展脉络
Chat Paper
正在生成论文摘要